Navigating the Trends: Data Annotation Jobs in 2024
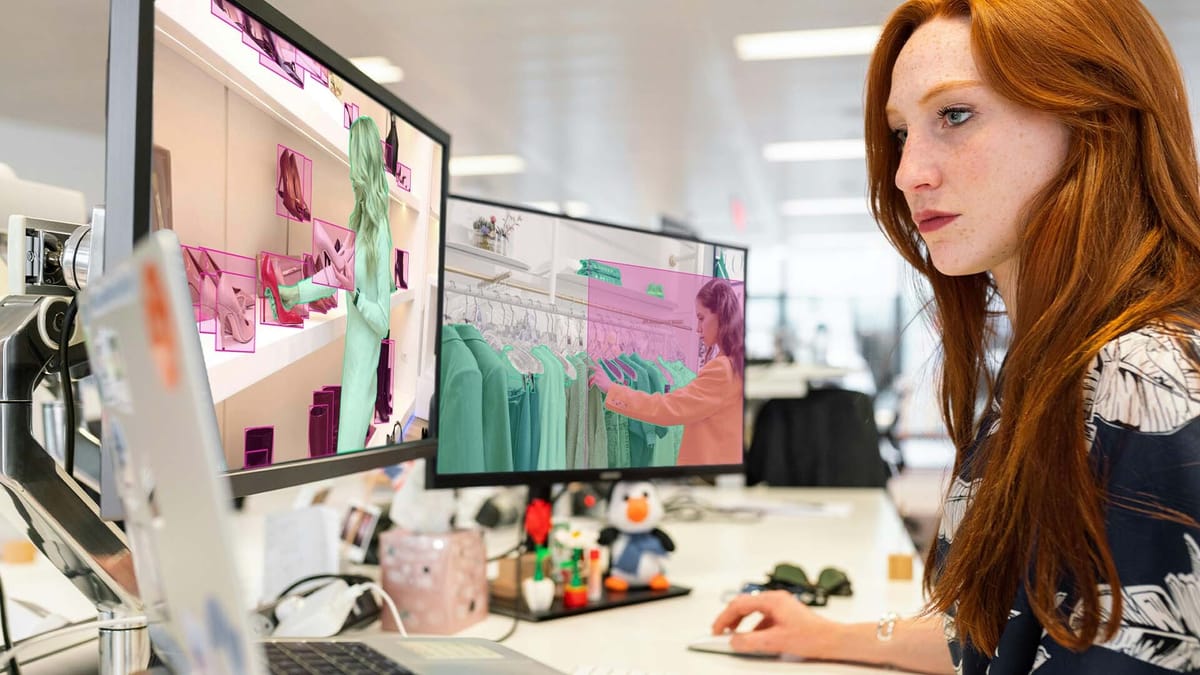
By 2028, the data annotation market could reach a shocking USD 8.22 billion. This big jump shows how much organizations need data annotation pros to make AI better. Sectors like health and cars are getting more into this. With more use of digital images and mobile tech, jobs in data annotation are growing fast. And as we head into 2024, knowing what's new in data annotation jobs is key. This area helps improve AI from small projects to big ones.
In 2024, the data labeling job scene is very competitive. People in these jobs can make $15 to $70 per hour. Those in top positions, like ML Data Operations Leads, might make $117,000 to $350,000 each year. Remote data annotation jobs are now as well-paying as jobs in the office. This shows how work is changing. For those with 2 years or more in the field, the future looks bright. More than ever, companies need skilled data annotators for accurate AI training data annotation.
Key Takeaways
- The global data annotation market is projected to reach USD 8.22 billion by 2028.
- Hourly rates for data annotation roles in 2024 range from $15 to $70.
- Remote data annotation jobs offer competitive salaries and flexible work arrangements.
- Data annotators in high demand due to the necessity for accurate AI training data.
Introduction to Data Annotation in 2024
Data annotation is growing fast. It's gone from niche to key in AI development. This change shows how essential labeling data is for making better AI. It helps us develop stronger AI for many areas.
Overview of Data Annotation
Data annotation tags data for AI to use. This includes text, images, videos, and sounds. The market for this work will likely hit USD 8.22 billion by 2028.
Every day, over 4 billion people online create a huge amount of data. Structuring this data is vital. It powers AI in things like customer service and driving cars safely.
Importance in AI and Machine Learning
Data labeling is a key part of setting AI on the right path. It makes AI better at what it does. This is crucial as many businesses worldwide now use AI in their work.
- Text Annotation: Text labeling improves chatbots, making them better at helping users.
- Image Annotation: It's key for spotting and understanding things, important in cars that drive themselves and in medical tests.
- Video Annotation: It helps computers see things in moving pictures, making them understand the videos better.
- Audio Annotation: The need for labeling speech and sounds is growing fast, showing more people want this service.
Thorough labeling is important in many fields. For medical tools or spotting fraud in finance, good labels are crucial. Retail, car making, and making things work better use these labels a lot too.
AIs are getting smarter too. They now learn from human feedback. This includes systems like ChatGPT. Such methods highlight the need for precise data annotation.
Sector | Application | Impact |
---|---|---|
Healthcare | Image Recognition | Enhanced diagnostics |
Retail | Sentiment Analysis | Better customer insights |
Automotive | Object Detection | Safer autonomous driving |
Finance | Fraud Detection | Improved security |
Data labeling's importance hints at a booming market by 2030. As we want more from AI, quality data labeling stays crucial.
Factors Driving Annotated Data Demand in 2024
In 2024, the need for annotated data is crucial for AI and machine learning growth. The increase in dataset volume and complexity is a big part of this. So is the need for quick data annotation and more automation in labeling. All these factors show how important data annotation is and how it meets industry needs.
Increasing Complexity of Datasets
Datasets are getting more complex, driving the need for annotated data. It's estimated that by 2025, world data volume will hit 175 zettabytes, with over 90% being unstructured. With such large and complex data, sectors like self-driving cars and medical imaging need detailed annotation. This helps them handle massive amounts of data efficiently.
Real-time Data Annotation Needs
There's a growing demand for real-time data annotation as datasets become more complex. This need is critical during data collection. It makes sure models learn from the freshest data, important in fields like digital commerce and customer analysis. Real-time annotation boosts model accuracy, meeting changing consumer demands.
Automation in Data Labeling
Automation in data labeling is changing the game, cutting down manual work and boosting efficiency. Tools with AI can make annotation twice as fast and more accurate. Yet, having people oversee this process is key for accurate and reliable annotation, especially for tough jobs. Balancing automation and human work is crucial, showing the importance of crowd annotation in the industry.
These points highlight the important, key role data annotation plays in the tech world's future for 2024.
Generative AI’s Impact on Data Labeling in 2024
Generative AI is changing how we label data, especially now in 2024. It makes data labeling faster and more precise using AI models. This means working with AI helps get labeling tasks done better and quicker.
Automated Data Labeling with GenAI
Generative AI is making a big difference in labeling data automatically. For example, OpenAI's DALL-E is making image labeling better without much human effort. Text data labeling is also improving a lot thanks to models like GPT-4o and BERT.
By 2030, the data annotation services market could grow to US$ 5.3 billion. This shows how big automated data labeling might be. Tools like Snorkel Flow show how we can use generative AI to label data more accurately and quickly.
Human-AI Collaboration
Even with all these advances, human skills are essential. Together, people and AI make sure data is labeled correctly. This is key as the amount of unstructured data we manage could double by next year. Human-AI teamwork makes sure we get accurate labels on complex data, blending AI speed with human judgement.
In 2040, McKinsey says generative AI might be as good as people in the top 25% of tasks. We're seeing this happen now as we use AI to handle complex tasks in data annotation better. AI helps us do more, creating chances for innovation and efficiency in data labeling.
It's vital to know how to combine generative AI with human expertise. This mix is becoming more important for data annotation's future. We rely on this balanced teamwork to keep up with the industry's changing needs.
Top Trends in Data Annotation Jobs in 2024
In 2024, we're seeing big changes in the data labeling field. One key trend is the growth of unstructured data. Experts predict companies will deal with double the unstructured data next year. Forrester points this out as a key factor driving the need for annotation experts.
Improvements in handling visual data are also crucial. Technologies like generative AI are making data annotation easier and faster. McKinsey even says that by 2040, this AI could work as well as the top 25% of humans.
The job market for data annotation is set to grow big, thanks to AI. By 2030, the market could be valued at USD 5.3 billion. This really shows how important accurate data labeling is for improving AI across many fields.
Looking at the job side, there's a wide range of pay in data annotation. You could make between $15 and $70 an hour, depending on the job and where it is. If you reach top positions like a Machine Learning Data Operations Lead, you could earn from $117,000 to $350,000 every year - but that goes far beyond annotation and into managing all data ops up to the model's deployment.
The need for high-quality data is booming, especially with advanced AI. Companies have to be very careful in their data annotation work to keep up. The market for data annotation tools is set to hit USD 3.4 billion by 2028, showing its growing importance.
- Increasing volume of unstructured data
- Generative AI's impact on reducing manual annotation tasks
- Rapidly growing market value and job opportunities
- Highly variable earning potentials based on roles and expertise
For those in the field, staying updated with these trends is crucial. In 2024, data annotation jobs offer great growth chances thanks to new tech and more complex data needs.
Remote Data Annotation Opportunities
The world of data annotation has opened up. Thanks to fast internet and online platforms, working remotely is easier than ever. Now, people from all over can take part in these tasks.
Market Projection and Growth Rates
The data annotation market is set for strong expansion. With more focus on AI, businesses will see a lot more unstructured data. Right now, about 40% of all businesses worldwide use AI. This shows the big need for data annotators to keep up with the demand.
Regional Developments and Opportunities
In places like India, there's huge growth in data labeling jobs. It's expected to be a big market, exceeding USD 7 billion by 2030. India's strong foundation is a key reason for this. It has good support for small and medium enterprises. As a result, more jobs and investors are likely to come.
Below is an informative table summarizing the critical data tagging job growth statistics:
Statistic | Data |
---|---|
Global Market Value by 2028 | USD 8.22 billion |
Expected CAGR through 2030 | 26.6% |
Market Worth by 2030 | USD 5.3 billion |
Percentage of Organizations Using AI | 40% |
Managed Unstructured Data Increase by 2025 | Twofold |
Impact of Automation on Data Annotation Workforce
Automation is changing how we annotate data. It's making things faster and more efficient. Tasks that once took a long time can now be done much quicker. This means we can work with a lot of data without spending as much time or money.
Increased Efficiency and Productivity
Automation has greatly boosted how efficient and productive we are in data annotation. It can do complex jobs like sorting images and understanding text. Professionals in this field can now focus on quality checks rather than manual work.
Job Displacement and New Skill Requirements
Yet, automation also means some jobs might change or even disappear. People working in data annotation will need to learn new things to cope. They'll need to understand more about AI and keep learning about new tech.
Key Impact Areas | Details |
---|---|
Efficiency | Tasks reduced from hours or days to a fraction of the time. |
Consistency | Ensures uniform annotations across datasets, enhancing AI model reliability. |
Scalability | Allows efficient handling of large datasets with reduced labor costs. |
Job Evolution | Requires annotators to oversee and validate automated processes, demanding new skills. |
Cost Savings | Eliminates extensive human intervention, reducing labor costs significantly. |
Data Annotation for Large Language Models (LLMs)
The rise of Large Language Models (LLMs), such as GPT and Gemini, has made data annotation crucial. Natural Language Processing (NLP) relies heavily on accurate annotations. They are key for properly training and improving LLMs.
Role of Annotations in LLM Training
Annotations are vital for teaching LLMs. They add labels to data, helping models understand how we use words. For instance, they teach models the meaning behind social media posts and legal documents. Thanks to these labels, LLMs can create text that feels more human.
Specific Job Roles in LLM Annotation
The world of LLMs has given rise to special job roles. People work as data evaluators and trainers to make models better. Their tasks include checking data quality and customizing models for specific needs, like in law or healthcare.
- Data Evaluators: They focus on keeping the data correct, checking and fixing annotations to ensure models learn well.
- Trainers: These experts adjust the models for specific tasks, making them smarter for things like legal reviews or healthcare.
- Model Testers: They make sure the models are correct by comparing their answers to known good answers.
Humans are still critical in training AI, a trend expected to grow. The need for accurate data annotation is increasing rapidly. This demand shows how crucial good annotations are for the advancement of AI.
LLMs with special annotations are helping in many fields. For example, in healthcare, chatbots provide better care. In law and academics, these models help with document reviews and understanding complex data. This shows the wide-reaching impact of good annotations.
Adding the right labels to LLMs leads to better outcomes and makes it easier to use these models. This approach also overcomes the challenge of finding enough labeled data. By providing a solid basis for NLP tasks, tailored annotations meet the needs of various industries.
Summary
In 2024, data annotation jobs are changing fast because of new technology and trends. Automation and AI are making a big impact, especially in how we label different types of data. This change is key for handling a mix of data, from images to text to audio and video.
We will see more specific annotation tools for different fields like healthcare, finance, and more. These tools will help meet the unique needs of each industry. They will focus on checking for mistakes and being fair when labeling.
There will also be more ways to keep data private but still useful for projects. These methods will follow strict privacy laws like GDPR and CCPA. They plan to hide personal details when needed.
Collaborative platforms for labeling data will become more popular. They help teams work together better, especially when they are not in the same place. This teamwork will keep the quality of work high.
The future of data labeling looks bright. Being part of this field means you're helping teach AI, which is a big deal for our future technology. Stay sharp by learning new things, and your career in data annotation will grow as the industry does.
FAQ
What are the current trends in data annotation jobs?
Advancements in AI are shaping data annotation job trends for 2024. Datasets are getting more complex. Real-time data annotation needs are also increasing.
Besides, the use of crowdsourcing for data annotation is growing. Businesses are tapping into diverse talents. They do this to improve their AI models in many fields.
How important is data annotation in AI and machine learning?
Data annotation is crucial for strong AI systems. It turns messy data into something machine learning can use. This step is key to training accurate models. These models are used in various areas like autonomous vehicles or retail.
What factors are driving the demand for annotated data in 2024?
More complex datasets, a need for real-time annotation, and automation are key. These make businesses look for skilled annotators. They want high-quality data for training their AI models.
How is generative AI impacting data labeling in 2024?
Generative AI is changing data labeling. It cuts down on manual work. It allows human-AI teamwork.
What are the top trends in data annotation jobs in 2024?
Trends in data annotation jobs are changing. They include handling more unstructured data and advanced visual processing. Generative AI is also making its mark. These trends are affecting the types of jobs and demand in the field.
What are the opportunities for remote data annotation jobs?
Remote work is opening up new opportunities in data annotation. Professionals can work from anywhere using the internet and cloud platforms.
This trend is attractive for balancing work and life. It also connects professionals to a global job market.
What are the job growth statistics for data tagging?
The job market for data tagging is growing fast. It's expected to reach USD 8.22 billion by 2028. There will be big growth in India's market too. This is because of a strong infrastructure and support for businesses.
How is automation affecting the data annotation workforce?
Automation is making data annotation more efficient. But it also brings challenges like job changes.
The workforce is adapting by learning new skills. They focus on overseeing and checking automated work. This ensures the data remains accurate and of high quality.
What role do annotations play in training Large Language Models (LLMs)?
Annotations are key in training LLMs. They provide the labeled data models need. This helps models understand context and create text that seems human. Roles like evaluator and trainer are critical. They keep the training data quality high and improve model-user interactions.
Comments ()