Discover Public Datasets, Extract and Preserve Data Slices: A Comprehensive Guide
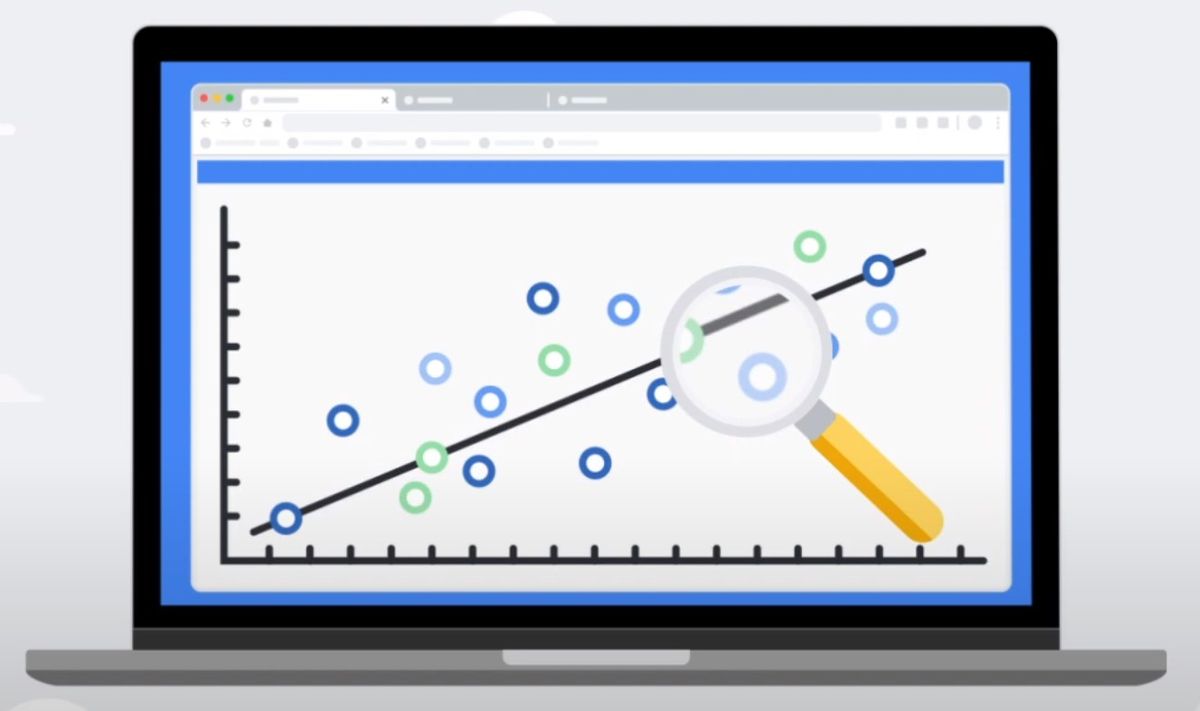
In today's data-driven world, the availability of public datasets has become increasingly important for researchers, analysts, and businesses alike. Public datasets are collections of data that are freely available to the public, often maintained by government agencies, academic institutions, or non-profit organizations. These datasets can provide valuable insights into a wide range of topics, from healthcare and education to economics and demographics.
However, working with public datasets can be a daunting task for those who are unfamiliar with the process. From finding the right dataset to extracting and analyzing the data, there are many steps involved in working with public datasets. That's why we've put together a comprehensive guide to help you navigate the world of public datasets.
In this article, we'll cover everything you need to know about public datasets, including how to find them, extract and prepare data slices, and preserve and manage your data. We'll also discuss the challenges and limitations of working with public datasets, as well as future trends and opportunities for innovation. Whether you're a researcher, analyst, or business owner, this guide will provide you with the tools and knowledge you need to work with public datasets effectively and efficiently.
Understanding Public Datasets: What They Are And Why They Matter
Public datasets are an essential resource for researchers, allowing for the verification of publications, interdisciplinary use, and the advancement of science. These datasets are often made available through government open data portals such as Data.gov and the EU Open Data Portal. Researchers can also access public data archives such as Harvard Dataverse and VertNet to retrieve information relevant to their research.
However, some researchers may refrain from reusing public datasets due to concerns about quality or lack of awareness. To ensure that these datasets are used effectively, metrics should be in place to evaluate the impact and utility of a given repository. Furthermore, data preservation is also crucial in maintaining the accuracy and accessibility of public datasets. This involves converting data into a preservation format before storing it in a specialized repository.
Datasets provide scope, robustness, and confidence to results obtained through computational fields like machine learning or bioinformatics research. It is therefore imperative that researchers have sufficient knowledge of bioinformatics tools when working with these large-scale databases for optimal efficiency.
In conclusion, public datasets provide invaluable resources for researchers across different fields who want reliable information at their fingertips whenever necessary. They significantly ease access to valuable information while optimizing storage space by avoiding unnecessary duplication from multiple sources offering similar types of dataset content thereby giving room for more effective scientific exploration activities while guaranteeing optimal data usage optimization choices where appropriate ones need more than just generic trends!
How To Find Public Datasets: Tips And Tools For Effective Search
One of the most challenging aspects of data analysis is finding the right dataset. Fortunately, there are a variety of tools and resources available to help you find the perfect dataset for your analysis. Cloud hosting providers like Amazon and Google offer large public datasets for free, which can be accessed easily by researchers all over the world.
In addition to these cloud-hosted datasets, public repositories like Data.gov and Google Dataset Search can help researchers find relevant data quickly and easily. Web scraping can also be a highly effective method for extracting specific information from unstructured data sources. Tools like Scrapingbee and ParseHub offer options for web scraping with modest investments.
Researchers should think strategically when searching for public datasets - it's important to select appropriate resources that match research requirements effectively. Apart from traditional search engine operating technique, subreddits on Reddit provide information on potential datasets e.g., r/Datasets & r/DataViz will take you through experiences from other users who have dealt with certain types of analyses or specific projects.
Proper cleaning and wrangling techniques must be applied when using raw datasets; Geospatial data dealing with geographies may also require special handling techniques unique to spatial data subset. Visualization tools like GapMinder should also be considered in analyzing acquired sets: they assist in understanding trends faster than manual review scans would allow.The size may vary so using AWS Public Data sets that offer access to massive collections is the direction one could head towards depending on project size and processing capacity limitations.
Extracting Data Slices: Techniques And Best Practices
Extracting data slices is an essential process in data analysis, involving a number of techniques and best practices. Excel is a basic tool for data extraction, with its PIECES approach serving as a more advanced method. Incremental and full extraction methods exist, with the former being advantageous in situations where only new or modified data needs to be extracted.
To organize extracted data more effectively, evidence and summary tables are used. Data mining can also improve the efficiency of traditional systematic reviews, allowing relevant information to be obtained quickly. ETL (extract, transform, load) further consolidates data from different sources into one centralized location.
To reduce human error during the cleaning aggregation step after extraction, automation techniques are applied. Additionally, developing a framework for secure big data collection is important for storing sensitive information while maintaining privacy safeguards such as pseudonymization and minimizing unnecessary data.
Effective extraction and preservation of public datasets involves applying proper techniques such as incremental extractions and developing frameworks to ensure secure big dataset collection without infringing on privacy rights. Employing best practices like PIECES approach or automating the cleaning aggregation steps can improve accuracy and efficiency while extracting necessary information using tools available like Excel as well as organizing them through tables and summarization methods can help package the information for use later on in research or analysis tasks. Finally making sure that compliance policies are followed when masking sensitive personal identifiable datapoints is imperative for preserving public trust in large scale datasets which have potentially personal information included within them
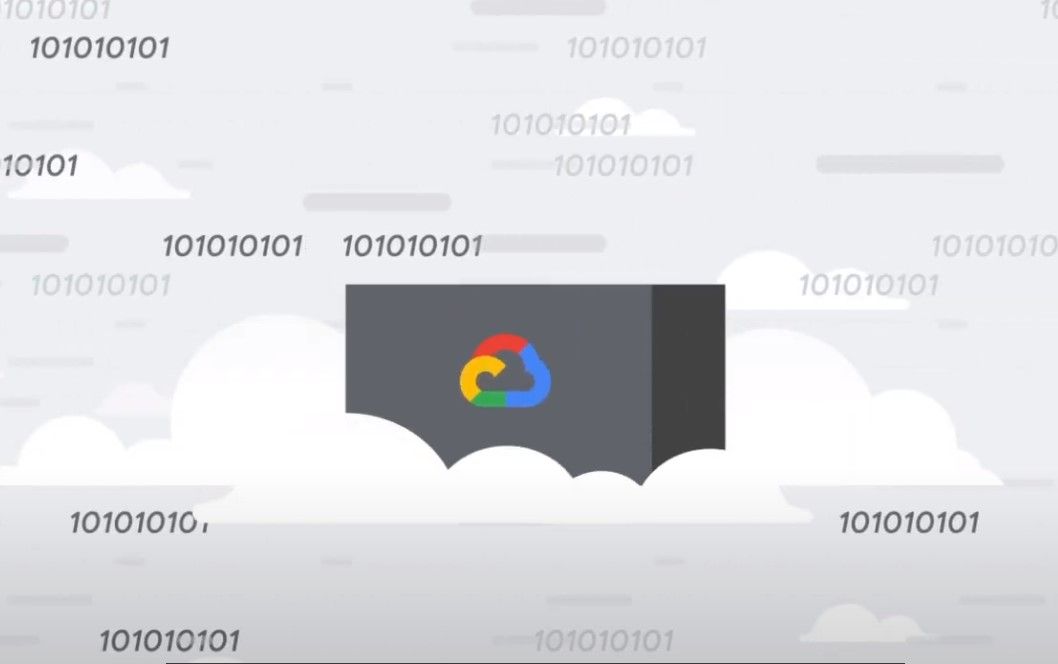
Data Cleaning And Preparation: Ensuring Quality And Consistency
Data cleaning is an essential first step in any machine learning project and involves editing, correcting and structuring data within a dataset so that it's generally uniform and prepared for analysis. The process of data cleansing helps to spot and resolve potential data inconsistencies or errors proactively, thereby improving the quality of your dataset.
An important concept in data cleaning is the principle of data quality. There are several characteristics that affect the quality of data including accuracy, completeness, consistency, timeliness, validity, and uniqueness. Data cleansing involves removing unwanted, duplicate or incorrect information from datasets to help analysts develop accurate insights into different trends. The analyst can choose to initiate this process at various stages of their work as it involves a repetition cycle of diagnosing suspected abnormalities.
There are three stages involved in the data cleaning process: Cleaning (the actual removal of unnecessary pieces), Verifying (ensuring all corrected action has been done correctly) and Reporting (placing the cleansed dataset back into storage). Having a proper approach towards these three stages will save more resources like time working on needed improvements while disregarding areas that have already resolved issues or well functioning as intended by limiting unnecessary interference with well performing datasets
To maintain high-quality datasets~ experts advocate addressing any inherent biases within datasets throughout acquisition through identifying trends across sources. Removing wrongly formatted imports caused by unicode translation occurrences which distort gathered records during ingestion events into raw form before transformation so they're preserved accurately later is also common practice during preparation stage before cleaning involves regular checking steps such as statistical measures applied on subsets published previously duplicates/null values among features relations with other provided features difference existence between training/testing subsets stored externally.
Ensuring you work with clean consistent public datasets is crucial because this guarantees accurate analyses while saving resources to reshape dirty noisy one's later in your pipeline. Though getting spotless aggregations can be tough It will ultimately favor understanding intricacies within each column feature since compiled source typically holds vital facts not easily accessible that can link to each other and discovered for new insights which wouldn't be possible otherwise.
Preserving Data Slices: Storage, Management, And Sharing
Data preservation, management, and sharing are essential practices for scientific research. The Stanford Digital Repository provides digital preservation services that ensure the long-term citation, access, and reuse of research data. To preserve security and privacy while sharing encrypted data, privacy-preserving technologies such as fully homomorphic encryption and differential privacy can be used.
Best practices in data management include depositing datasets in trusted subject-specific, governmental, or institutional repositories while providing metadata to make them findable. Regular backups should also be scheduled to prevent data loss. Distinguishing between working storage and preservation is important because it allows researchers to maintain secure working copies of their data while preserving the original dataset.
Despite PLOS's establishment of stringent policies on data availability in 2014, few researchers adopt best practices for data sharing. Sound data management practices are necessary for Open Science goals to be accomplished successfully. With the proper maintenance of public datasets through efficient preservation techniques and with access provided via trusted repositories accompanied by good metadata support will help generate a vast amount of high-quality research results that anyone could use freely without any restrictions.
A solid plan may guarantee optimal storage space allocation which ensures easy accessibility towards useful scientific outcomes derived from concatenated clean subsets saved into trusted archives at reliable institutions accessible globally by big collaboration teams similar to those associated currently with Climate Sciences looking forward towards new collaborative technologies enabling innovative proposals fostering further interoperability among different archives on a multidisciplinary level worldwide.
Analyzing Public Datasets: Methods And Approaches For Insightful Results
Public datasets are a valuable resource for data analysts and researchers looking to gain insights into various topics. These datasets can be accessed from government agencies and other organizations that collect and share data on everything from population demographics to healthcare outcomes.
To best analyze public datasets, it's essential to first clean up the data by removing inaccuracies and duplicates. This will ensure that the resulting analyses are accurate. Transforming the dataset into a more useful format is also important, as it can make it easier to work with and extract meaningful insights.
Once the dataset is cleaned up and transformed, analysts can explore its contents using various analytical methods such as descriptive statistics, regression analysis, or clustering techniques. These methods enable researchers to identify patterns in the data that may yield interesting insights.
One increasingly popular approach used for exploring public datasets is machine learning. Machine learning algorithms allow analysts to discover complex patterns in large datasets that may not be visible using traditional approaches. For example, machine learning has been used successfully in areas like healthcare and finance where predicting future trends is critical.
Ultimately, analyzing public datasets requires careful planning and attention to detail during all phases of the process from exploratory analysis through refinement of models until final conclusions are drawn. Researchers should pay attention to how they transform their data slices so they do not alter them significantly while preserving most features necessary for insightful results.
Challenges And Limitations Of Public Datasets: Strategies For Overcoming Obstacles
One of the main reasons why researchers are hesitant to reuse publicly available datasets is due to concerns about quality and reliability. However, using secondary data can eliminate many challenges and confer benefits. With the increasing adoption and implementation of digital technology, there is a wealth of big data that can be utilized in research projects.
Managing large volumes of data can be a challenge in big data, as it requires advanced bioinformatics knowledge and skills. There are eight major data integration challenges that must be tackled, including metadata management, standardization, validation, security/privacy protection, storage/retrieval optimization, interoperability between platforms/systems/users/applications and long-term preservation.
Open government data presents an opportunity for researchers to access vast amounts of information that would otherwise not be available. However, it also requires careful consideration around individual-level data privacy protection. As such, feedback and peer review mechanisms should be put in place to improve data analysis skills and overcome challenges associated with analyzing public datasets.
Centralizing the management of public datasets is one way to help protect it from breaches or unauthorized access while reducing the risk of errors or inconsistencies arising from different versions or formats. By centralizing regulation efforts towards access control mechanisms like authentication protocols that include credentials-based systems or single sign-on integration while backing up all critical files regularly on secure servers or cloud providers providing encryption services make them more trustworthy sources for research purposes with better security features than decentralization alternatives without any clear responsibility assigned hence higher exposure through more attack surfaces potentially leading to worse damages if attacked by danger actors like hackers thus making sure you extract valid slices of information from them safely and effectively.
Future Directions In Public Datasets: Trends And Opportunities For Innovation
The increasing demand for secure and privacy-preserving sharing technologies is driving the trend of data-sharing. More than 70% of global data and analytics decision-makers are expanding their ability to use external data. In order to share data more openly, it is critical to have reliable methods for extracting and preserving data slices.
Data science has become a crucial component in successful scientific research, including public health research. Big data is inducing changes in how organizations process, store, and analyze information. Context-driven analytics and AI models are expected to supersede 60% of traditional data models by 2025.
Machine learning (ML) techniques such as natural language processing (NLP) are being used to extract insights from diverse datasets automatically. Furthermore, NLP can generate intelligent summaries directly from extens ive collections of texts that would take humans significant time to analyze manually.
The growing volume of public datasets provides opportunities for researchers across different domain applications to analyze large-scale datasets that was previously impossible. Analyzing these massive amounts of digital archives will provide new insights into human behavior, culture growth and other societal aspects that were previously undiscovered or inaccessible through intuition alone.
Comments ()