AI models cannot match the visual processing ability of humans, no matter how smart they are
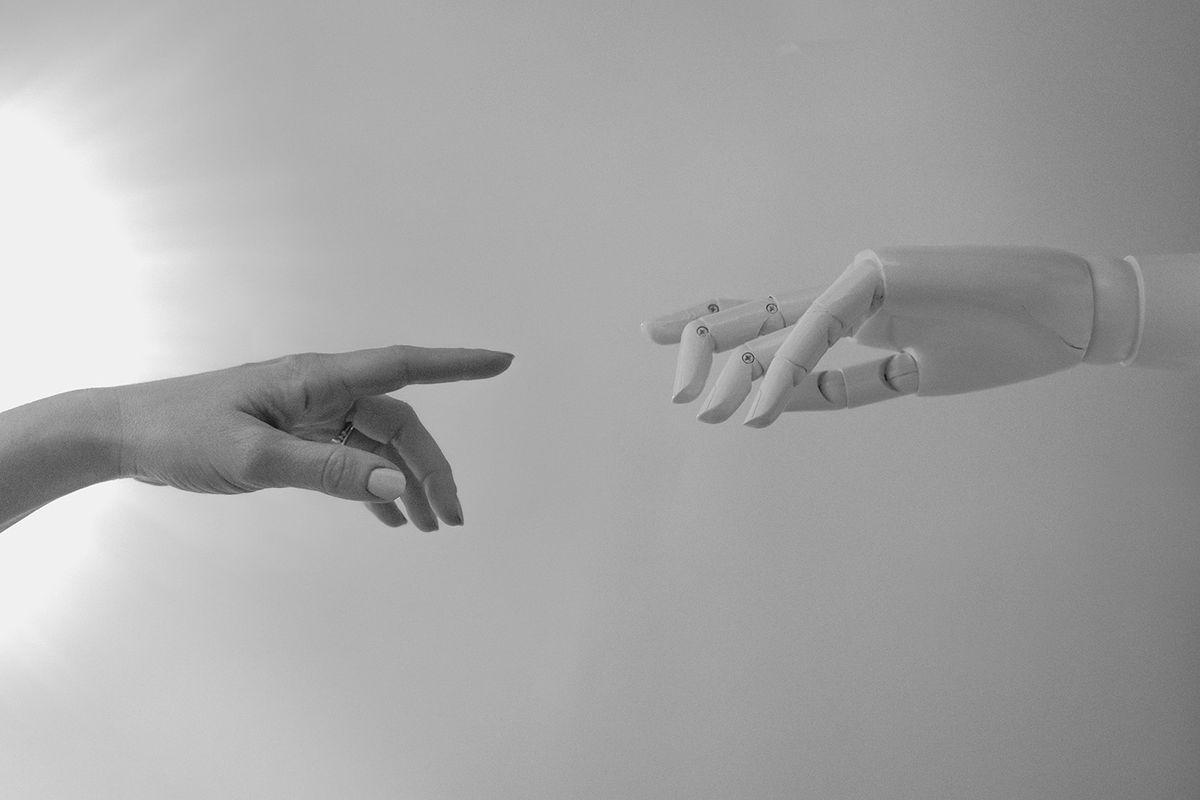
Human object perception is characterized by its sensitivity to the holistic configuration of the local shape features of an object. At present, deep convolutional neural networks (DCNNs) are the dominant models for the recognition of objects in the visual cortex.
However, do they capture this configural sensitivity? For answering this question, York University researchers used a dataset of animal silhouettes and created a variant that disrupts the configuration of each object while preserving local features.
Despite the fact that human performance was adversely affected by this manipulation, DCNN performance was not affected, indicating that object configuration is not significant. Attempts to modify training and architecture to make networks more brain-like failed to produce configural processing, and none of the networks predicted human object judgements accurately.
To match human configural sensitivity, networks may have to be trained to perform a wide range of tasks beyond the recognition of categories of objects.
In real-world AI applications, deep convolutional neural networks (DCNNs) may not be able to perceive objects in the same way humans do -- using configural shape perception. This could be dangerous, according to Professor James Elder, co-author of the study.
The study published in Cell Press Journal iScience, Deep learning models fail to capture the configural nature of human shape perception, was conducted by Elder, a York Research Chair in Human and Computer Vision and Co-Director of York's Centre for Artificial Intelligence & Society, and Nicholas Baker, a former York postdoctoral fellow in psychology, at Loyola College in Chicago.
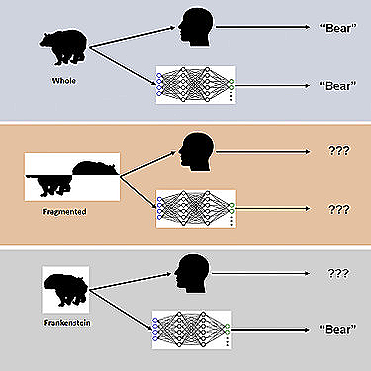
Using novel visual stimuli known as "Frankensteins", the study examined how the human brain and DCNNs process holistic, configurable object properties.
Elder explains that A Frankensteins are simply objects that have been disassembled and reassembled in the wrong direction. The result is that they have all the right local features, but they are located in the wrong locations.
According to the study, while the human visual system is confused by Frankensteins, DCNNs are not, indicating that they are insensitive to the properties of configurable objects.
As a result of this study, deep AI models fail under certain conditions, indicating the need to take into account tasks beyond object recognition to gain a deeper understanding of how the brain processes visual information. As a result of these deep models, it is common for them to take 'shortcuts' when attempting to solve complex recognition tasks. Despite their effectiveness in many cases, these shortcuts can pose a danger in some real-world AI applications.
Traffic video safety systems are one such application: Traffic objects, such as vehicles, bicycles, and pedestrians, obstruct each other and appear to a driver as a jumble of disconnected fragments. It is necessary for the brain to properly group these fragments in order to identify the correct categories and locations of the objects. Using an AI system for monitoring traffic safety that is unable to perceive fragments individually will fail at this task, potentially misinterpreting risk to vulnerable road users.
In the study, the researchers found that modifications to training and architecture aimed at making networks more brain-like did not result in configural processing, and none of the networks could accurately predict human object judgements on a trial-by-trial basis.
It is necessary for networks to be trained to solve a broader range of object tasks in order to match the configuration sensitivity of humans.
Comments ()