Managing a Successful Data Annotator Team
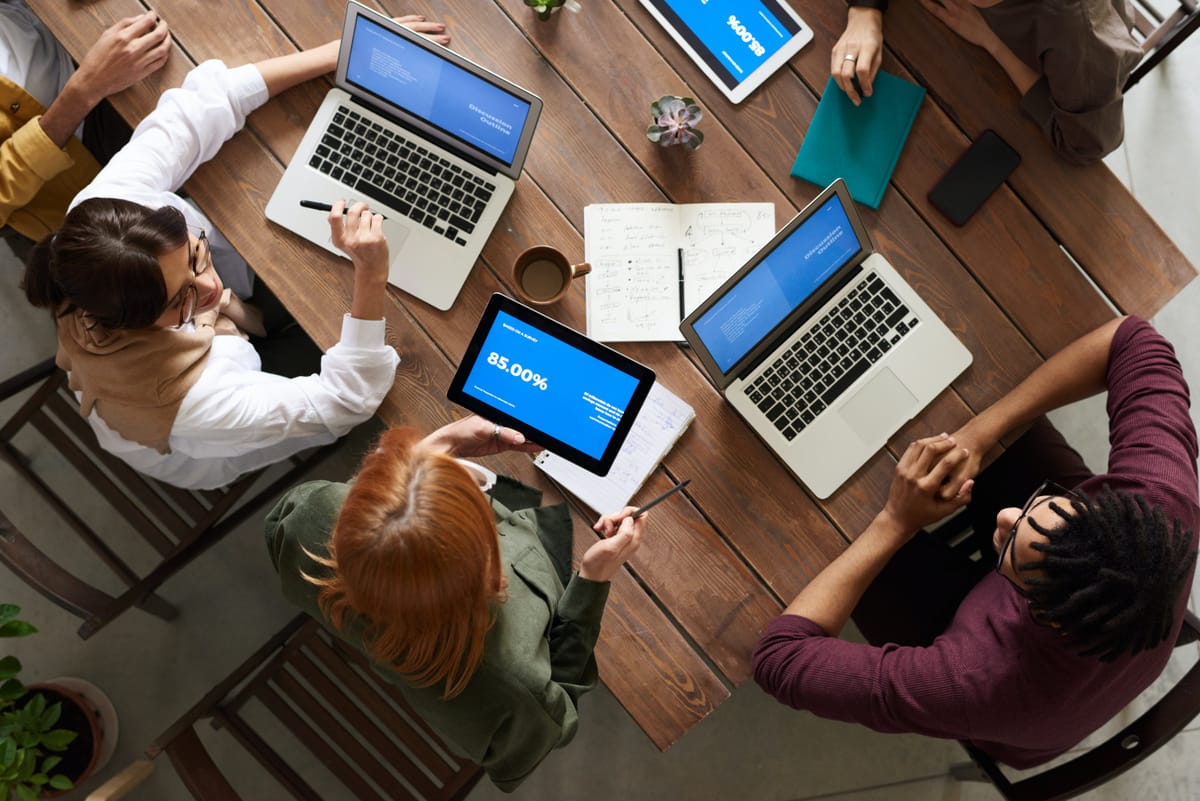
In this article, we will explore expert strategies for managing a data annotator team to boost efficiency and productivity in data labeling projects. We'll provide insights from industry experts and discuss best practices for data annotation, team collaboration, and project management.
Key Takeaways:
- Data annotation teams are essential for accurate data labeling and categorization.
- Effective management and collaboration enhance team productivity.
- A carefully planned data annotation process ensures consistent and accurate results.
- Quality assurance and continuous iteration are vital for reliable machine learning models.
- Choosing the right data annotation platform plays a crucial role in project success.
The Role of a Data Annotator Team Manager
In the realm of data annotation, the data annotator team manager plays a crucial role in ensuring seamless collaboration between the data science team and the annotation teams. With their expertise, they facilitate the flow of labeled data, which is essential for training language models and driving advancements in AI technology.
The data annotator team manager is responsible for fulfilling the data requirements of the data science team. They oversee the process of providing labeled data, which serves as the foundation for training language models. This involves curating datasets that align with the specific needs of the data science team, ensuring that the models are equipped with accurate and comprehensive training data.
Moreover, the manager plays a pivotal role in establishing effective communication channels between the annotators and the data science team. They facilitate annotator feedback loops, allowing for constant improvement and refinement of the labeling process. By incorporating feedback from the annotators, the manager ensures that challenges and obstacles are promptly addressed, leading to enhanced annotator performance and increased overall efficiency.
The Importance of Annotator Feedback Loops
Annotator feedback loops serve as a valuable mechanism for the data annotator team manager to gather insights and suggestions from the annotation teams. This feedback aids in refining the labeling interface, making it more user-friendly and intuitive. By actively seeking input from the annotators, the manager fosters a collaborative environment that empowers the team and helps optimize their performance.
Through continuous evaluation and improvement of the labeling interface, the data annotator team manager enhances the efficiency and accuracy of the annotation process. This enables the team to produce high-quality labeled data, which is vital for the successful training of language models and the development of cutting-edge AI applications.
To further support the annotators in their tasks, the manager ensures that they have a comprehensive understanding of the annotation guidelines. Clear and concise guidelines not only streamline the labeling process but also minimize errors and promote consistency in the labeled data.
The manager's role is not limited to administrative tasks alone but extends to providing support and addressing any questions or concerns raised by the annotators. Swift and effective support enables the team to overcome any obstacles they may encounter during the annotation process, ensuring uninterrupted productivity and progress.
Annotator Performance and the Role of the Manager
Annotator performance is crucial in delivering accurate and reliable labeled data. The data annotator team manager plays an active role in monitoring and evaluating the performance of annotators, ensuring that they meet the required standards and deliver consistent results.
By implementing performance metrics and evaluation criteria, the manager can objectively assess annotator performance and identify areas for improvement. This enables targeted training and feedback, which enhances the skill set of the annotators and elevates the overall quality of the labeled data.
Benefits of an Efficient Data Annotator Team | Annotator Performance | Quality of Labeled Data |
---|---|---|
Enhanced efficiency and productivity | Continuous evaluation and improvement | Increased accuracy and reliability |
Streamlined collaboration between teams | Targeted training and feedback | Consistency in labeled data |
Optimized use of resources | Swift support and problem-solving | Improved performance of language models |
The interplay between the data annotator team and the manager is integral to the success of data annotation projects. A skilled and efficient manager navigates the intricacies of data science and annotation, enabling effective collaboration, optimizing annotator performance, and ultimately contributing to the development of innovative language models.
Importance of Building a Data Annotation Team
Building a data annotation team is essential for accurate labeling and categorization of data. This process enables easier analysis and generates reliable results, directly impacting the quality of data models. By having a dedicated team, businesses can ensure that the data used in their models is accurate, up-to-date, and aligned with their specific use cases.
The expertise and commitment of the data annotation team significantly contributes to the overall success of data-driven decision-making. The team's thorough understanding of accurate labeling and categorizing data enhances the reliability of the results and analysis conducted.
Accurate labeling is a crucial aspect of data annotation that ensures the correct identification of relevant information and attributes within the data. By employing a skilled team, businesses can confidently rely on the annotated data for insights and predictions in various scenarios.
"The level of accuracy in data labeling directly impacts the quality and effectiveness of the resulting data models. Having a dedicated data annotation team not only ensures accurate labeling but also enables businesses to extract reliable insights and make informed decisions based on the analyzed data."
Furthermore, categorizing data appropriately facilitates efficient data analysis and empowers businesses to uncover valuable patterns, trends, and correlations. The team's expertise in categorization ensures that the data is classified correctly, leading to more meaningful and reliable analysis results.
Reliable results from data analysis enable businesses to make informed decisions and develop data-driven strategies. By having a dedicated data annotation team, businesses can confidently rely on the accuracy and quality of their data models, fostering enhanced performance and success in various business use cases.
Overall, building a data annotation team plays a vital role in ensuring accurate labeling and categorization of data, which leads to reliable results and improved data models. The expertise and commitment of the team contribute to the overall success of data-driven decision-making in various business use cases.
Benefits of Building a Data Annotation Team |
---|
Accurate labeling |
Categorizing data effectively |
Generate reliable results |
Enhanced data models |
Up-to-date and accurate data |
Business-specific use case alignment |
Estimating Training Data Needs for ML Models
The amount of training data required for machine learning models is influenced by the complexity and specific requirements of each project. To determine the necessary data volume, there are estimation guidelines that can be considered. These guidelines provide a starting point, but it is crucial to consult with technical partners and data annotation team members to determine the optimal input data size.
For smaller models, a general rule of thumb suggests using approximately 10 times the data compared to the number of degrees of freedom in the model. This provides an adequate amount of training data to capture the necessary variations and patterns.
When working with larger models, consideration should be given to both the number of images used for training and their size. Image parameters, such as color channels, need to be taken into account as they affect the complexity and information content of the data.
Estimation guidelines provide rough estimations to begin with, but it is essential to keep in mind that the exact data volume needed can vary based on the uniqueness of each project and the specific objectives. Consulting with technical experts and leveraging the expertise of the data annotation team can help refine these estimations and achieve the optimal training data volume for machine learning models.
Note: The table below illustrates the estimation guidelines for determining training data needs based on model complexity.
Model Complexity | Training Data Volume |
---|---|
Small Models | Approximately 10 times the number of degrees of freedom |
Large Models | Varies based on the number and size of images, accounting for image parameters like color channels |
Managing Data Drift in ML Models
Data drift is a phenomenon that occurs when the characteristics of the data used for training a machine learning model differ from the data it encounters in the real world. This can lead to a decline in model accuracy and hinder its performance in practical applications. In order to ensure accurate predictions and maintain model relevance, it is crucial to effectively manage data drift.
The responsibility of monitoring and addressing data drift falls on the shoulders of the data annotation team. By regularly testing the model using real-world data, they can identify any discrepancies between the training data and the data the model encounters in the field. This ongoing monitoring process helps detect and address any inaccuracies caused by data drift before the model is deployed to production.
Monitoring data drift involves comparing the data characteristics of the training data and the real-world data. By analyzing the differences, the annotation team can make strategic adjustments to the model or the training dataset to ensure optimal performance.
"Data drift occurs when the characteristics of the data used for training differ from the data the model is being used on."
"To ensure accurate predictions and maintain model performance, data annotation teams play a vital role in monitoring and addressing data drift."
One effective approach is to use a data annotation platform that allows for easy monitoring and tracking of data drift. These platforms provide tools to help the annotation team analyze the data predictions and compare them with the real-world data. By visualizing the differences and identifying patterns, the team can take appropriate actions to address any drift and improve model accuracy.
By actively managing data drift, the data annotation team contributes to the overall success of the machine learning project. Accurate and reliable predictions are essential for making informed business decisions and delivering valuable results.
Data Drift Challenges | Monitoring Techniques |
---|---|
Changing distributions of real-world data | Regular testing of the model using real-world data |
Shifting correlations between features | Comparing data characteristics of the training data and real-world data |
Evolution of target variables | Analyzing data predictions and comparing them with real-world data |
Concept drift | Visualizing differences and patterns in data predictions |
By continuously monitoring and addressing data drift, the data annotation team plays a critical role in maintaining model accuracy and relevance. Their expertise and proactive approach contribute to the overall success of the machine learning project.
Benefits of a Dedicated Data Annotation Team
Having a dedicated data annotation team offers several advantages. First and foremost, such a team ensures that annotation requirements are met adequately and within the set timeframe. This level of dedication and focus allows for consistent and accurate labeling across datasets, which is crucial for producing reliable and usable data for machine learning purposes.
Data labeling can be a challenging task, often involving subjective decisions. A diverse team with varying backgrounds and expertise brings different perspectives to the table, mitigating the risk of bias and ensuring a more comprehensive understanding of the dataset. This diversity helps address the data labeling challenge by identifying areas that require additional annotation or clarification, resulting in more accurate and reliable data.
Furthermore, a dedicated data annotation team brings an increased level of commitment and professionalism to the task. The team members can collaborate and seek clarification or guidance from one another, enhancing the overall quality and usability of the data. This collaborative approach fosters a supportive environment, enabling team members to learn from each other and continually improve their annotation skills.
"A dedicated data annotation team ensures consistent and accurate labeling, bringing together diverse perspectives to address data labeling challenges."
Overall, the benefits of a dedicated data annotation team include efficient and timely annotation, consistent and accurate labeling, diversity of expertise, dataset clarification, and improved data usability. By leveraging the strengths and collaboration of a dedicated team, businesses can maximize the potential of their data and unlock valuable insights for machine learning applications.
Options for Building a Data Annotation Team
Building a data annotation team is a critical step in ensuring accurate and efficient data labeling. However, organizations have several options to consider when it comes to establishing this team. Each option has its own pros and cons, and the choice depends on project goals, budget, requirements, and complexity.
In-House Annotation
One option is to hire and train full-time annotators within the organization. In-house annotation offers the advantage of consistency and management control over the annotation process. With an in-house team, there is direct oversight, and the team can closely align with the organization's goals and requirements. However, this option can be costlier since it involves additional expenses such as hiring, training, salaries, and benefits.
Freelancers
Hiring freelancers is another option that provides flexibility and cost-effectiveness. Freelancers can be engaged on a project basis, allowing organizations to scale the annotation team to match the project's needs. This option is particularly suitable for projects with fluctuating workloads or short-term demands. However, quality control and consistency can be challenging when working with a diverse pool of freelancers.
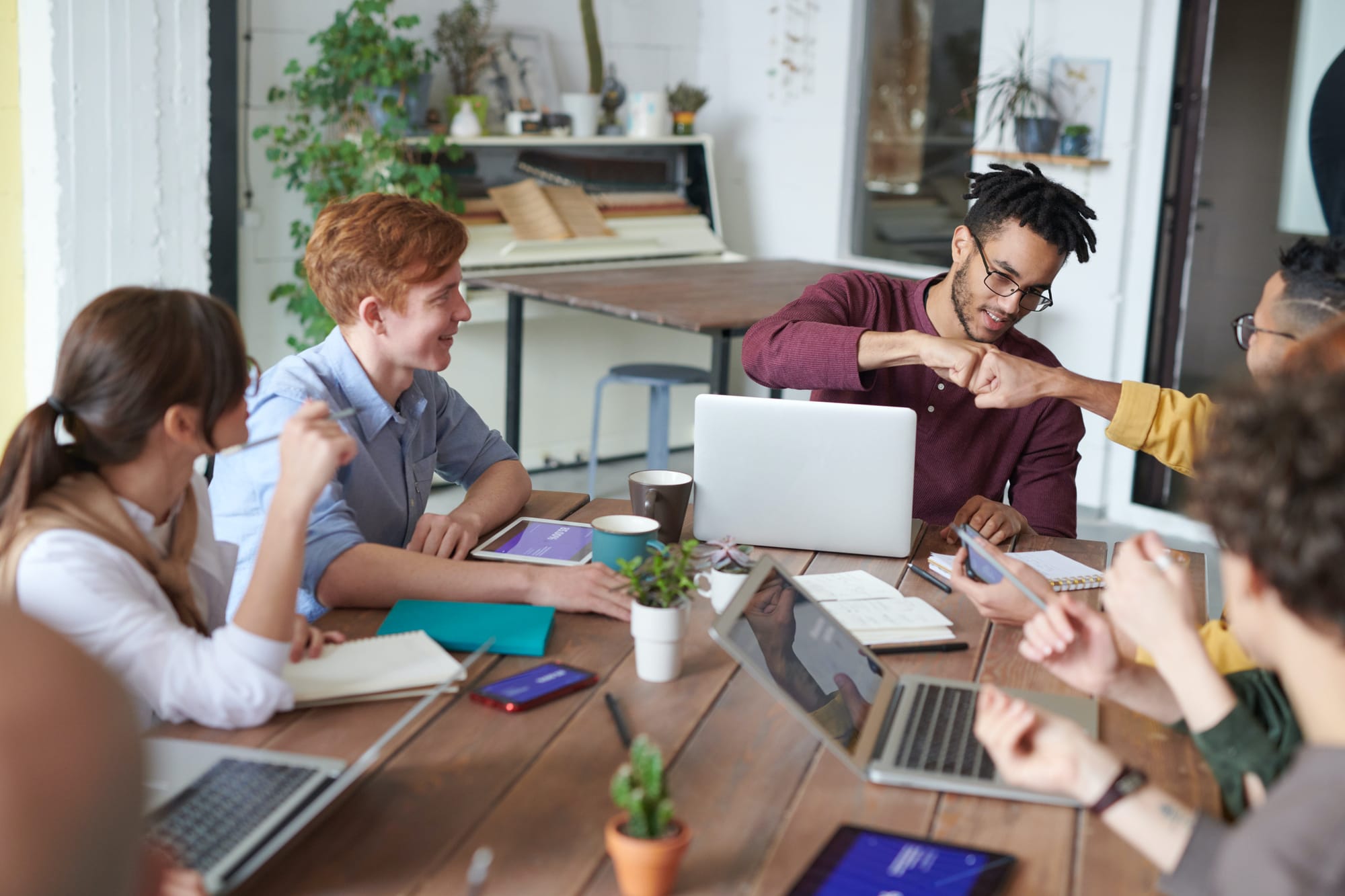
Outsourcing
A third option is to fully outsource the annotation task to professional annotation services. This approach offers turnkey solutions, expertise, and a dedicated team focused on annotation. By outsourcing, organizations can leverage the knowledge and experience of specialized annotation providers. However, this option comes with a higher price tag and potential limitations in tool integration and customization. Organizations should carefully evaluate the provider's capabilities and ensure alignment with their project requirements.
Pros and Cons
Here is a summary of the pros and cons of each option:
In-House Annotation
Pros:Consistency and management controlAligned with organization goalsCons:Higher cost
Freelancers
Pros:Flexibility and cost-effectivenessScalability for fluctuating workloadsCons:Quality control and consistency challenges
Outsourcing
Pros:Turnkey solutions and expertiseDedicated team focused on annotationCons:Higher price tagPotential limitations in tool integration
Ultimately, the choice of building a data annotation team depends on various factors, including the project's specific needs, budget constraints, and the desired level of control and customization. Organizations should carefully evaluate the pros and cons of each option and select the most suitable approach that aligns with their goals and requirements.
Establishing an Effective Data Annotation Process
A well-defined data annotation process is crucial for achieving consistent and accurate results. To ensure clear instructions and labeling conventions, the workforce needs guidance and support to maintain consistency and adhere to quality standards. By establishing a comprehensive process, businesses can streamline their data annotation efforts and maximize workforce productivity.
Clear instructions play a vital role in guiding annotators throughout the annotation process. These instructions should provide detailed information on labeling conventions, highlighting specific requirements and guidelines for accurate data annotation. By providing clear and concise instructions, businesses can minimize ambiguity and enhance the quality of labeled data.
[labeling conventions, clear instructions]
In addition to clear instructions, adequate training procedures are essential for the data annotation team. Training should be provided to familiarize annotators with the labeling tool and ensure they understand the guidelines and requirements. This training should be comprehensive and ongoing to address any challenges or questions that may arise during the annotation process.
[training procedures]
Quality control measures are crucial for maintaining the accuracy and reliability of labeled data. Regular monitoring and evaluation of annotator performance should be implemented to identify areas for improvement and provide timely feedback. This feedback loop helps address any challenges or errors, ensuring the consistency and quality of the annotated data.
[quality control]
Guidelines accessibility is another important aspect of establishing an effective data annotation process. Annotators should have easy access to the guidelines and documentation necessary for their tasks. This accessibility promotes a collaborative and supportive working environment, enabling annotators to perform their tasks efficiently and accurately.
[guidelines accessibility]
Process documentation is critical for ensuring transparency and consistency in the data annotation process. Thoroughly documenting the process and keeping it regularly updated facilitates easy access for annotators and other team members. It also allows for better management of evolving protocols and fosters collaboration among the data annotation team.
[process documentation]
Implementing Quality Assurance in Data Annotation
Quality assurance plays a vital role in ensuring the accuracy and reliability of data annotation projects. By implementing effective quality control measures, organizations can enhance the overall quality of labeled data, leading to the development of robust and trustworthy machine learning models.
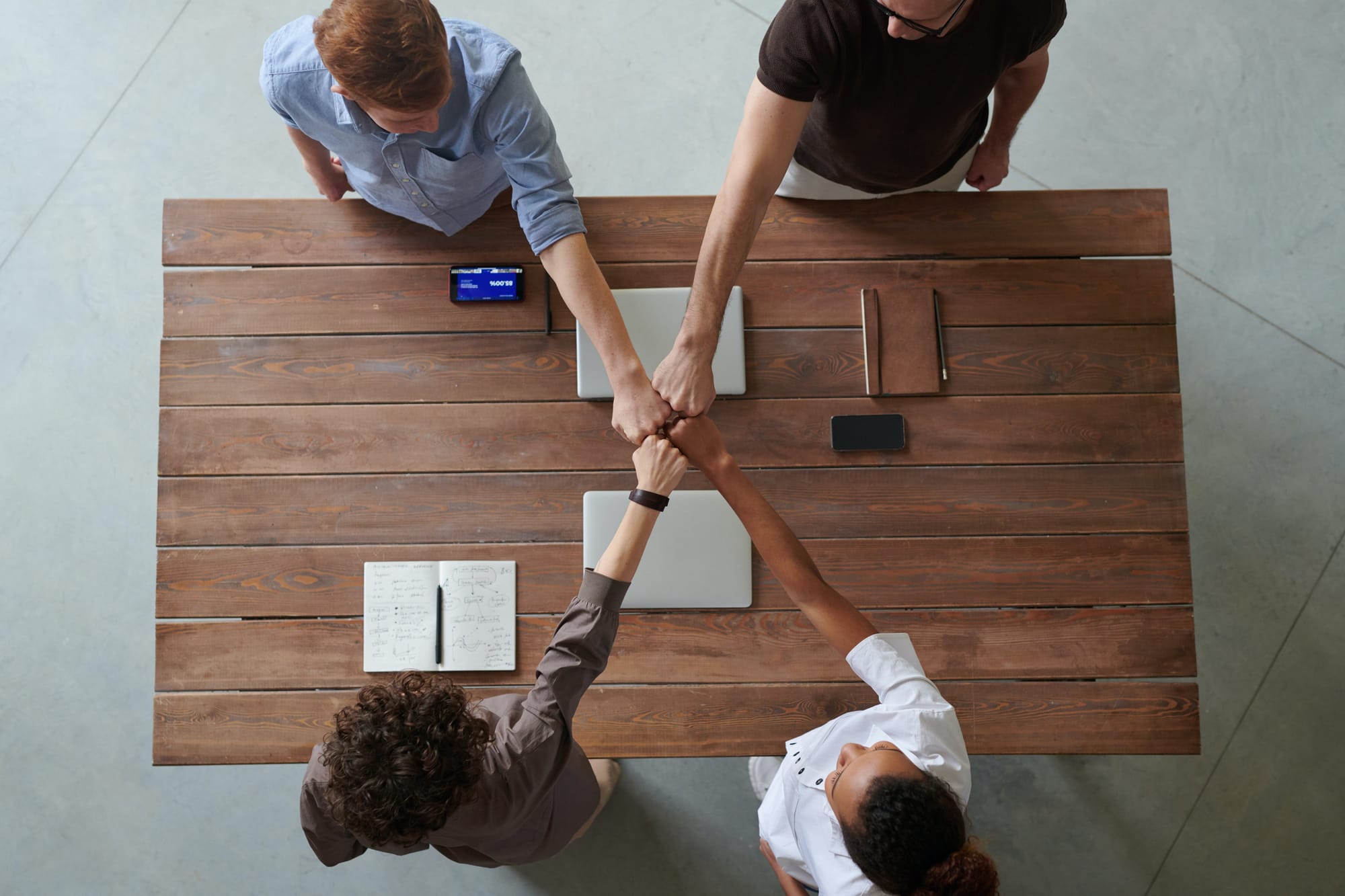
Consensus Metrics and Honeypots
Consensus metrics and honeypots are valuable tools in ensuring agreement among annotators and filtering out discrepancies. Consensus metrics measure the level of agreement between multiple annotators, providing insights into the reliability of the annotations. Honeypots, on the other hand, are known examples with pre-determined labels that allow annotators to showcase their competence. By comparing annotator responses to these known examples, organizations can identify and address any inconsistencies or inaccuracies in the annotation process.
Data Visualization for Annotation Consistency
Data visualization tools offer a powerful means to identify and address annotation inconsistencies. Visualizing the labeled data helps annotators and managers identify patterns, outliers, and potential errors. By visualizing the data, organizations can ensure consistency in annotation practices, enhancing the overall quality and accuracy of the labeled datasets.
Strategic Adjustments and Data Balancing
Identification of annotation anomalies through quality control measures allows for strategic adjustments to improve data quality. These adjustments may involve providing additional guidelines or training to the annotators, revising existing guidelines, or recruiting subject matter experts to provide clarifications. Balancing the dataset to ensure adequate representation of different classes or categories is also essential for data accuracy and improving the performance of machine learning models.
Continuous Quality Assurance Process
Establishing a continuous quality assurance process is vital for maintaining the effectiveness of the data annotation team and the overall success of the project. This process involves regular monitoring, feedback, and timely adjustments to ensure consistent data quality. By continually assessing and improving the annotation process, organizations can enhance the accuracy and reliability of the labeled data, leading to the development of robust machine learning models.
Benefits of Quality Assurance in Data Annotation |
---|
1. Ensures accuracy and reliability of labeled data |
2. Improves consistency and annotation quality |
3. Enhances the performance of machine learning models |
4. Supports strategic adjustments and data balancing |
5. Contributes to the development of reliable and trustworthy AI solutions |
Implementing quality assurance measures in data annotation projects is crucial for ensuring the accuracy and reliability of labeled data. Through the use of consensus metrics, honeypots, data visualization tools, strategic adjustments, and continuous quality assurance processes, organizations can enhance the effectiveness of their data annotation teams and contribute to the development of reliable machine learning models.
The Importance of Continuous Iteration in Data Annotation
Continuous iteration in data annotation is essential for optimizing model performance and reducing annotation costs. By consistently refining and fine-tuning the training dataset, businesses can achieve higher accuracy and realize significant cost savings.
One key aspect of continuous iteration is monitoring model outputs. By closely examining the performance of the model, data annotation teams can identify areas of underperformance and take strategic actions to improve the training dataset. This iterative process allows for targeted adjustments and refinements, ensuring that the model is constantly evolving to meet the desired performance levels.
Iterative improvements can involve different elements of the data annotation process. For example, adjusting the number of assets in the training dataset can help improve model performance by ensuring sufficient coverage of relevant data points. Modifying the percentage of consensus required among annotators can enhance the accuracy and consistency of the annotations.
Dataset composition is another critical aspect to consider during iteration. By incorporating new real-world parameters, businesses can improve the model's ability to handle diverse scenarios. This strategic adjustment allows the model to perform better in real-world applications, ensuring that it can handle various data inputs and produce reliable outputs.
Fine-tuning the training dataset is not only crucial for model performance but also contributes to cost savings. By eliminating redundant or unnecessary data points, businesses can reduce annotation efforts and streamline the data labeling process. This lean and agile approach to data annotation management optimizes resource allocation and reduces annotation costs, enabling businesses to allocate their resources effectively.
The Benefits of Continuous Iteration in Data Annotation:
- Optimizes model performance through targeted refinements and adjustments.
- Reduces annotation costs by eliminating redundant data and streamlining the labeling process.
- Enables adaptability to evolving business requirements and real-world parameters.
- Ensures the model's accuracy and relevance in real-world applications.
By adopting a data-centric approach to AI and prioritizing continuous iteration throughout the project lifecycle, businesses can maximize the effectiveness of their data annotation efforts. This iterative process not only improves model performance but also drives cost savings, ensuring that businesses stay agile and competitive in today's rapidly evolving data-driven landscape.
Benefits of Continuous Iteration in Data Annotation | Keywords |
---|---|
Optimization of model performance | model performance |
Reduction of annotation costs | cost savings, lean and agile management |
Adaptability to evolving business requirements | strategic adjustments |
Ensuring accuracy and relevance in real-world applications | model performance, monitoring model outputs |
Choosing the Right Data Annotation Platform
When it comes to selecting a data annotation platform for your project, several key factors need to be considered. These factors include data privacy, workforce access, integration capabilities, and the user experience (UX) and user interface (UI) design of the platform. Each of these elements plays a crucial role in ensuring the success of your data annotation endeavors.
Selecting the Right Level of Solution
One of the first choices you'll need to make is deciding between entry-level, advanced, or data-centric solutions. Entry-level solutions provide basic functionality and are suitable for smaller projects that do not require extensive scalability or advanced quality control features. However, they may have limitations when it comes to managing data quality.
Advanced solutions, on the other hand, offer a more comprehensive set of options that cater to the needs of larger projects. These platforms often provide features like automated quality control, customizable workflows, and enhanced data management capabilities. Despite their advancements, they may still have some limitations in terms of data quality management.
For those seeking a more holistic approach to data annotation, data-centric solutions are the ideal choice. These platforms prioritize data training and management, facilitating collaboration and iterative improvement. Additionally, data-centric solutions typically offer robust privacy features, seamless integration capabilities, and user-friendly interfaces to support efficient project management.
Consideration for Data Privacy and Integration Capabilities
When choosing a data annotation platform, it's essential to prioritize data privacy. Look for platforms that provide robust security measures to protect sensitive data. Additionally, ensure that the platform complies with relevant data privacy regulations, giving you peace of mind throughout the annotation process.
Integration capabilities are also crucial, especially if you plan to incorporate the platform into your existing workflows or data management systems. Seek platforms that offer seamless integration with popular tools and technologies, allowing for a smooth and efficient annotation process.
User Experience and Project Management Tools
The UX/UI interface of a data annotation platform can significantly impact the productivity and satisfaction of your annotation team. Look for platforms that provide an intuitive and user-friendly interface, minimizing the learning curve for annotators and maximizing their efficiency.
Additionally, project management tools are essential for organizing and monitoring the progress of your annotation projects. Features like task assignment, progress tracking, and collaborative annotations can greatly enhance the overall management of your data annotation team and improve the project's outcomes.
Choosing the Perfect Fit
Ultimately, the right data annotation platform depends on the specific requirements and goals of your project. Consider factors such as project size, data complexity, privacy needs, integration capabilities, and the level of data-centricity desired. By taking these aspects into account, you can select a platform that aligns perfectly with your needs, enhancing the efficiency and accuracy of your data annotation endeavors.
Conclusion
Successfully managing a data annotator team requires implementing data annotation best practices and adopting a data-centric AI approach. By following these strategies, businesses can enhance the efficiency and productivity of their data annotation projects, leading to the development of efficient machine learning solutions and value creation.
One of the key factors for project success is prioritizing data quality. Accurate and reliable data labeling is essential for training machine learning models that produce reliable results. Collaboration within the data annotator team and effective communication with the data science team help maintain consistency and improve the overall quality of the labeled data.
Continuous iteration is vital for ensuring the success of ML projects. Monitoring model outputs and making strategic adjustments to the training dataset based on real-world parameters help optimize model performance. Furthermore, adopting a lean and agile management approach promotes cost savings by eliminating redundant data and improving overall efficiency.
In conclusion, by implementing data annotation best practices, adopting a data-centric AI approach, and prioritizing data quality, collaboration, and continuous iteration, businesses can achieve project success and create valuable machine learning solutions with significant real-world impact.
FAQ
What is the role of a data annotator team manager?
The role of a data annotator team manager is to bridge the gap between the data science team and the annotation teams. They are responsible for fulfilling the data needs of the data science team, providing both training and retraining data for language models. Additionally, the manager ensures that annotators have sufficient data, understand the guidelines, and can use the labeling tool effectively. The manager also plays a crucial role in addressing annotators' questions and providing swift support to prevent any obstacles in the labeling process. Annotator feedback loops and flexible labeling interfaces help enhance the efficiency and performance of the team.
Why is building a data annotation team important?
Building a data annotation team is crucial for ensuring accurate labeling and categorization of data. This process allows for easier analysis and produces reliable results, which directly impact the quality of data models. By having a dedicated team, businesses can ensure that the data used in their models is accurate, up-to-date, and aligned with their specific use cases. The team's expertise and commitment to data accuracy significantly contribute to the overall success of data-driven decision-making.
How can the volume of training data for machine learning models be estimated?
The amount of training data required for machine learning models depends on the specific project and its complexity. However, there are estimation guidelines to help determine the necessary data volume. For smaller models, a general rule of thumb suggests using 10 times the data compared to the number of degrees of freedom in the model. Larger models require considering both the number of images and their size, accounting for parameters like color channels. These guidelines provide rough estimations to start with, but consulting with technical partners and data annotation team members is crucial for determining the optimal input data size.
How do data annotation teams help manage data drift in ML models?
Data drift occurs when the characteristics of the data used for training differ from the data the model is being used on. To ensure accurate predictions and maintain model performance, data annotation teams play a vital role in monitoring and addressing data drift. Regular testing of the model using real-world data helps detect and address any inaccuracies caused by data drift before the model is deployed to production. This ongoing monitoring and adjustment process is essential for maintaining model accuracy and relevance.
What are the benefits of having a dedicated data annotation team?
Having a dedicated data annotation team offers several advantages. First, the team ensures that annotation requirements are met adequately and within the set timeframe. Additionally, a team approach promotes consistent and accurate labeling across datasets. Subjectivity in data labeling is a common challenge, and the diversity and expertise of a team help address this issue. Team members can identify areas that require additional annotation or clarification, improving the overall quality and usability of the data for machine learning purposes.
What are the options for building a data annotation team?
There are multiple options for building a data annotation team, each with its pros and cons. In-house annotation involves hiring and training full-time annotators within the organization, offering consistency and management control but at a higher cost. Hiring freelancers provides cost-effective flexibility, but quality control and consistency may be challenging. Fully outsourcing the annotation task to professional services offers turnkey solutions and expertise but comes with a higher price tag and potential limitations in tool integration. Choosing the most suitable option depends on project goals, budget, requirements, and complexity.
How can an effective data annotation process be established?
A well-defined data annotation process is crucial for achieving consistent and accurate results. Clear instructions and labeling conventions should be established to guide the workforce, ensuring consistency and adherence to quality standards. The process should be thoroughly documented and regularly updated to facilitate easy access and keep up with evolving protocols. The process should also prioritize workforce productivity by addressing their questions and providing timely feedback. Proper documentation and guideline accessibility promote a collaborative and supportive working environment.
How can quality assurance be implemented in data annotation?
Quality assurance is essential in data annotation projects. Consensus metrics and honeypots can be used to ensure agreement among annotators and filter out discrepancies. Data visualization tools help identify and address annotation inconsistencies, unbalanced datasets, and other quality issues. Strategic adjustments based on the identified anomalies improve the overall quality and accuracy of the labeled data. Establishing a continuous quality assurance process enhances the effectiveness of the data annotation team and contributes to the development of reliable machine learning models.
Why is continuous iteration important in data annotation?
Continuous iteration in data annotation is essential for optimizing model performance and reducing annotation costs. Monitoring model outputs helps identify areas of underperformance, enabling strategic adjustments to the training dataset. Iteration can involve adjusting the number of assets, the percentage of consensus, dataset composition, guidelines, or incorporating new real-world parameters. Fine-tuning the training dataset not only improves model performance but also enables cost savings by eliminating redundant data. A data-centric approach to AI prioritizes continuous iteration and improvement throughout the project lifecycle.
What should be considered when choosing a data annotation platform?
When selecting a data annotation platform, it is crucial to consider data privacy, workforce access, and integration capabilities. Entry-level solutions provide basic functionality but lack scalability and quality control features. Advanced solutions offer more comprehensive options, but may still have limitations in data quality management. Data-centric solutions prioritize data training and management, facilitating collaboration, and iterative improvement. These platforms typically offer data privacy, integration capabilities, and user-friendly interfaces to support efficient project management. Choosing the right platform depends on the specific project needs and the desired level of data-centricity.
How can a data annotator team be effectively managed?
Successfully managing a data annotator team requires implementing best practices and adopting a data-centric AI approach. By following the strategies outlined in this article, businesses can enhance the efficiency and productivity of their data annotation projects, leading to the development of effective machine learning solutions and value creation. Prioritizing data quality, collaboration, and continuous iteration is key to the success of ML projects and achieving tangible results in real-world applications.
Comments ()