How Video Annotation Services Train Deep Learning Systems
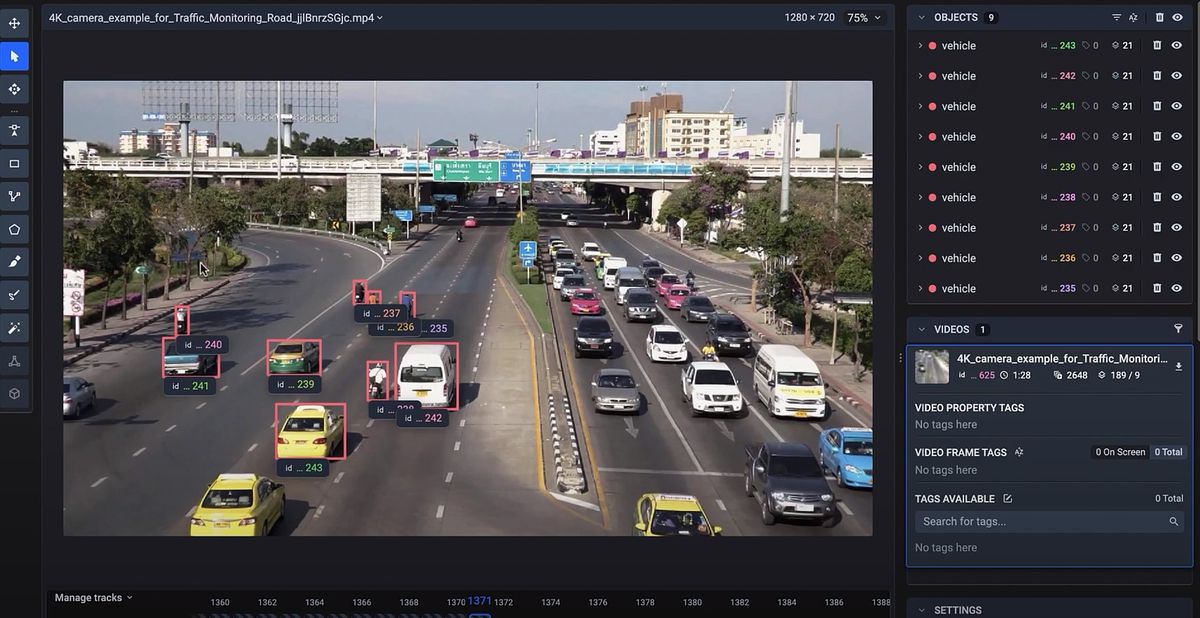
Video annotation involves labeling and categorizing content for training machine learning algorithms. Video annotation services annotate objects, actions, and events depicted in a video. They can provide a deep learning system with the data it needs to learn and make informed decisions.
This article explores the various types of video annotation tasks. You'll also learn about the process of creating a video annotation project. Finally, you'll learn the importance of quality control in achieving accurate results.
Types of Video Annotation Tasks
Several types of video annotation tasks are common in training deep learning systems. Some of the most common types include:
- Object detection – In object detection, annotators identify and label objects in a video. For example, an annotator can label all instances of a person, car, or particular animal in a video.
- Semantic segmentation – Annotators label pixels with classes in semantic segmentation tasks. For example, an annotator can label the pixels corresponding to the sky as "sky," "grass," and so on.
- Tracking – In tracking, annotators follow the movement of an object throughout a video. For example, an annotator might track the person's face as they speak. Or they can track the movement of a vehicle as it travels through a scene.
These are a few examples of video annotation tasks in training deep learning systems. You may also need other annotations depending on a project's specific goals.
The Process of Creating a Video Annotation Project
Creating a video annotation project involves several key steps, including
- Selecting a video annotation tool – Different tools are available for video annotation projects. Some tools are for specific annotation tasks, while others are more general-purpose. Choosing a tool that is well-suited to your particular needs and the goals of your project is crucial.
- Creating a project plan – Develop a detailed plan before your video annotation project. Outline the project's goals, the types of annotation tasks, and the timeline. This plan should also include details about the necessary resources. For example, include the number of annotators required.
- Recruiting and training annotators – Once you have a project plan, you need a team. Choosing annotators who are detail-oriented and able to follow instructions is essential. You may also need to provide training. This step ensures annotators understand your project's specific requirements.
- Managing the annotation process – You must manage the work as the project progresses. You'll also need to ensure that they meet the deadlines and produce high-quality work. Provide feedback and guidance to your annotators. Track their progress and make adjustments as needed.
Following these steps and paying careful attention to your project details ensures success. You'll also get the results you need to train a high-quality deep learning system.
Quality Control in Video Annotation Projects
Ensuring the quality of the data produced in a video annotation project is crucial. It's the only way to ensure the success of the deep learning system. There are several critical considerations for quality control in video annotation projects.
All annotators in a project must follow the same guidelines and standards. As a result, the data produced is consistent and can train a deep learning system. The data produced in a video annotation project must be accurate. Otherwise, it cannot train a deep learning system. Annotators must pay close attention to detail and follow the instructions.
Reviewing the work of annotators and providing feedback can help. Checks ensure that the quality of the data remains high throughout the project. You can check a random sample of annotation tasks. Then, provide annotators with examples of good and bad work. Finally, provide guidance and support as needed.
Besides regular review and feedback, it may help to have formal quality assurance. More processes ensure that the video annotation project is high quality. You could also include a separate team of reviewers. They can also install automated quality checks using software tools.
How to Choose the Best Video Annotation Services
Look for video annotation services with a track record of success. Also, look for services with experience in your specific industry or application area. You want annotators with the knowledge and experience to create high-quality annotated data. Look for providers with established quality control processes.
You may have specific turnaround time requirements. Be sure to choose a provider to meet your deadlines and deliver the annotated data you need on time. These tips can help you choose the best video annotation service for your project.
Conclusion
Video annotation services are crucial in developing deep learning systems. By labeling video content, annotators develop deep learning systems with novel capabilities. Quality control is an important consideration. The accuracy and consistency of the data are essential to get the results you're looking for.
Comments ()