What Makes the Best Machine Learning Data Annotation Tool?
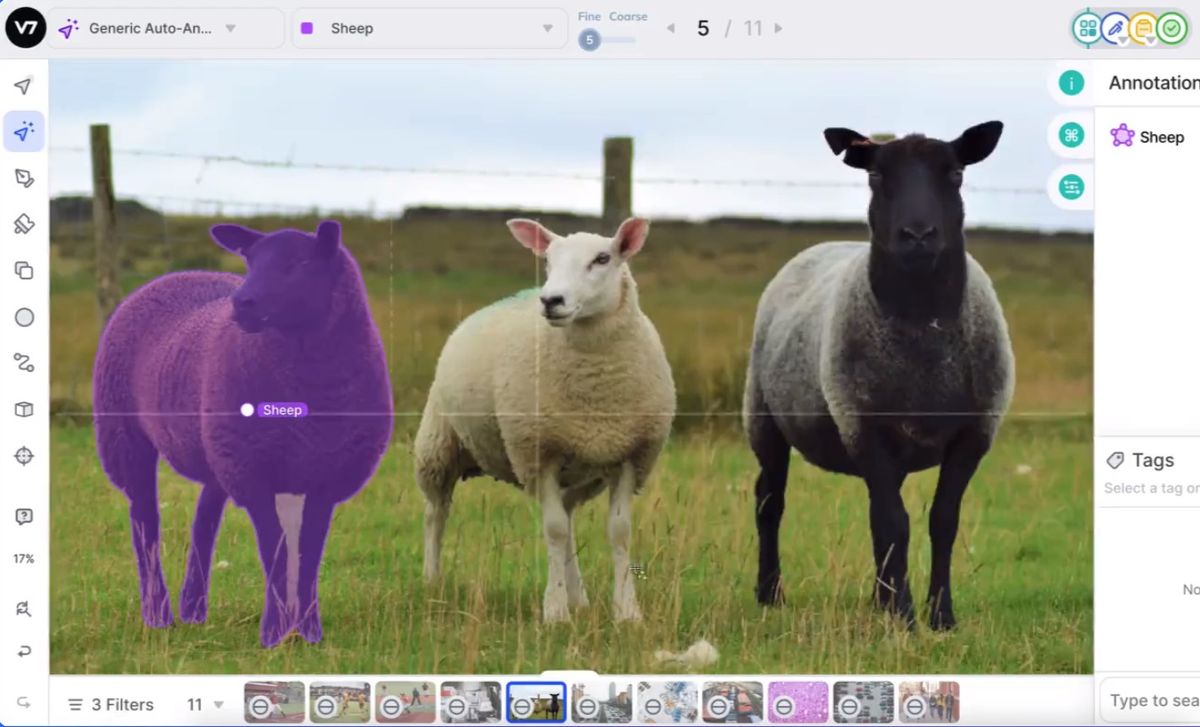
Data annotation tools are essential for training and developing machine learning models. These tools allow for the annotation and labeling of data. This step is crucial for models to perform image and speech recognition tasks.
This blog explores the features that make the best machine-learning annotation tool. It examines user-friendliness, scalability, accuracy, collaboration, and security. By the end, you'll gain a deeper understanding of machine-learning annotation tools.
What to Look for in a Data Annotation Tool
The need for accurate and efficient annotation tools has never been greater. But how do you decide which tool to choose when so many options exist? Explore what sets a great annotation tool apart from the rest.
User-Friendliness
An annotation tool can improve the efficiency of the data annotation process. It should have an intuitive interface and customizable options for advanced users.
A good data annotation tool should be simple to navigate. Also, the tool interface should be easy for users to understand and use. Features should include clear labels, well-organized menus, and simple instructions.
The tool should have customizable options for advanced users. Examples could include custom annotation formats, advanced data visualization, and more options.
Furthermore, the tool should have comprehensive training and support resources to assist users. Training and support systems can assist users in maximizing the tool's potential.
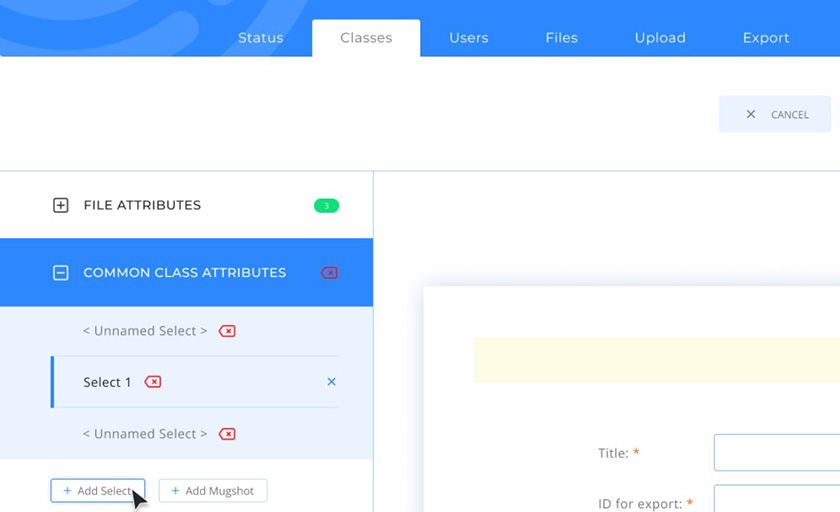
Scalability
An effective data annotation tool should be capable of handling large datasets. It should function regardless of the scale or quantity of annotations. Images, text, audio, and video are examples.
The tool should also integrate with other machine-learning tools. For instance, tools for data pre-processing and model training may be necessary. It should also work in cloud-based environments to accommodate different uses. This feature makes the tool more versatile, effective, and accessible.
Accuracy
A data annotation tool should produce high-quality results across different annotators. Consistency guarantees the labels' accuracy and enables the training of machine learning models. It should also include other languages.
Quality assurance guarantees the tool has built-in quality control mechanisms. Examples include automatic annotation quality reviews, guidelines, and quality assurance workflows. These ensure consistency and accuracy in your annotations.
Furthermore, the tool should provide detailed annotation statistics and reports. Then, users can test and improve the performance of their annotations in real time. This capability aids in enhancing the machine-learning model’s quality.
Collaboration
The tool should support team annotation. It will allow different users to edit the same dataset. As a result, you can increase productivity and complete processes faster.
The annotation tool should also possess version control functionality. This feature ensures that data stays accurate and up to date. In addition, it facilitates data modification tracking and allows for easy rollbacks if there are errors.
Annotation review and feedback features are also important. It enables users to examine and give feedback on annotations other users make. As a result, the data is correct and catches errors early in the process.
Additionally, the tool should have a centralized annotation management system. This feature allows you to track the annotation progress and assign tasks to different annotators. You can keep the annotation process organized and efficient.
Collaboration features help improve efficiency, accuracy, and quality. When working in a team, tracking and managing the annotation progress is simple.
Security
Data privacy and protection are crucial annotation tool features. They're essential when working with sensitive or confidential information. Your tool should have robust security features that protect users' privacy.
Access controls for sensitive data allow users to set access levels for other users. For example, you can restrict access to specific data or annotation tasks. Compliance with industry regulations is also essential. The tool should follow HIPAA, GDPR, and CCPA.
Data encryption and secure data storage options are also essential features. They ensure data is safe from unauthorized access, even in a security breach. The tool should have a robust backup and disaster recovery system. This feature allows you to restore data in case of its loss.
Conclusion
Your data annotation tool is crucial for the success of machine learning projects. User-friendliness, scalability, accuracy, collaboration, and security are all critical factors. Choose a data annotation tool for your specific needs and machine-learning goals.
Comments ()