'Raw' data shows AI signals mimic brain activity
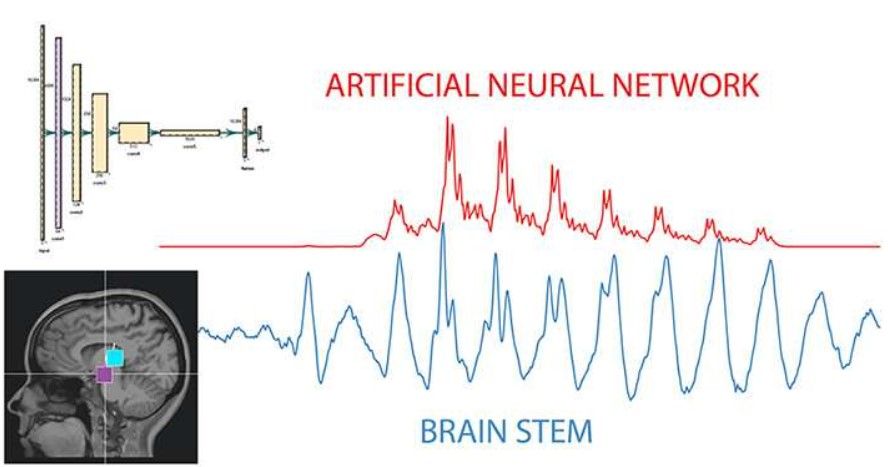
Researchers found strikingly similar signals between the brain and artificial neural networks. The blue line is brain wave when humans listen to a vowel. Red is the artificial neural network’s response to the exact same vowel. The two signals are raw, meaning no transformations were needed.
A recent research from the University of California, Berkeley, reveals that artificial intelligence (AI) systems process signals in a manner strikingly like to how the human brain interprets speech. This discovery could potentially shed light on the "black box" of AI system operations.
The Berkeley Speech and Computation Lab monitored brainwaves of participants listening to the single syllable "bah" using a system of electrodes placed on their heads. They then compared this activity to the impulses generated by an AI system trained to learn English. Lead author Gasper Begus, an assistant professor of linguistics at UC Berkeley, stated that the shapes of these signals were "remarkably similar," indicating similar encoding and processing mechanisms.
Despite the rapid advancement of AI systems like ChatGPT and their potential to transform various sectors of society, scientists' understanding of the inner workings of these tools remains limited. Researchers like Begus, Alan Zhou from Johns Hopkins University, and T. Christina Zhao from the University of Washington are striving to unravel the mystery behind AI system operations.
By examining the brainwaves for speech, which closely follow the actual language sounds, the researchers compared them to the output of an unsupervised neural network interpreting the "bah" sound. Analyzing the raw waveforms from both brain and AI systems could help researchers comprehend and enhance how these systems learn and increasingly resemble human cognition.
Gaining a deeper understanding of AI system operation could provide insights into how errors and biases are incorporated into learning processes, and it could help establish boundaries for increasingly powerful AI models. Begus and his colleagues are collaborating with other researchers to examine how brain imaging techniques might reveal further similarities between human brain activity and AI systems, as well as how different languages are processed in the brain.
Begus believes that speech, with its relatively limited range of sounds compared to visual cues, may hold the key to understanding AI system learning. By closely examining the similarities between AI and human brainwaves, researchers can gauge their progress toward creating mathematical models that accurately resemble human cognition. Begus emphasizes the importance of understanding how different architectures compare to human processing, whether or not the goal is to build systems exactly like humans.
Src: University of California - Berkeley
Comments ()