Video Annotation: In-depth guide and Use Cases
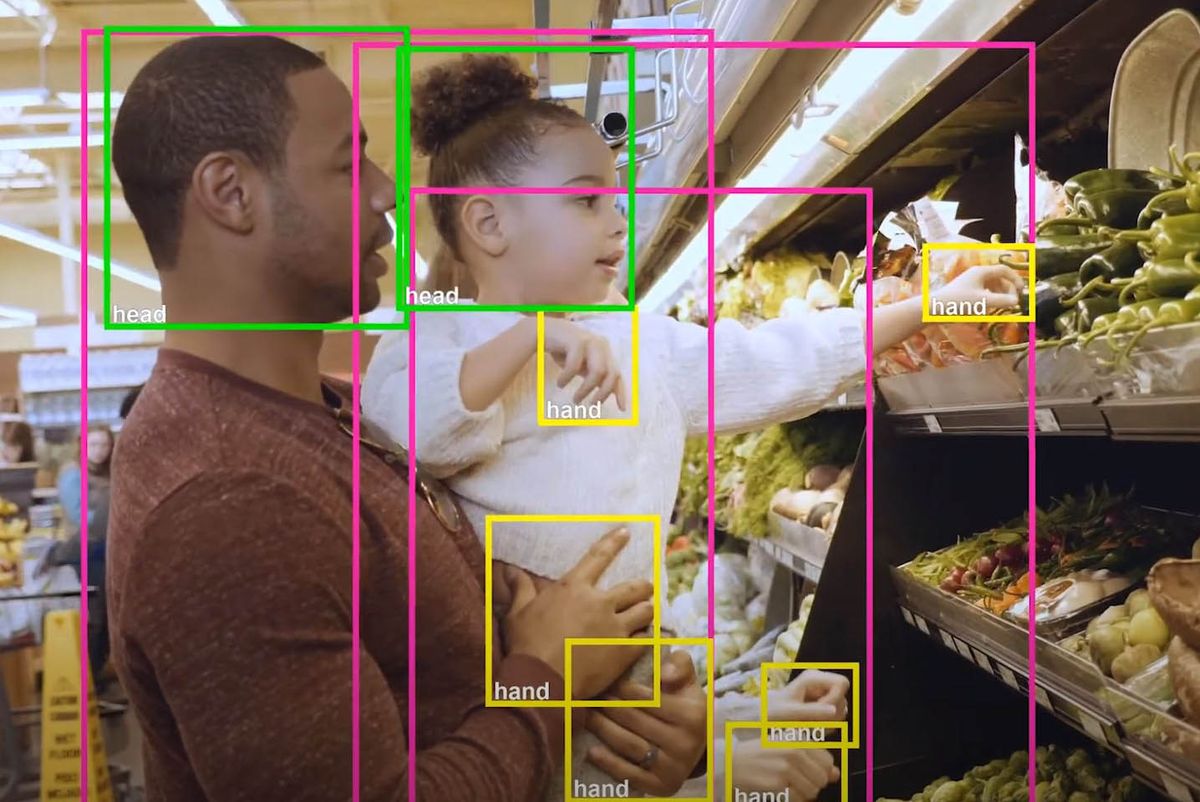
In this in-depth guide, we will explore the world of video annotation, its importance, and its various applications. We will delve into the different types of video annotation and the tools and techniques used for annotation. We will also discuss best practices for video annotation, including how to ensure accuracy and consistency.
Furthermore, we will examine the use cases of video annotation in various industries, from education and entertainment to marketing and healthcare. We will also explore the challenges and limitations of video annotation and how they can be overcome. Finally, we will look at the future of video annotation and its impact on technology.
To illustrate the power of video annotation, we will provide a case study of a successful implementation of video annotation in a business setting. By the end of this guide, you will have a comprehensive understanding of video annotation and its potential to transform the way we consume and interact with video content.
What Is Video Annotation And Why Is It Important?
Video annotation is the process of identifying and labeling objects within a video. It plays a crucial role in many industries, particularly in automotive and medical fields. This technology helps power autonomous driving systems by enabling vehicles to recognize objects on the road such as traffic signs, pedestrians, and other cars. Moreover, video annotation is used to monitor unsafe driving behavior or the driver's condition.
Apart from automotive use case, video annotation also serves as a tool for medical AI systems for identifying various diseases such as tumors or assisting surgeons during complex surgical procedures. Furthermore, it can be used to create checkout-free retail environments that offer convenience to shoppers without compromising security measures.
It's worth mentioning that annotations are necessary for Artificial Intelligence (AI) systems to make intelligent decisions based on visual information provided in images or videos. Video annotation requires more time, processing power and entails higher costs compared with image annotation due to complexity arising mainly from latencies associated with high frame rates typical in videos.
In general terms, video annotation plays an essential role in creating multimedia datasets necessary for Machine Learning algorithms that drive automation processes across different sectors of the economy. The use of Video Annotation goes beyond improving computer vision-based applications; it has broader implications across various industry branches where data-driven insights are critical factors driving future business growth potentials.
Types Of Video Annotation And Their Applications
Video annotation is a data annotation method widely used in computer vision. There are different formats for video annotation such as COC JSON, Pascal Voc XML, and Tensorflow TFRecord that allow for consistent and efficient labeling of videos.
Six types of video annotations are commonly used: frame level, bounding boxes, semantic segmentation, landmark, polyline, and 3D cuboids. Frame level video annotation involves labeling each frame with information such as object classification or tracking. Bounding boxes involve drawing rectangles around objects in a scene to indicate its location in the frame. Semantic segmentation involves labeling pixels within an image with categories like road or sky to enable accurate object detection.
Landmarks are points marked on specific features of an object such as ears or nose to aid facial recognition algorithms while polyline annotations involve marking the contour of objects like roads or buildings on maps. Finally, 3D cuboids provide depth information about objects in a scene.
Video annotation has numerous applications across industries like media where it's used for content analysis in broadcasting environments and machine learning where it's utilized for training models to recognize patterns and identify new information sources. It also finds its use in government surveillance systems where it serves as an assistive tool for authorities by aiding investigations by providing visual evidence and extract critical insights from large volumes of footage!
Tools And Techniques For Video Annotation
Video annotation tools and techniques are essential for training AI-powered algorithms to identify objects accurately. The global market for data annotation tools is projected to surpass $3 billion by 2028, highlighting their growing importance in the technology industry. Video annotations can be used for detection and tracking in various fields, such as medical AI and autonomous vehicle systems.
Common video annotation techniques include landmark annotation and semantic segmentation, which improve the accuracy, precision, and consistency of annotated videos. 2D bounding boxes are popular types of video annotations used to train object detection models effectively.
Keylabs data annotation platform allows users to annotate videos with object tracks quickly. It includes features like segmentation masks for detailed annotations like pixel-level instance or thing clicks that help solve problems like identifying similar-looking objects accurately.
Effective video annotations are necessary for high-performing AI applications increasingly integrated into our daily lives from virtual assistants to security systems through advanced neural networks trained on annotated data; therefore, accurate labeling is critical in achieving successful results using automated computer vision models as they continue improving technology further forward into the future.
Best Practices For Video Annotation
When it comes to video annotation, following best practices is crucial for accuracy and efficiency. Choosing easy-to-use software with functionality that suits your needs is the first step. Frame sampling strategy should be used to ensure enough labeled frames for ground truth. When selecting a tool, consider its efficiency and functionality.
Video annotation is important for AI and machine learning in detection and tracking, as well as labeling hidden objects. It's critical to capturing teaching practices on video which can provide benefits such as peer feedback and exploring “what-if” scenarios.
Moreover, annotations and AI video labeling are helpful in creating training datasets for classification, object detection, and recognition models. The use cases of video annotation are vast - from monitoring unsafe driving behavior to autonomous vehicles' collision braking systems. Therefore choosing software that enables you to upload videos easily, annotate videos & notify stakeholders efficiently are key factors in optimizing your video annotation workflow.
Overall noting the best practices mentioned above enable accurate ground truth data which thus contribute towards an efficient and effective machine learning or artificial intelligence model building process: leading overall higher success rate when using these algorithms for data-driven decision making processes specific to different applications ranging from healthcare industries to cutting-edge self-driving cars technologies providing a quicker response when identifying potential driving hazards on the roadways before impacts take place ultimately leading towards saving lives on an everyday basis ensuring a safer environment within our surroundings!
Use Cases Of Video Annotation In Various Industries
Video annotation is a powerful tool used in several industries, including autonomous vehicles, medical imaging, robotics, and drones. It provides more contextual information than image annotation and is useful for video object detection, tracking, and predicting movements.
In the field of autonomous vehicles, video annotation plays a crucial role in training machine learning algorithms for object recognition. Video annotation enables AI to recognize objects in real-time while collecting data on traffic patterns and monitoring driver behavior. This technology has revolutionized the way we drive by increasing safety measures with collision braking systems.
In medicine, video annotation is used to enhance medical imaging diagnostics by identifying specific structures within images or videos. Medical professionals use this information to make informed decisions on treatment plans for optimal patient care. Additionally, drones equipped with video annotated data can be used to map difficult terrain or disaster-stricken areas to bring relief efforts more effectively.
Despite the complexities involved with annotating videos -- such as its time-consuming nature -- professional services are available that help overcome these challenges. In education too innovative technicalities like video annotations provide students access to advanced learning techniques aiding deep understanding of classroom concepts using visual representation.
Overall video annotation serves an important purpose across multiple industries beyond just those mentioned here. Its adoption will continue broadening from present-day uses ensuring efficient objective detection becoming an essential aspect of modernizing innovations providing improved accessibilty and enhancing digitalization advancements for business purposes as well as educational influences.
Challenges And Limitations Of Video Annotation
Video annotation poses several challenges and limitations, which impact its efficacy in training Artificial Intelligence (AI) models. Compared to image annotation, video annotation is more complex, time-consuming, and has higher processing latency. This complexity arises from the need to capture the temporal redundancy present in videos effectively. In addition, video frames contain low information contribution to the neural network per frame compared to images.
Accurate annotation requires expertise and knowledge of different kinds of AI models. These models require a certain level of complexity during training phase or deployment phase. Developing annotation guidelines for Medical Video Annotation is an especially challenging task as it necessitates identifying a surgeon's intent from visual cues alone without relying on additional documentation or data.
Properly categorized and labeled datasets enhance model accuracy in specific use cases through targeted learning algorithms for medical devices or robotics development such as human-robot collaboration or surgeon's education program by providing accurate annotations that describe every aspect of visual data with text labels indicating tool usage alongside anatomical landmarks.
Overall while video annotation may be challenging there are many potential use cases such as developing Healthcare Robotic systems that support surgeries within hospitals or improving accuracy rates on Convolutional Neural Networks (CNNs) used within radiology departments worldwide. Thus mitigating current challenges and limitations will open machine learning technology towards new applications leading to safer medical treatments for all patients.
Future Of Video Annotation And Its Impact On Technology
Video annotation is an essential part of teaching AI and ML systems to recognize objects in videos accurately. It involves labeling all the objects on a frame-by-frame basis, which is a time-consuming and challenging task that requires careful attention to detail. Two types of annotations are generally used: 2D and 3D boxes, with 3D offering more accuracy but being more complex.
The annotated video data can be used in multiple applications, including training autonomous vehicles and medical AI. However, the use of video annotation involves many complexities compared with image annotation, making it difficult for ordinary businesses without expertise in tech not opting for outsourcing partners like CloudFactory.
Technological innovations such as cloud computing have helped foster scalability and solve infrastructure issues for video technology labeling over recent years. This has allowed for greater innovation in the industry while improving scalability by reducing costs associated with hardware acquisition while increasing speed through distributed networks. Overall, video annotation holds much potential for the future of technology development across diverse industries if applied appropriately.
Case Study: Successful Implementation Of Video Annotation In A Business Setting
One popular use case for video annotation is in autonomous vehicle systems. Properly annotated videos create a high-quality reference database that these systems can refer to when making real-time decisions. This can help improve the safety and efficiency of self-driving cars.
Another important use case for video annotation is in medical AI. By annotating medical images and videos, AI algorithms can accurately identify anomalies and assist doctors in making diagnoses. This can help improve the speed and accuracy of medical treatment.
One successful implementation of video annotation in a business setting was with an e-commerce company looking to improve their product recommendation system. They used video annotation to label objects and people in product videos, which allowed them to better understand customer preferences and make more accurate recommendations. This led to a significant increase in sales and customer satisfaction.
It's important for businesses looking to implement video annotation to acknowledge potential labeling challenges, such as difficult lighting conditions or overlapping objects on screen. Additionally, models should be trained as part of a continuous learning workflow so that they can adapt over time based on new data inputs. Ultimately, being data-centric is key when implementing video annotation in a business setting, as it allows for more accurate predictions and better decision-making overall.
Comments ()