Robots learn from words
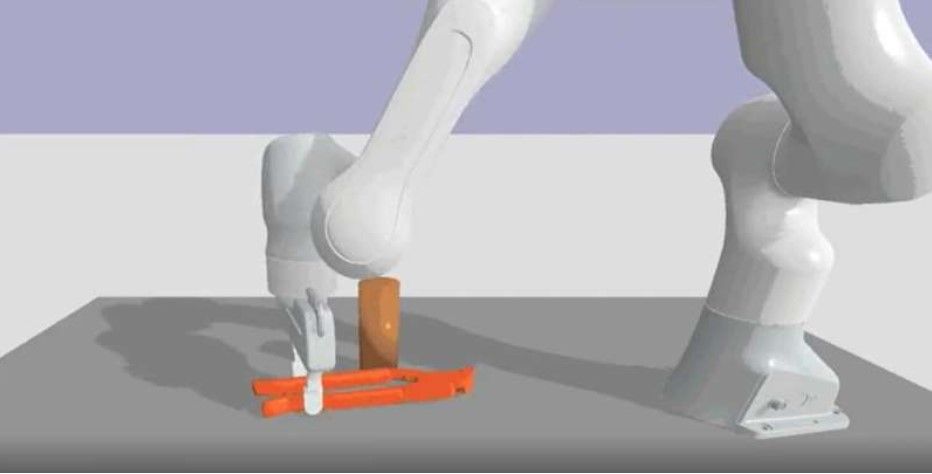
Princeton researchers have discovered that human-language descriptions of tools can assist in accelerating the learning of simulated robotic arms that lift and use a variety of tools.
This research builds on the evidence that by providing richer information during the training process of artificial intelligence (AI), autonomous robots can become more adaptable to new situations, improving their safety and effectiveness.
By including descriptions of a tool's form and function in the training process, the robot was able to manipulate new tools that were not included in its original training set. It was presented by a team of mechanical engineers and computer scientists at the Conference on Robot Learning on Dec. 14.
The development of robotic arms holds great promise for automating repetitive or challenging tasks, however, training robots to manipulate tools effectively is challenging since tools have a wide variety of shapes and robots lack the dexterity and vision of humans.
As you can see, this robotic arm is pushing a tool. The task is one of four assigned to the simulated arm by Princeton researchers. As well as lifting the tool and sweeping a cylinder along a table, they also asked it to hammer a peg into a hole, or try to hammer a peg into a hole. Robots could learn to use unfamiliar tools faster and perform better when they were given human-language descriptions of the tools. Currently, researchers are working to improve the ability of robots to function in novel situations that differ from those in which they have been trained. Aaron Nathans and Allen Z. Ren
Anirudha Majumdar, who leads Princeton's Intelligent Robot Motion Lab, said that additional information in the form of language can assist the robot in learning to use its tools more quickly.
In order to obtain tool descriptions, the team queried GPT-3. It's a large language model that was released by OpenAI in 2020. This model uses a type of AI — deep learning — to generate text in response to a prompt. Having experimented with various prompts, they settled on "Describe the shape or purpose of [tool] in a detailed and scientific response."
In some sense, this is a different way to retrieve information that has been gathered from the internet, as these language models were trained using the internet. This method is more efficient and comprehensive than crowdsourcing or scraping specific websites for tool descriptions. Narasimhan is a faculty member in Princeton's natural language processing (NLP) group and served as a visiting researcher at OpenAI during the development of the original GPT language model.
Narasimhan and Majumdar collaborate for the first time on this project. As a researcher, Majumdar develops AI-based policies to help robots generalize their functions across new environments, and he is keenly interested in the potential for robots to benefit from recent "massive progress in natural language processing."
A set of 27 tools was selected by the team for their simulated robot learning experiments, ranging from an axe to a squeegee. Robotic arms were programmed to perform four different tasks: pushing, lifting, sweeping a cylinder along a table, and hammering a peg into a hole. By combining machine learning training approaches with and without language information, the researchers developed a suite of policies, and then compared them with nine tools with paired descriptions in a separate test set.
Known as meta-learning, this approach involves the robot improving its ability to learn with each successive task it undertakes. It is not only learning how to use each tool, but it is also learning how to understand the descriptions of each of these hundred different tools, so that when it encounters the 101st tool, it is able to learn how to use it quickly, according to Narasimhan. As we teach the robot how to use the tools, we are also teaching it English.
Using the nine test tools, the researchers measured the robot's success in pushing, lifting, sweeping, and hammering and compared the results obtained with policies that utilized language information in the machine learning process and those that did not. Generally speaking, language information enhanced the robot's ability to use new tools significantly.
One task showed differences between the policies was the use of a crowbar to sweep a cylinder, or bottle, along a table.
Ren explained that through language training, the animal gains the ability to grasp the long end of the crowbar and use the curved surface to better constrain the bottle's movement. Since it grasped the crowbar closely to the curved surface, it was difficult to control it without the language."
Majumdar's research team is conducting this study as part of a larger project to develop robots that are capable of operating in new situations that are different from those in which they were trained.
A major objective of the project is to generalize robots—specifically those trained using machine learning—to new environments, according to Majumdar." In addition to failure prediction for vision-based robot control, his group has applied an "adversarial environment generation" approach to robot policy development in environments outside of the initial training environment to improve robot policy performance.
Src: Princeton University
Comments ()