Mastering Data Annotation: Techniques for AI Success
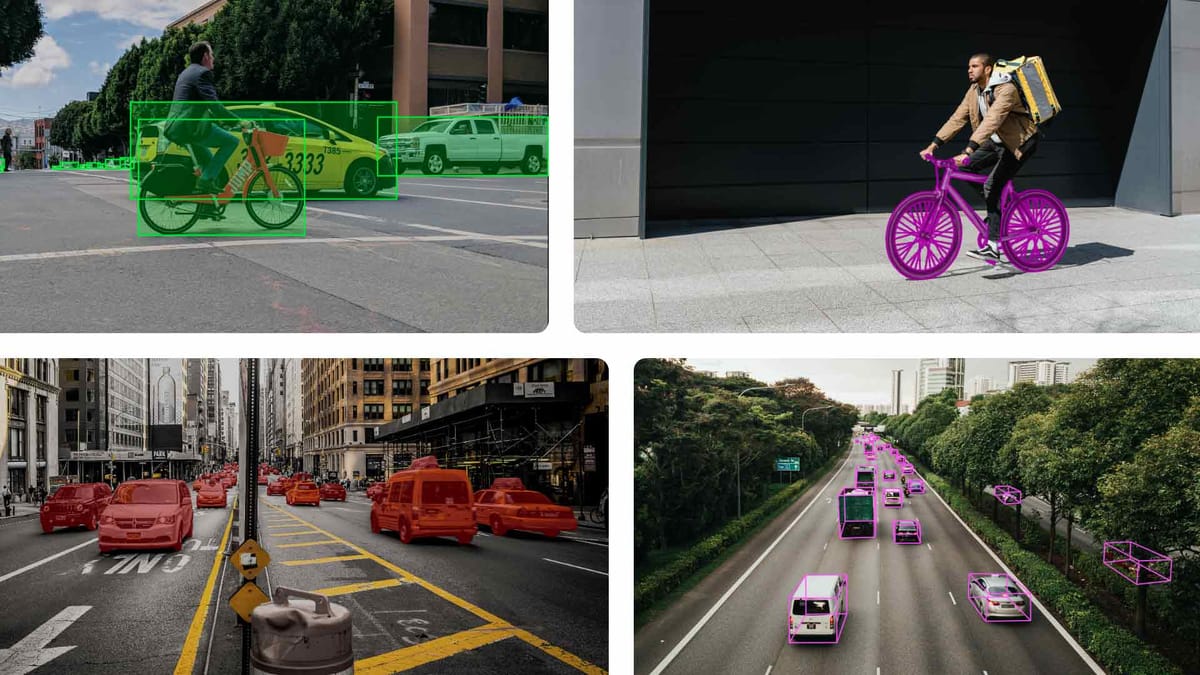
Data annotation is a critical step in enhancing the accuracy and performance of AI and machine learning models. By properly labeling and annotating data, businesses can train their models to recognize patterns and make accurate predictions. Advanced data annotation strategies, such as computer vision and natural language processing, play a crucial role in the success of AI projects. Implementing these techniques can optimize the performance of AI models and improve overall results.
Key Takeaways:
- Data annotation is essential for improving the accuracy and performance of AI models
- Computer vision and natural language processing are advanced data annotation techniques
- Proper implementation of data annotation strategies can optimize AI model performance
- Data annotation plays a crucial role in the success of AI projects
- Advanced data annotation techniques improve overall AI results
The Importance of Data Annotation
Data annotation serves as the foundation for AI and machine learning models, playing a critical role in their success and performance. Without effective data annotation, these models would struggle to interpret and understand the data they are trained on. Properly labeling and annotating data provides the necessary information for AI models to learn from and make accurate predictions. By following best practices for data annotation, businesses can optimize the performance of their AI projects and achieve optimal results.
"Data annotation is crucial for AI project success as it enables models to understand and process information accurately."
One of the key benefits of data annotation is the creation of labeled data, which serves as the input for training AI and machine learning models. Labeled data provides the necessary context and information for AI models to make informed predictions and decisions. Whether it is annotating images, videos, text, or audio data, the process of data annotation ensures that AI models have the knowledge and understanding needed to perform effectively.
Following best practices in data annotation is essential to achieve accurate and reliable results. Establishing clear annotation guidelines helps maintain consistency and ensures that all labeled data adheres to the same standards. Implementing quality control measures, such as spot-checking and site monitoring, helps identify and rectify any inconsistencies or errors in the annotated data.
An example of a best practice in data annotation is establishing inter-annotator agreement, where multiple annotators label the same data to ensure consistency and accuracy. This approach helps identify any discrepancies or ambiguities in the annotation process and fosters collaboration and knowledge-sharing among annotators.
Additionally, leveraging advanced data annotation techniques, such as computer vision and natural language processing, can significantly enhance the performance of AI models. Computer vision techniques enable the annotation of visual data, while natural language processing techniques focus on annotating text and audio data. These techniques contribute to the development of more robust and accurate AI models.
Overall, data annotation is a crucial step in the AI project lifecycle, ensuring the success and accuracy of AI and machine learning models. By following best practices, leveraging advanced techniques, and utilizing labeled data, businesses can unlock the full potential of AI and achieve remarkable outcomes in various industries.
Benefits of Data Annotation | Best Practices | Advanced Techniques |
---|---|---|
1. Enables AI models to learn and make accurate predictions | 1. Establish clear annotation guidelines | 1. Computer vision |
2. Creates labeled data for training AI models | 2. Implement quality control measures | 2. Natural language processing |
3. Improves performance and results of AI projects | 3. Foster inter-annotator agreement | 3. Advanced image and text annotation |
Key Data Annotation Techniques
When it comes to data annotation, businesses have access to various techniques that can enhance the accuracy and performance of their AI models. In this section, we'll explore two key data annotation techniques: computer vision and natural language processing.
Computer Vision
Computer vision is a data annotation technique that focuses on annotating visual data, such as images and videos. By labeling and annotating these visual elements, AI models can understand and process visual information, enabling them to recognize objects, extract features, and make informed predictions. Image annotation is a crucial component of computer vision, allowing AI models to identify and classify objects, detect boundaries, and track movements.
Natural Language Processing
Natural language processing (NLP) is another data annotation technique that revolves around annotating text and audio data. NLP involves the annotation of text to help AI models comprehend and generate human-like language. By annotating text with semantic information, key phrases, and named entities, NLP enables AI models to understand context, sentiment, and linguistic structures. Text annotation ensures that AI models can accurately interpret and respond to textual inputs with natural language generation and understanding capabilities.
In summary, computer vision and natural language processing are two valuable data annotation techniques for enhancing AI model performance. While computer vision enables models to process visual information, natural language processing empowers models to comprehend and generate human-like language.
Best Practices for Data Annotation
Implementing best practices for data annotation is crucial to ensure accuracy and reliability in AI projects. By following these guidelines, businesses can optimize their data annotation process and maximize the value of their labeled datasets.
1. Establish Clear Annotation Guidelines
Setting clear annotation guidelines is essential to maintain consistency and accuracy across labeled data. These guidelines provide annotators with specific requirements and criteria for annotating data accurately. By ensuring a standardized approach, businesses can enhance the quality and reliability of their annotated datasets.
2. Train Annotators for Expertise
Training annotators is a vital step to ensure that they are familiar with the specific requirements of the project. Providing comprehensive training programs helps annotators understand the annotation guidelines, labeling techniques, and the overall project objectives. With expertise in place, annotators can consistently deliver high-quality annotations.
3. Implement Quality Control Measures
Implementing quality control measures is essential to maintain the integrity and reliability of annotated data. Spot-checking and site monitoring are effective ways to identify and correct any errors or inconsistencies in the annotations. Regular quality control assessments help maintain the accuracy and validity of the labeled datasets.
"Establishing clear annotation guidelines and providing proper training to annotators are key components of successful data annotation. By implementing quality control measures, businesses can ensure the reliability and integrity of the annotated data."
By following these best practices, businesses can streamline their data annotation process and achieve accurate results. The table below provides a summary of these best practices for easy reference.
Best Practices | Description |
---|---|
Establish Clear Annotation Guidelines | Ensure consistency and accuracy by setting clear guidelines for annotators. |
Train Annotators for Expertise | Provide comprehensive training programs to enhance annotators' skills and understanding. |
Implement Quality Control Measures | Regularly assess and monitor annotated data to maintain its accuracy and integrity. |
By adhering to these best practices, businesses can ensure the accuracy and reliability of their data annotations, ultimately leading to better AI model performance and project success.
The Role of Data Annotation Tools
The development of data annotation tools has revolutionized the annotation process, significantly improving efficiency and accuracy. These tools leverage automation and AI-powered annotation platforms to streamline the annotation workflow and reduce the time and effort required. By incorporating these advanced tools, businesses can enhance productivity and achieve higher quality labeled data.
Data annotation tools, such as annotation software and AI-powered annotation platforms, employ sophisticated automation techniques to expedite the annotation process. With the assistance of machine learning algorithms, these tools assist annotators in labeling data more quickly and effectively. By reducing manual labor and repetitive tasks, annotation tools optimize efficiency and allow annotators to focus on more complex annotation tasks.
"The use of data annotation tools has significantly improved our annotation process. We have experienced a substantial increase in efficiency and accuracy, leading to more reliable AI models."
Automation introduced by data annotation tools not only improves efficiency, but also enhances overall data annotation efficiency. These tools can handle large volumes of data with minimal human intervention, allowing businesses to process data at scale. By automating certain aspects of the annotation process, such as pre-labeling or suggesting annotations, these tools enable annotators to work more swiftly and efficiently.
Furthermore, data annotation tools promote efficient collaboration among annotators and improve consistency in labeling. With centralized platforms and intuitive interfaces, these tools facilitate communication and coordination between annotators, ensuring uniformity in the annotation guidelines and minimizing discrepancies or errors that may arise during the annotation process.
Data Annotation Tools | Benefits |
---|---|
Annotation Software | Streamlines the annotation process, reduces manual effort, and improves accuracy. |
AI-Powered Annotation Platforms | Leverages machine learning algorithms to assist annotators, enabling faster and more effective annotation. |
Automation | Automates repetitive tasks, increasing efficiency and allowing annotators to focus on more complex annotation. |
Efficient Collaboration | Facilitates communication and coordination among annotators, ensuring consistency in labeling and guidelines. |
Data Annotation Process at Keymakr
Keymakr, a leading data labeling company, employs a meticulous data annotation process to ensure accurate and reliable annotations. With a team of skilled annotation experts, Keymakr follows a comprehensive checklist to maintain the highest quality standards throughout the annotation process.
"Accuracy and reliability are at the core of our data annotation process. We understand the critical role labeled data plays in training AI models, and our experts are committed to delivering the best results."
The Data Annotation Checklist
The checklist followed by Keymakr's annotation experts covers every crucial aspect of the data annotation process:
- Understanding project requirements: Keymakr starts by gaining a deep understanding of the client's specific needs and objectives. This allows them to tailor the annotation process accordingly.
- Collecting high-quality data: Keymakr ensures the collection of high-quality and diverse data suitable for training AI models effectively. This includes leveraging Keymakr's extensive data resources or working with client-provided datasets.
- Establishing annotation guidelines: Clear and well-defined annotation guidelines are crucial for maintaining consistency across annotations. Keymakr establishes comprehensive guidelines to ensure accurate labeling and annotation of data on proprietary data annotation platform Keylabs.
- Training annotators: Keymakr provides extensive training to annotators, ensuring they have an in-depth understanding of the annotation guidelines and requirements. This training equips them with the necessary skills to effectively annotate data.
- Performing continuous quality control: To ensure the highest level of annotation accuracy, Keymakr performs continuous quality control measures. This includes spot-checking annotations, providing feedback to annotators, and resolving any discrepancies.
- Real-time collaboration: Keymakr enables real-time collaboration between annotators and project managers. This facilitates seamless communication and ensures that any queries or challenges are addressed promptly.
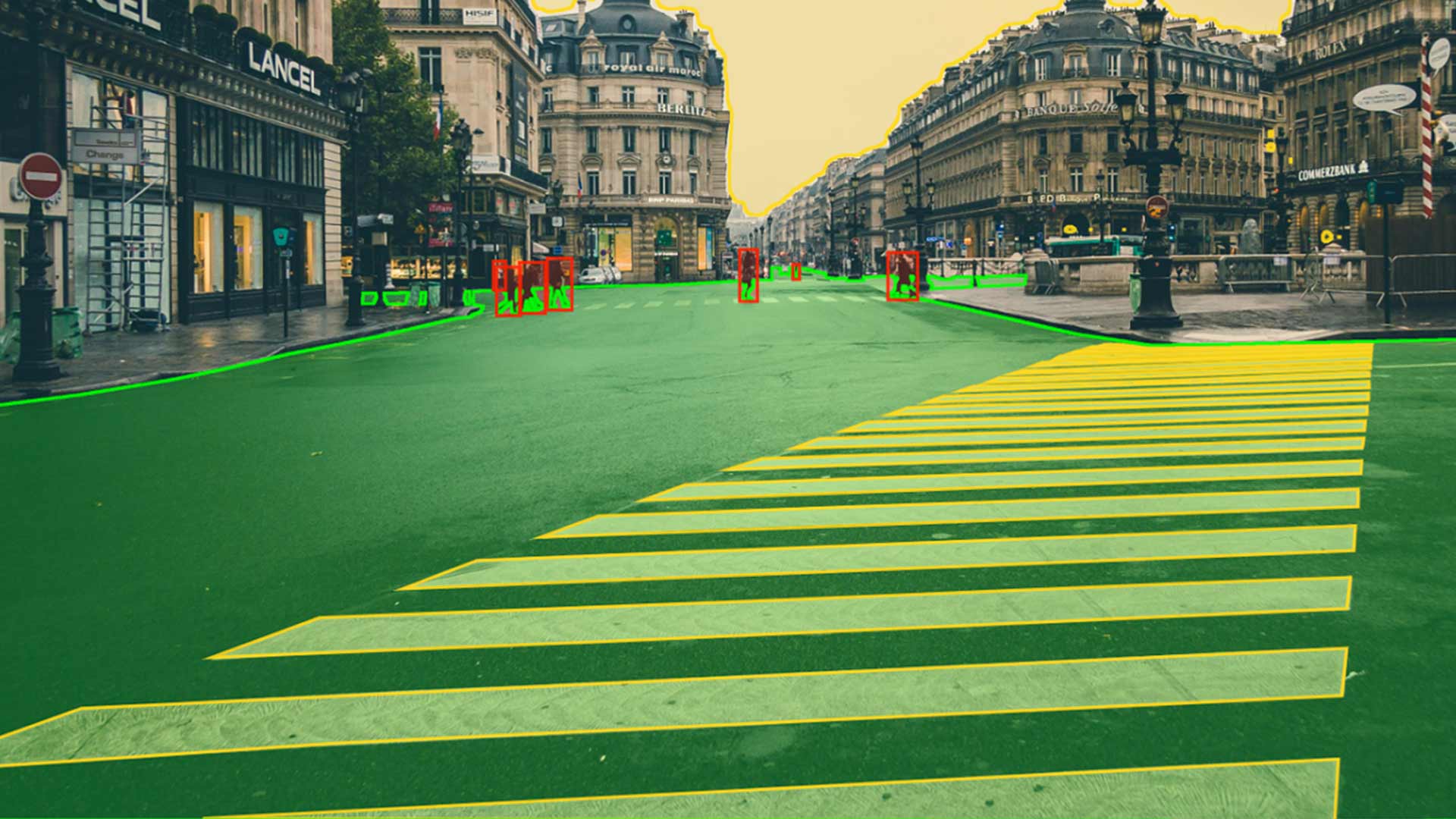
Industry-leading Tools and Techniques
Keymakr utilizes advanced data annotation software Keylabs and AI-powered tools to streamline the annotation process:
- Annotation software: Keymakr employs sophisticated annotation software Keylabs that enables efficient annotation of various data types, including images, videos, and text.
- AI-powered annotation platforms: By harnessing the power of AI and machine learning, Keymakr utilizes annotation platforms that assist annotators in automating repetitive tasks and improving annotation efficiency.
- Specialized annotation techniques: Keymakr offers specialized annotation techniques, such as semantic segmentation and named entity recognition, to cater to specific AI application needs.
By combining the expertise of their annotation professionals with state-of-the-art tools and techniques, Keymakr delivers high-quality annotated datasets that drive the success of AI projects.
Streamlining the Annotation Process
Streamlining the annotation process is crucial for optimizing productivity and minimizing human error in data annotation tasks. Keymakr, a leading annotation service provider, has developed an efficient workflow and utilizes cutting-edge annotation tools to deliver exceptional results to their clients.
The Annotation Workflow in ScaleAI
ScaleAI follows a well-defined annotation workflow that ensures the smooth and systematic execution of data annotation tasks. The workflow consists of the following steps:
- Annotation Planning: Before starting the annotation process, ScaleAI plans the annotation strategy, considering factors such as annotation guidelines, label consistency, and project requirements.
- Annotation Execution: With a team of experienced annotators, ScaleAI precisely labels and annotates the data according to the established guidelines. The annotators pay meticulous attention to detail, ensuring accurate and consistent annotations.
- Quality Control and Review: ScaleAI implements stringent quality control measures to minimize human error. The annotated data undergoes thorough review and spot-checking to identify and rectify any discrepancies or inconsistencies.
- Delivery: After completing the annotation process, ScaleAI delivers the high-quality annotated dataset to their clients, meeting their specific project deadlines.
By following this streamlined annotation workflow, ScaleAI ensures the efficient and accurate annotation of data, maximizing the value of the labeled dataset.
Efficiency and Minimized Human Error in Data Annotation
The use of automation features in annotation tools reduces the time and effort required for manual annotation, resulting in quicker turnaround times and increased productivity.
Minimizing human error is crucial for maintaining data accuracy and overall annotation quality. Quality control measures, including thorough review and spot-checking of annotated data, ensure the identification and resolution of any errors or discrepancies. This commitment to precision and accuracy enhances the reliability of the annotated dataset and contributes to the success of AI projects that rely on this data.
The Value of Manual Work in Data Annotation
Despite advancements in automated data annotation techniques, manual work continues to play a crucial role in data annotation. Human annotators bring expertise and accuracy to the process, especially when dealing with highly specialized data. Their dedication to the project ensures precise and meaningful annotations, resulting in high-quality labeled datasets. Automated techniques may streamline the process, but human annotators provide the precision and nuance that machines struggle to replicate.
Manual data annotation is invaluable when it comes to handling complex and nuanced data. Highly specialized domains, such as medical imaging or legal documents, often require human expertise to accurately annotate intricate details that automated systems might overlook. Human annotators possess the domain-specific knowledge necessary to understand the context and make accurate annotations.
"Manual annotation allows us to capture the subtle nuances that automated systems may miss. It requires the expertise of trained professionals to interpret complex information, making it an essential component in the data annotation process."
In addition to expertise, human annotators bring a level of accuracy that is unparalleled. They can spot and rectify potential errors, ensuring the quality and integrity of the labeled datasets. With their attention to detail and meticulous approach, human annotators contribute to the creation of reliable datasets, which subsequently enhance the performance of AI models.
Moreover, human annotators are capable of understanding the wider context and specific requirements of each AI project. They can adapt their annotation techniques to suit the objectives and constraints of the project, resulting in annotations that align with the desired outcomes. By tailoring the annotations to project requirements, human annotators contribute to the overall success of AI initiatives.
Expertise and Experience Speak for Themselves
When it comes to data annotation, the human touch cannot be underestimated. The value of manual work becomes evident through the expertise and experience that human annotators bring to the table. By leveraging their knowledge, precision, and attention to detail, businesses can enhance the accuracy and reliability of their AI models.
In an industry where accuracy and performance are paramount, human annotators play a vital role. While automated techniques have their merits, they cannot replicate the nuanced understanding and domain-specific expertise that human annotators possess. By combining automated techniques with the skills of human annotators, businesses can capitalize on the strengths of both approaches to maximize the potential of their AI projects.
Manual data annotation: human annotators bring expertise and accuracy to the process.
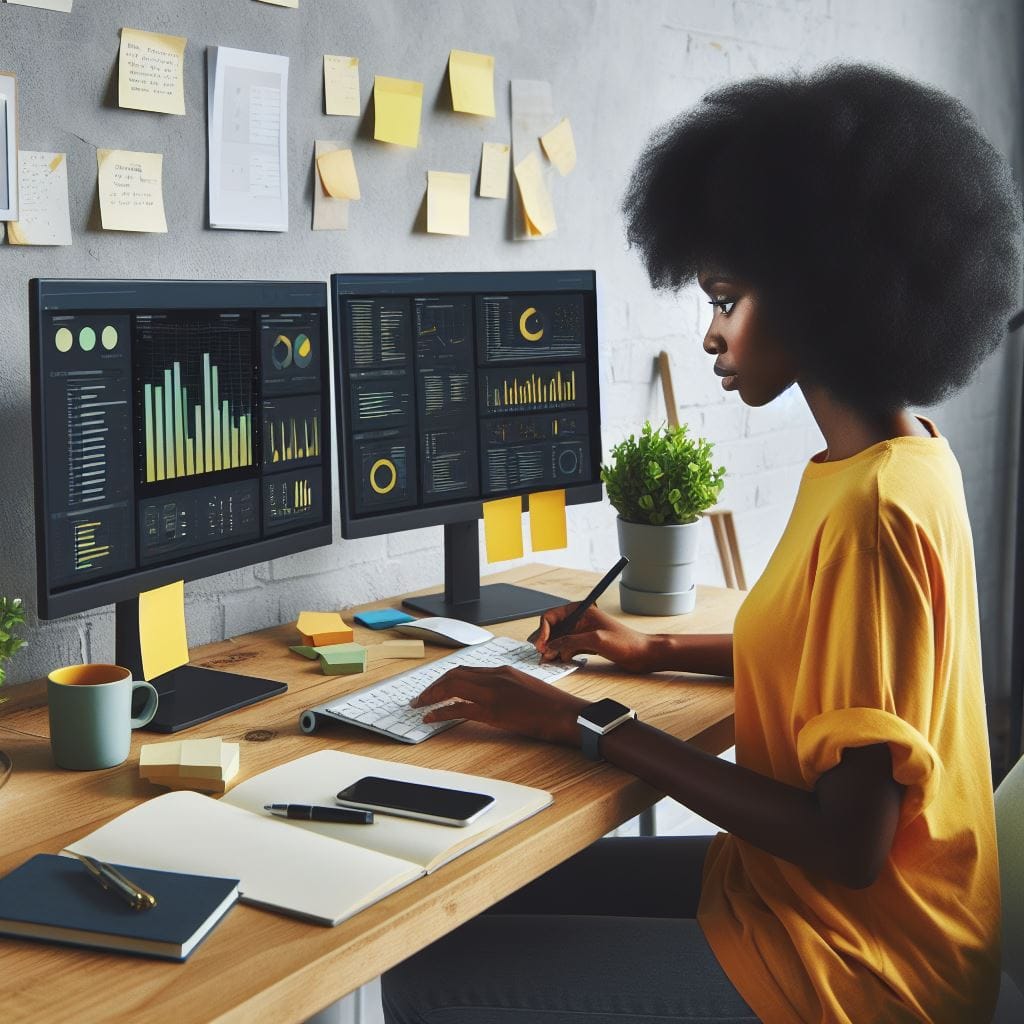
Expert Insight
The expertise and accuracy brought by human annotators significantly contribute to the success of AI projects, providing a foundation for building robust and reliable models.
The Role of Human Annotators in AI Projects
Human annotators play a vital role in the development and training of AI models. Their expert insight and deep understanding of project requirements enable them to accurately label and annotate data, improving model performance. Unlike automated techniques, human annotators possess the ability to interpret nuances and context that machines often struggle with. Their critical thinking skills and domain expertise allow them to make informed decisions during the annotation process, driving the creation of high-quality labeled datasets.
"Human annotators contribute valuable domain expertise and critical thinking skills that enhance the accuracy and reliability of AI models."
By leveraging the expertise of human annotators, businesses can overcome challenges related to complex datasets and specialized domains. These annotators have the ability to handle intricate data types and intricate labeling tasks, ensuring accurate annotations that align with the project's objectives. The human touch provided by annotators adds a layer of understanding and precision to the data annotation process, resulting in improved model performance and more successful AI projects.
Maximizing Model Performance through Human-Annotated Data
Research has shown that human-annotated data plays a crucial role in optimizing the performance of AI models. When models are trained on accurately labeled datasets created by skilled human annotators, they demonstrate higher accuracy and reliability. The meticulous nature of human annotators ensures that the training data is consistent, diverse, and representative of real-world scenarios.
With expert insight and careful considerations, human annotators can handle challenging tasks such as named entity recognition, sentiment analysis, and object detection with precision. By fine-tuning the annotations based on project requirements and target performance metrics, human annotators contribute to the development of models with superior capabilities.
Human Annotators: The Key to AI Success
In the constantly evolving field of AI, the role of human annotators remains invaluable. Their expertise, critical thinking skills, and commitment to understanding project requirements result in data annotations that enable AI models to perform at their best. By partnering with human annotators, businesses can harness their insights to build robust, reliable, and accurate AI models that drive success in various industries and applications.
Benefits of Human Annotators | Challenges Addressed |
---|---|
Domain expertise | Handling complex datasets |
Critical thinking skills | Interpreting nuanced data |
Contextual understanding | Ensuring accurate annotations |
Adaptability to specialized domains | Meeting project requirements |
Conclusion
Data annotation is a critical process in enhancing the accuracy and performance of AI models. By following best practices, implementing advanced techniques, and leveraging data annotation tools, businesses can achieve optimal results.
The value of manual work in data annotation should not be overlooked, as human annotators bring expertise and accuracy to the process. Their attention to detail and domain knowledge ensure precise annotations that machines struggle to replicate.
With the right approach and thorough data annotation, businesses can unlock the full potential of AI and machine learning to drive innovation and transform industries. By harnessing the power of labeled data, AI models can make accurate predictions, recognize patterns, and deliver meaningful insights that fuel growth and success.
FAQ
What is data annotation?
Data annotation is the process of labeling and annotating data to enhance the accuracy and performance of AI and machine learning models.
Why is data annotation important for AI models?
Data annotation provides the necessary information for AI models to learn and make accurate predictions, improving their understanding and interpretation of the data they are trained on.
What are some data annotation techniques?
There are various techniques, including computer vision for annotating visual data, and natural language processing for annotating text and audio data.
What are best practices for data annotation?
Best practices include establishing clear annotation guidelines, training annotators, and implementing quality control measures to ensure consistency and accuracy.
How do data annotation tools enhance the annotation process?
Data annotation tools automate aspects of the annotation process, increasing efficiency and reducing time and effort required. They leverage machine learning algorithms to improve accuracy.
What is the value of manual work in data annotation?
Human annotators bring expertise and accuracy to the process, especially with highly specialized data, providing precise and meaningful annotations.
Conclusion
Data annotation is a critical process in enhancing the accuracy and performance of AI models. By following best practices, implementing advanced techniques, and leveraging data annotation tools, businesses can achieve optimal results. The value of manual work in data annotation should not be overlooked, as human annotators bring expertise and accuracy to the process. With the right approach and thorough data annotation, businesses can unlock the full potential of AI and machine learning to drive innovation and transform industries.
Comments ()