What Are The Main Challenges in Data Annotation for In Waste Management projects
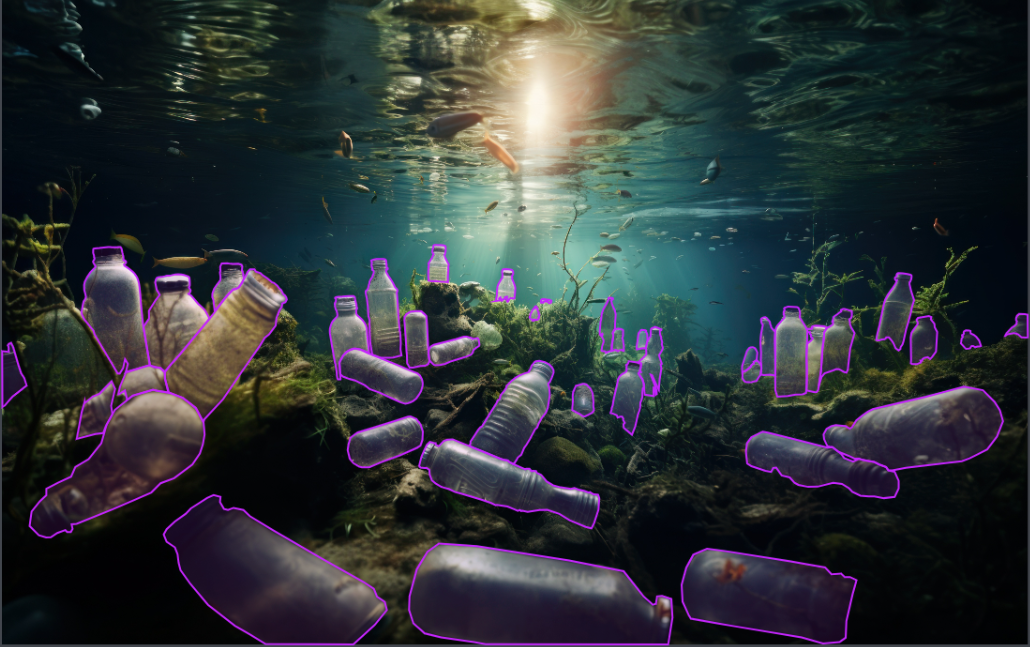
Waste management is a critical issue that affects our environment, economy, and health. Despite the growing awareness of the importance of waste management, there are still many challenges that need to be addressed, particularly in the area of data annotation. Data annotation is the process of identifying and categorizing waste materials to create accurate and reliable data sets that can be used for analysis and decision-making.
However, there are several challenges to data annotation in waste management. One of the main challenges is the lack of standardized data annotation methods, which makes it difficult to compare and analyze data from different sources. Another challenge is the difficulty in identifying and categorizing waste materials, which can lead to inaccurate data. Additionally, collecting accurate data from waste management facilities can be challenging, and there is a limited availability of skilled personnel for data annotation.
Despite these challenges, data annotation is crucial for effective waste management strategies. In this article, we will explore the main data annotation challenges in waste management and discuss the future prospects for improving data annotation in this field.
Introduction To Data Annotation In Waste Management
If you're pursuing better waste management, data annotation is a critical part of the process. Accurate labeling of waste materials helps to streamline and manage the collection, treatment, and disposal process. However, businesses face challenges in managing and streamlining data annotation effectively.
In manual image annotation, managing a large workforce is a challenge that can diminish data consistency and quality in labels. Ensuring consistency in labeling is essential to creating useful datasets for developing AI systems for effective waste management. Inadequate dataset quality due to ineffective data annotation can lead to lower performance when training machine learning models.
Waste management has become a global concern due to increasing environmental degradation caused by pollution from untreated waste. Poor waste management can result in health hazards or environmental contamination issues like water or soil pollution that may take years to remediate fully.
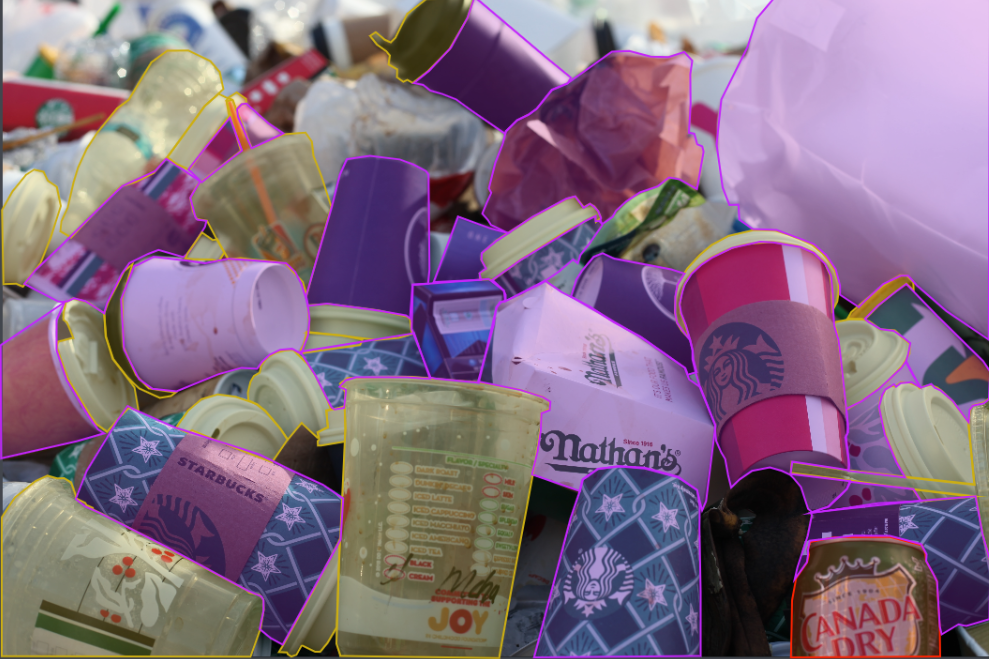
As such, it's critical to consider the quality and consistency of labels while effectively leveraging technology solutions such as AI systems for more accurate automated identification of recyclable items like plastics or metals. Furthermore, balancing objectives between recycling and protecting consumers' safety or minimizing resource use during the disposal process is essential for optimal sustainable practices.
Lack Of Standardized Data Annotation Methods
Waste management data plays a crucial role in creating policies and planning for the local context. However, one of the biggest challenges in waste management is the lack of standardized data annotation methods. Often, subset data annotations are inconsistent and not unified to one standard style, making it difficult for policymakers to make informed decisions.
To solve this problem, all subset data annotations should be unified to one standard style. Moreover, segmentation and instance segmentation masks must be converted to standardized COCO bounding box annotations. This will ensure that waste management data is consistent across different platforms and can be easily analyzed.
Standards play an important role in waste management practices and test methods pertinent to handling residential, commercial, and industrial wastes. Integrated solid waste management concepts are being used by communities as they plan for their future waste disposal needs.
In conclusion, in all target countries where waste data collection and management systems are established or in ongoing improvement processes by stakeholders involved with proper disposal and recycling procedures, not only must standards be followed while managing such wastes but also concerning specifically aspects like providing more consistency among the subsets of all available information sources facilitates better-informed decisions from policymakers or stakeholders who want prudent planning towards community sustainability goals while reducing negative environmental impacts from trash heaps that attract vermin on streetscapes causing unsanitary circumstances.
Difficulty In Identifying And Categorizing Waste Materials
An essential aspect of waste management is identifying and categorizing waste materials to determine their proper disposal. This task can be challenging due to the varying types and quantities of waste generated in cities worldwide. Proper identification and categorization are critical for reducing environmental hazards and managing waste effectively.
To ensure that sustainable technologies reduce, reuse, and recycle appropriate components of solid wastes, properly structured, labeled data is vital to building reliable Artificial Intelligence (AI) models. However, data annotation tasks up to one-fourth of AI projects can range from manual annotations that are sensitive to human error or outsourcing annotation tasks placing an organization's internal and external operations at risk.
Furthermore, businesses seeking solutions for effective waste management encounter internal/external obstacles like personnel untrained in data processes required for labeling the information needed for AI models' development. The opportunity presents itself during crises such as COVID-19 outbreaks with automation steps leading manual intervention processes usable by non-technical staff aiding rapid yet efficient responses enhancing sustainable green economies.
Challenges In Collecting Accurate Data From Waste Management Facilities
The coronavirus pandemic has brought about significant disruptions in the way municipal solid waste management systems operate. The pandemic has created operational and managerial challenges, impacting daily health, productivity, and cleanliness of communities. One of the main issues that facilities face is collecting accurate data on their waste management activities.
Data annotation is a complex and inefficient process as it entails marking up raw data with critical information to enhance its usefulness. Facilities often struggle with insufficient data collection or poor quality aspects related to recycling. There is also the challenge of balancing recycling objectives while ensuring consumer protection from harmful substances found in recycled materials.
Waste sorting robots
Another challenge in waste management is energy recovery from waste. Energy conversion technologies such as biomass combustion or anaerobic digestion can extract value from organic components within the wastes while reducing greenhouse gas emissions associated with traditional disposal practices.
While there are numerous challenges facing waste management facilities today, organizations such as Energy Star Portfolio Manager are providing consistent metrics for evaluating these activities. It remains crucial that stakeholders within the industry continue collaborating on reducing inefficiencies and finding new ways of measuring performance accurately through innovative solutions such as big data analytics techniques to optimize resource utilization.
Limited Availability Of Skilled Personnel For Data Annotation
Data annotation is an essential part of data management, especially in waste management. Unfortunately, businesses can face several external and internal obstacles that make the task challenging, inefficient, and ineffective.
One of the significant challenges in waste management is limited availability of skilled personnel for data annotation tasks. Data annotation requires a combination of skills, knowledge, and effort to provide accurate labeling that helps with efficient decision making. However, finding properly trained personnel who can navigate complex datasets without errors is not easy.
Another challenge associated with this skill shortage is the time needed to properly train new hires to manage data correctly as any label inaccuracies may have negative consequences such as increased disposal costs or contamination risks affecting environmental health.
Therefore companies need solutions such as partnering with professional data annotation services that are well equipped for the handling complex datasets to provide quality labelling or collaboration with educational institutions to produce highly-skilled trainees who can come right into your business operations seamlessly.
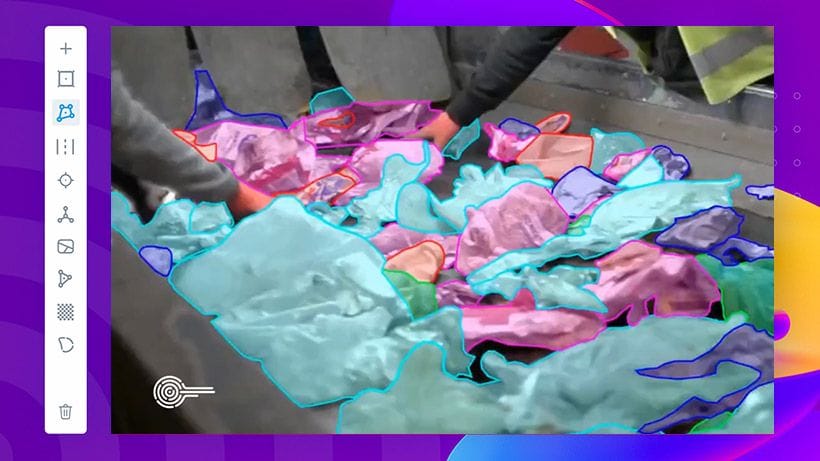
Keymakr's data annotation service is the ideal choice for waste management companies. Their project list includes partnerships with Recycleye, Waste Robotics, Waste Vision, and other waste management companies. As a result, there's no need to spend time and money on personnel training or data collection, as they can handle the data creation stage as well.
Integration Of Data Annotation With Waste Management Systems
To integrate data annotation with waste management systems, it is essential to address the challenges faced in waste management. Insufficient data collection and quality issues related to recycling pose significant challenges in accurately planning for future demand and appropriate management methods.
Data annotation is crucial in generating policies and effectively planning for waste management at a local level. Accurately understanding the types of waste generated and their volume enables effective decision-making in planning for its disposal. Data-related tasks consume a considerable amount of time in AI projects, with data labeling taking up to one-quarter of project time, highlighting the importance of addressing the challenges posed by insufficiently annotated data.
To overcome these difficulties, it is necessary to prioritize balancing quality and quantity when annotating data accurately. It requires going to the bottom of the problem - outsourcing video annotation specialists can prove helpful while also ensuring quick turnaround times, reducing manual workload assignments.
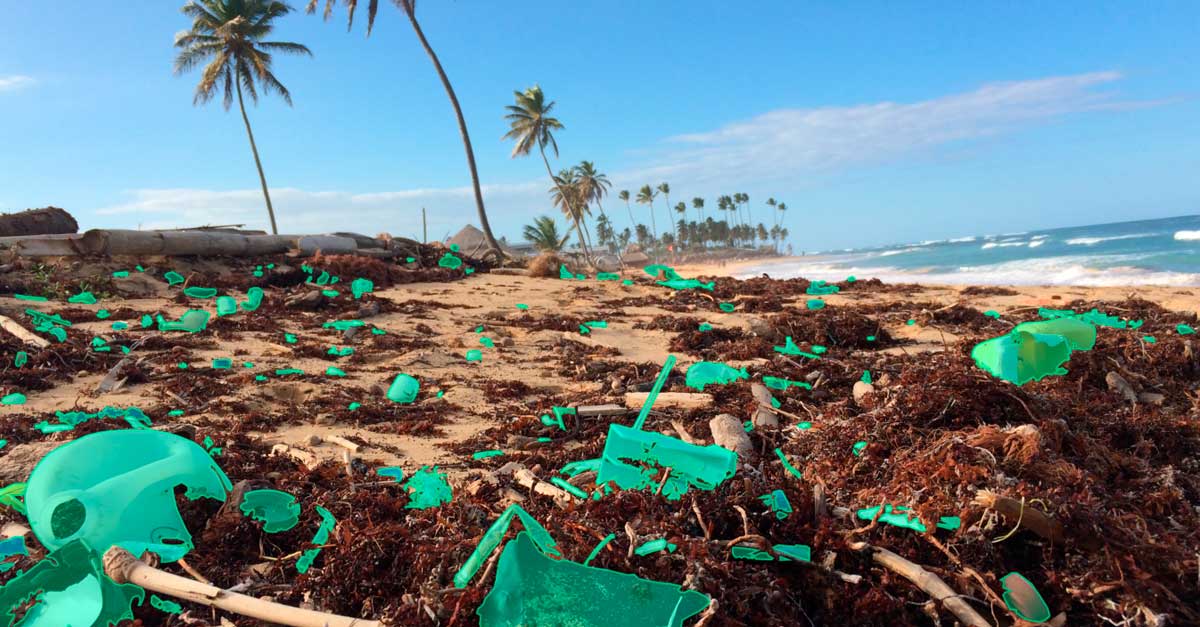
Integrating AI solutions into existing waste management systems will provide an accurate guide on which areas to channel resources such as infrastructure development for efficient delivery of services without spending unnecessary resources. By combining optimal technology use with effective policy creation based on well-annotated data sets, we can create more sustainable living spaces.
Importance Of Data Annotation For Effective Waste Management Strategies
Waste management data are vital for developing effective policies and planning for future demand. To achieve this, stakeholders in target countries have established waste data collection and management systems that continuously improve over time. Although businesses require an organized process of collecting and streamlining waste data annotation, it's often challenging to manage them effectively.
Creating a scalable budget strategy requires anticipating costs for data scraping, annotation tools, storage systems, and personnel training. An efficient national waste management strategy should also involve a participative process that aligns with the economic structure of each nation. The integrated waste management strategies must consider challenges such as funding mechanisms for infrastructural investments and opportunities like transitioning to the circular economy model.
As global e-waste is set to almost double by 2030, it highlights the importance of managing the growing volume of electronic wastes through sustainable measures. Accurate data annotation is needed to track progress towards recycling targets while keeping up-to-date on emerging technologies to minimize environmental impact. With well-planned life cycle assessments covering various stages from product creation through disposal or recycling along with better public policies around smooth information transfers amongst stakeholders will continue advancing sustainable materials management for sound environmental outcomes in physical transformations of resources into products people rely on day by day.
Comments ()