A creative approach to drug discovery
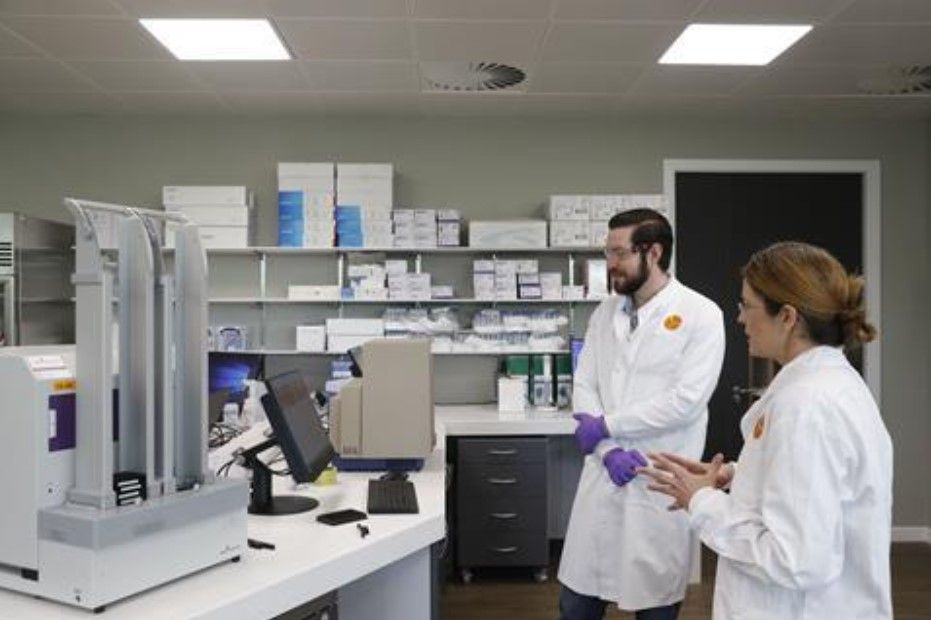
Human intuition and experience can be complemented and reinforced by machine learning.
During the last decade, artificial intelligence (AI) has become increasingly prevalent in drug discovery. A number of start-ups have worked to convince traditional firms that artificial intelligence and machine learning can save them time and money while increasing the novelty of their products.
In the period 2000-2015, 86% of drug development programs failed. It is estimated that the costs associated with bringing a new drug to market could range from hundreds of millions to several billion dollars. The pharmaceutical industry's economics need to be changed. It is imperative that we change the way drugs are invented,' asserts Andrew Hopkins, the founder of Exscientia.
Hopkins recalls that when Exscientia began around 10 years ago, there was strong skepticism about how algorithms and machine learning might be able to accomplish such a creative task as drug discovery. There has been a change in attitudes, however. A growing number of partnerships are being forged between established pharmaceutical companies and younger start-ups in the field of artificial intelligence.
It was announced in May 2022 that Sanofi would partner with Exscientia to develop 15 novel small molecule candidates in oncology and immunology with a cash contribution of $100 million (£80 million). There is a possibility of Sanofi paying as much as $5.2 billion if the project reaches its development milestones.
Recursion, an AI biotechnology company based in Utah, signed an agreement with Roche and Genentech to uncover molecules using AI for neurological and cancer indications. The deal is valued at $150 million up front, with performance milestone payments of up to $300 million. Moreover, AstraZeneca expanded its collaboration with BenevolentAI to cover lupus and heart failure last year.
Human intuition and experience must be combined with data in order to achieve success
A joint venture between Bayer and Google Cloud was announced in January 2023 to accelerate Bayer's quantum chemistry calculations in order to drive early drug discovery using machine learning. This will enhance in silico modeling of biological and chemical systems, and will therefore aid in the identification of potential drug candidates.
However, in late January, Alphabet, the parent company of Google, announced it would cut 12,000 jobs worldwide, including many in its drug discovery teams. InstaDeep was acquired by BioNTech for £362 million to aid in the development of immunotherapies and vaccines during the same period.
Miraz Rahman, a medicinal chemist at King's College London, UK, states, "The ability to map novel disease pathways and identify new targets has tremendous potential." As a result of the deluge of data, which can be mined by AI, as well as increasingly automated wet lab experiments, large pharmaceutical companies are partnering with AI-based companies.
Taking a step back from messy biology
The study of biology is complex, while the discovery of new drugs is based on information. Researchers established Recursion to develop better maps of human disease biology in order to discover better medications. According to Joseph Carpenter, Recursion's head of medicinal chemistry, we have a serious lack of understanding of fundamental human biology.
In addition, over the past few years there have been a large number of new scientific publications, as well as cell, tissue, and patient data pertaining to disease targeting. A machine learning algorithm is capable of detecting patterns in large quantities of data that are impossible for humans to recognize.
With its headquarters in Hamburg, Germany, Evotec views artificial intelligence as a means of bringing biologists and chemists together and digesting the flood of scientific articles and insights through natural language processing. The secret to bringing together human intuition and experience with data is to combine it with human intuition and experience, as explained by David Pardoe, head of global molecular architects at Evotec.
Although artificial intelligence and machine learning need to convert biology into quantifiable values, humans remain an integral part of the equation. The goal is to encode drug discovery, according to Hopkins. But we must examine how human expertise, tacit knowledge, and creativity become codable, repeatable, and scalable.'
This is the reason why the company calls its AI platform Centaur, after the human-horse hybrid from Greek mythology. In collaboration with the Japanese firm Sumitomo Dainippon Pharma, Centaur molecules are currently in clinical trials to treat cancer, obsessive-compulsive disorder and Alzheimer's disease psychosis.
Technological advancements have contributed to the growth of the field. First, there has been a significant increase in computing power, as well as the large amount of available data,' notes Rahman. In AI biotech, some companies use the fastest supercomputers in the world to train their predictive models, which are more accurate when there are large training sets.
It used to take Rahman six months to run a molecular dynamics simulation of a molecule interacting with a protein target, but now this can be done in a matter of days or weeks with a higher level of resolution. The result is that the data that is obtained on the interaction between compounds and the biological system is much more realistic, according to Rahman. An improved understanding of molecular docking, molecular dynamics, and pharmacokinetic properties of molecules is crucial in the preclinical phase to prevent costly failure in the clinical phase.
The possibilities are endless
By utilizing artificial intelligence, Insilico identified a new target for idiopathic pulmonary fibrosis, a chronic lung disease, and then developed a small molecule drug candidate to combat it. The only way to accomplish this was to tap into an enormous amount of information. Insilico has 10 million data samples, 40 million publications, and close to two million compounds as well as biological data, which includes structure, activity, safety and druggability. The amount of data that has been collected by Recursion has reached 21 petabytes, according to Carpenter.
The goal of machine learning and artificial intelligence is to produce fewer but better molecules using colossal datasets. Contrary to the traditional combinatorial approach adopted in the 1990s, which generated millions of compounds, only a small proportion of which reached the market. According to Pardoe, information-rich molecules are becoming a growing focus. The goal is no longer to manufacture thousands of molecules,' he explains. In other words, we are looking for molecules that can help us understand what a [drug] binding site looks like, or how to interact with a binding site.
It is no longer necessary to make thousands of molecules. In order to understand the problems, we need molecules that provide information
Earlier this year, Exscientia reported the discovery of a novel CDK7 inhibitor, which has the potential to treat cancer. In less than a year, 136 novel compounds were created and tested. According to Hopkins, the company's immuno-oncology candidate was developed as a result of a rapid discovery process, in which around 176 novel compounds were created and tested to discover the compound in question.
On average, he estimates, they make about 250 novel molecules going from an idea to a drug candidate, compared to about 2500 to 5000 molecules in the industry on average. A physics-based platform enabled Schrödinger to assess 8.2 billion compounds computationally and synthesize just 78 molecules over a period of 10 months of 'design, make, test,' before choosing an anticancer molecule that was cleared for a clinical trial in lymphoma patients.
A major benefit of AI is faster discovery. An Insilico paper published in 2019 described a deep generative model for small molecules that maximizes synthetic feasibility, novelty, and biological activity for the treatment of fibrosis and other diseases. In 21 days, we conducted the experiments and developed a molecule that was able to reach mice within 46 days, claims Alex Zhavoronkov, the founder of the company. With Evotec's breakthrough technology, Pardoe says the company will be able to develop a preclinical candidate within 21 months or less from the time it is exploring a target.
In spite of the enormous amounts of data available, Rahman cautions that predictive models are always subject to error. During the validation process, he concluded, you need experimental data. Insilico is one of several AI companies investing in highly automated wet labs. In the next five to ten years, experimental datasets will likely increase, enhancing these AI models." That will likely significantly increase the success rate.
Carpenter of Recursion concurs: 'Having a large dataset is critically important, but the data must also be reliable.' The company's highly automated laboratory in Salt Lake City, US, performs more than two million wet lab experiments a week. Predictions and calculations, synthesis, and lab experiments are then brought together to develop new drugs.
All areas are accessible
The AI community is quick to assert that their algorithms are agnostic to therapeutic areas. However, efforts are still skewed in favor of cancer, particularly. According to Rahman, that is where there is a greater return on investment. The author advocates the use of artificial intelligence (AI) to identify antimicrobials, particularly for Gram-negative bacteria such as Pseudomonas aeruginosa, where any increase in success rates would be welcome. Exscientia is collaborating with the Bill & Melinda Gates Foundation to develop therapeutics against viruses with pandemic potential in the laboratory of Jim Collins at the Massachusetts Institute of Technology, US.
Drug development for neurodegenerative diseases is also of interest, which has proven to be difficult in the past. The traditional approaches to these diseases have not been very successful, and there is a large unmet clinical need here,' says Rahman. It is possible that artificial intelligence could uncover druggable targets for Alzheimer's disease, a disease for which there are few known targets.
A phase 2 trial for BenevolentAI's AI-inspired candidate for Parkinson's disease has been successfully conducted in the UK. In phase two of the company's pipeline is a candidate for the treatment of atopic dermatitis. A five-year collaboration between Insitro and Bristol Myers Squibb was initiated in 2020 to address two neurodegenerative disorders - amyotrophic lateral sclerosis and frontotemporal dementia.
As well as central nervous system disorders, such as Parkinson's disease, Insilico is also interested in fibrosis, oncology, and immunology. Ren explains that all of these are related to aging: "We wish to utilize our algorithm to gain a greater understanding of the biology of aging and age-related diseases.".
Complexity on a scale
The application of AI and machine learning to complicated biological drugs is beginning to go beyond small molecule drugs. The Exscientia Corporation began developing human antibodies in late 2022. Hopkins states, "We are exploring deep learning approaches to generate and screen, virtually, incredibly large libraries of virtual antibodies as a proof of concept." This will ultimately lead to the development of antibodies that are directed against specific targets.
It is crucial to model how proteins will fold in order to determine the behavior of protein-based therapeutics, or drug targets that are not easily accessible in the lab. It has been found that AlphaFold, the algorithm developed by Google subsidiary DeepMind, can make much better predictions of 3D protein structures based on amino acid sequences than previous algorithms. Pardoe believes that AlphaFold will have a significant impact on drug discovery, despite the fact that it is unlikely to revolutionize it immediately.
Several companies, including Generate Biomedicines in the United States, are developing their own algorithms. Using a combination of computational and laboratory methods, Generate has examined amino acid sequences, structure, and function of known proteins in order to identify statistical patterns.
Generate's head of machine learning, John Ingraham, believes that learning general principles of protein folding from existing structures could enable the company to design entirely new protein architectures, or to develop binders that perturb human proteins in just the right way to address disease states. Five clinical targets are being pursued in collaboration with Amgen.
In addition, AI is increasingly being used not just in early drug discovery, but also in the development
Src: chemistryworld
Comments ()