Using deep learning to enhance the safety of self-driving cars
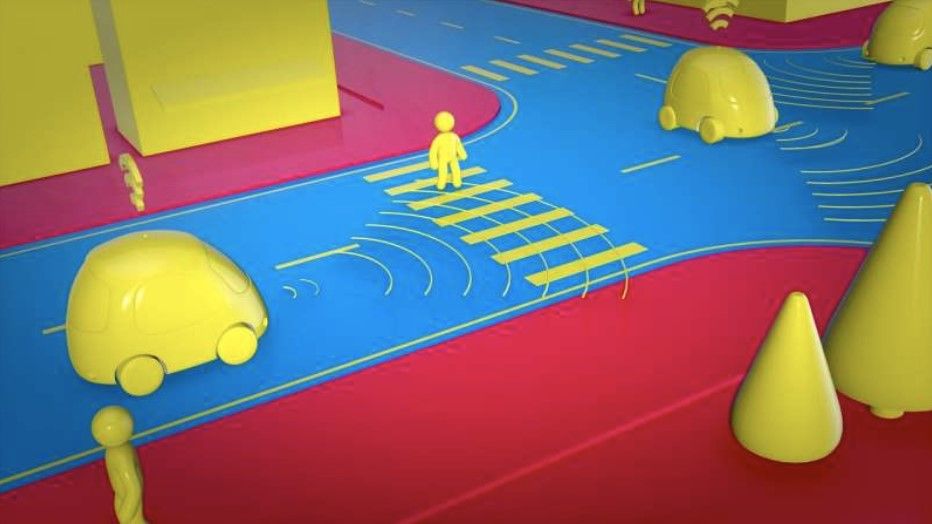
In recent years, autonomous vehicles have been hailed as the next generation of transportation. In order to facilitate autonomous navigation of such vehicles in a variety of scenarios, a variety of technologies must be developed, including signal processing, image processing, AI, deep learning, edge computing, and the Internet of things.
Among the most significant concerns surrounding the popularization of autonomous vehicles is their safety and reliability. Autonomous vehicles must be capable of monitoring and distinguishing their surroundings as well as potential threats to the safety of passengers accurately, effectively, and efficiently in order to offer a safe driving experience for the driver.
In order to achieve this, autonomous vehicles use high-tech sensors (LiDaR), radar, and RGB cameras, which produce large amounts of data in the form of RGB images and 3D measurements, or "point clouds."
This information must be processed and interpreted quickly and accurately in order to identify pedestrians and other vehicles. Advanced computing methods and the Internet of Things (IoT) can enable this through the integration of advanced computing methods into these vehicles, which enables fast, on-site data processing as well as easier navigation around various environments and obstacles.
As a result of a study published in IEEE Transactions of Intelligent Transport Systems, led by Professor Gwanggil Jeon from Incheon National University, researchers developed a smart IoT-enabled end-to-end system for 3D object detection in real-time utilizing deep learning and specialized for autonomous vehicles.
As part of autonomous vehicle operation, environment perception is crucial to answering the fundamental question, 'What's around me?' For autonomous vehicles to perform a responsive action, Prof. Jeon explains that they must have an accurate understanding of their surroundings and environments."
It was developed using the YOLOv3 algorithm, a well-known detection algorithm. The model was initially used to detect 2D objects and then modified to detect 3D objects," he explains.
Data from the RGB images and point clouds were fed into YOLOv3, which produced classification labels and bounding boxes with confidence scores. Their next step was to test its performance on the Lyft dataset. It has been demonstrated that YOLOv3 is capable of detecting both 2D and 3D objects with a high degree of accuracy (>96%), outperforming other state-of-the-art detection techniques.
As well as being applicable to applications requiring the detection, tracking, and visual localization of objects and obstacles, the method can also be applied to autonomous vehicles, autonomous parking, autonomous delivery systems, and future autonomous robots.
At present, autonomous driving is performed by LiDAR-based image processing, but it has been predicted that a general camera will eventually replace LiDAR. Therefore, the technology used in autonomous vehicles is continually evolving, and we are at the forefront of this development," says Professor Jeon. A safe autonomous vehicle should be available in the next five to ten years as a result of the development of element technologies.
Src: Incheon National University
Comments ()