New AI data standards outlined by scientists
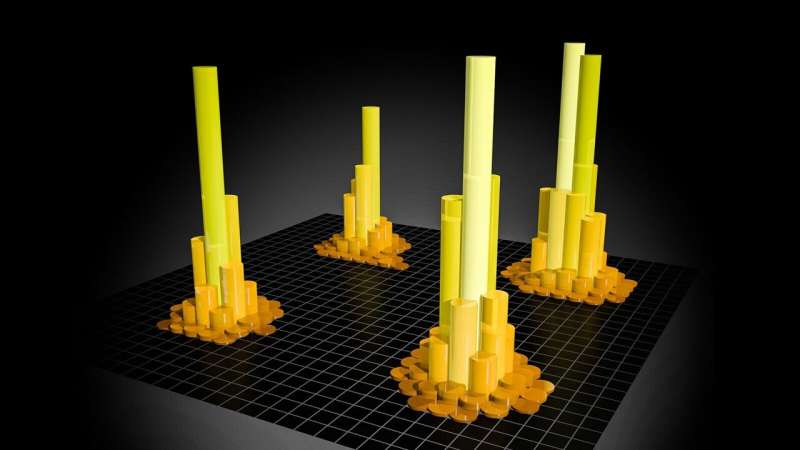
Many budding bakers have to adapt award-winning recipes to fit their kitchen setups. A prize-winning chocolate chip cookie can be made with an eggbeater instead of a stand mixer.
It's important for both talented chefs and computational scientists to be able to reproduce a recipe under different circumstances and with varying setups, since they have to adapt and reproduce their own "recipes". Validating and working with new AI models is also part of the process. Models like these are used in everything from climate analysis to brain research.
Eliu Huerta, a scientist at the Department of Energy's Argonne National Laboratory, said, "When we talk about data, we know what we're dealing with." "With an AI model, it's a little less clear; are we talking about structured data or is it computing, or software?"
Huerta and his co-authors come up with new standards for managing AI models. These standards, called FAIR, stand for findable, accessible, interoperable, and reusable, are adapted from recent research on data management.
It's easier to build AI models by making them FAIR, said Argonne computational scientist Ben Blaiszik. Cross-pollination between teams becomes easier when concepts are reused from different groups."
It's hard to make scientific discoveries because most AI models aren't FAIR, says Huerta. It's hard to access and replicate AI models in the literature for a lot of studies done to date, he said. "When we create and share FAIR AI models, we reduce duplication and share best practices for using these models to enable great science."
Huerta and his colleagues created a FAIR protocol and quantified the "FAIR-ness" of AI models using a unique suite of data management and high performance computing platforms. Researchers paired FAIR data published at a repository called the Materials Data Facility with FAIR AI models from another repository called the Data and Learning Hub for Science, as well as supercomputers and AI resources at Argonne Leadership Computing Facility (ALCF).
Using this framework, researchers were able to build AI models that can run on a variety of hardware and software, generating reproducible results across platforms. It's a DOE Office of Science user facility.
Researchers can access high performance computing resources straight from their laptops thanks to platforms called funcX and Globus. Argonne's Data Science and Learning division director Ian Foster said FuncX and Globus can bridge the gap between hardware architectures. "Our AI language has made it easier to communicate between people using different computing architectures. It's a huge step towards making AI more interoperable."
Researchers used diffraction data from Argonne's Advanced Photon Source, a DOE Office of Science user facility, as an example dataset for their AI model. A SambaNova system on the ALCF AI Testbed and NVIDIA GPUs on the Theta supercomputer were used to perform the computations.
Marc Hamilton, NVIDIA's vice president for Solutions Architecture and Engineering, said they're happy to see FAIR productivity benefits from model and data sharing. "We're working together to accelerate scientific discovery through the integration of experimental data and instrument operation at the edge with AI."
Argonne National Laboratory researchers are excited to partner with SambaNova Systems on innovation at the interface of AI and emerging hardware architectures, said Jennifer Glore, vice president for Customer Engineering. In the future, AI will play a big role in scientific computing. Researchers will be able to enable autonomous discovery at scale with FAIR principles and new tools. ALCF AI Testbed is gonna be a great place to collaborate and develop."
Src: Argonne National Laboratory
Comments ()