How to Hire the Best Data Annotators for Your AI Project
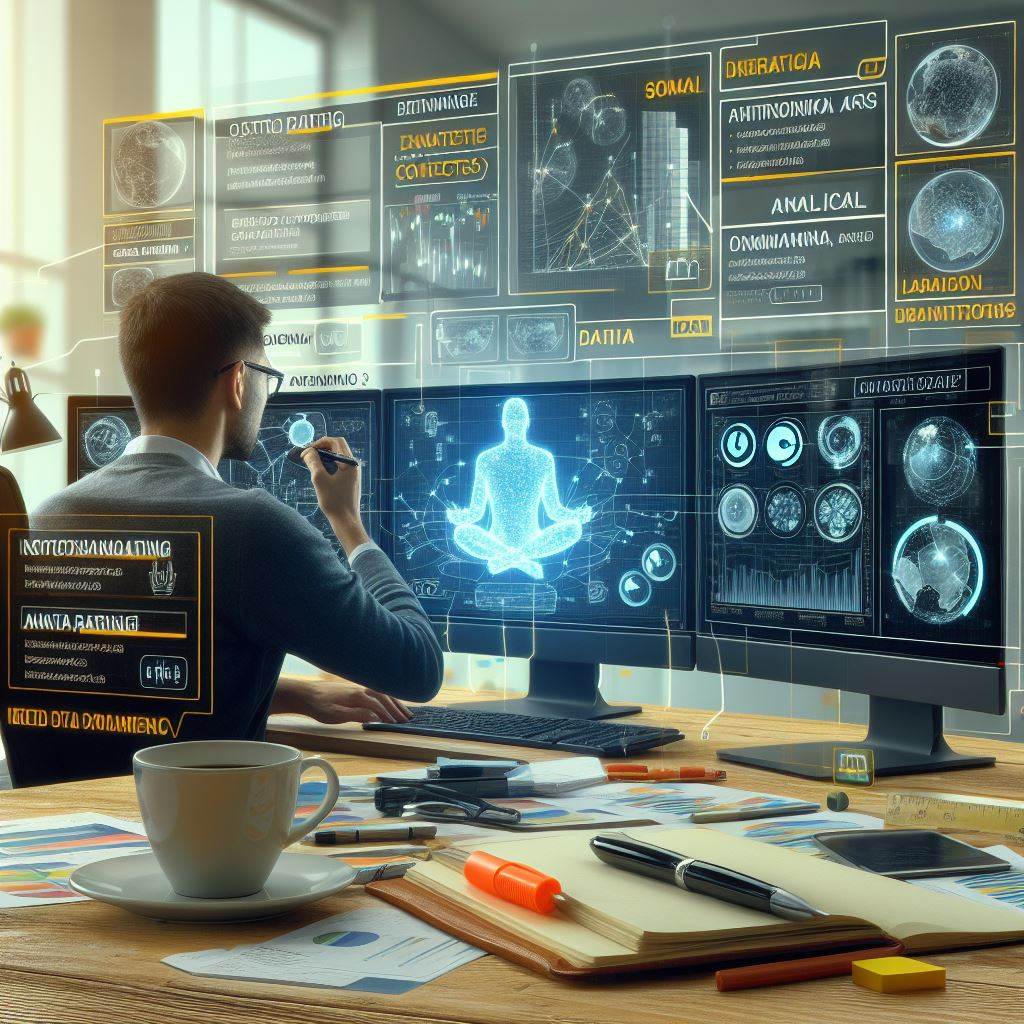
Data annotation is a crucial process for improving machine learning models. Hiring skilled data annotators is essential to ensure the accuracy and quality of the annotated data. Whether you are looking for data annotation services, data annotation companies, data labeling services, or data labeling companies, finding the right professionals can make all the difference in the success of your AI project.
Key Takeaways:
- Skilled data annotators are essential for improving machine learning models.
- Choose between in-house teams, freelancers, or outsourcing professionals from third-party companies.
- Consider the challenges of data annotation, such as accurate classification and time constraints.
- Explore different hiring options like job boards, social media, and referrals to find the best data annotators.
- Evaluate data annotator rates in different countries and balance cost-efficiency with expertise and quality of work.
What Does a Data Annotation Specialist Do?
A data annotation specialist plays a vital role in the annotation and labeling of large volumes of data. Working as part of a team, they meticulously tag and label data, ensuring its accuracy and relevance for training machine learning models. Beyond annotation, data annotation specialists are responsible for validating the annotated data to guarantee its suitability for training machines effectively. They possess the expertise to analyze, tag, and label data accurately, utilizing their knowledge in data annotation to produce high-quality training datasets.
Data annotation specialists are crucial for ensuring the integrity and usefulness of labeled data, which ultimately drives the success of AI projects and machine learning models. Hiring someone who excels in data annotation and can be trusted with sensitive company data is of paramount importance.
“The ability to accurately analyze, tag, and label data is a key skill that a data annotation specialist must possess, ensuring the high quality and usability of training datasets.”
By leveraging their expertise in data annotation, these specialists contribute to the development of robust AI systems that power various applications, including image recognition, natural language processing, and sentiment analysis.
Data Labeling and Tagging
One of the core responsibilities of a data annotation specialist is data labeling and tagging. This involves accurately assigning meaningful labels and tags to different types of data, such as text, images, and videos. This meticulous process enables machines to recognize patterns, classify objects, and make accurate predictions based on the labeled data.
Data labeling involves assigning relevant labels to data, such as categorizing images into different classes or assigning sentiment scores to text. On the other hand, data tagging involves marking specific attributes or characteristics within the data, providing more granular information for training machine learning models.
Overall, data labeling and tagging are essential tasks that ensure the availability of well-annotated and structured datasets, enabling efficient model training and accurate predictions.
Validating Annotated Data
Data annotation specialists are also responsible for validating annotated data. This validation process ensures the correctness, consistency, and quality of the labeled data. By meticulously reviewing and verifying the annotated data, specialists identify and correct any errors or inconsistencies that may impact the performance of machine learning models.
Validating annotated data is a critical step in the data annotation process as it eliminates potential bias, inaccuracies, and inconsistencies that could adversely affect the AI project's outcomes. Through rigorous validation, data annotation specialists enhance the reliability and accuracy of the training datasets, enabling more robust machine learning models.
Key Responsibilities of a Data Annotation Specialist
Here are some key responsibilities that data annotation specialists typically undertake:
- Analyzing data to identify key features and attributes.
- Developing annotation guidelines and standards.
- Accurately assigning labels and tags to data.
- Validating annotated data for quality assurance.
- Collaborating with cross-functional teams for annotation projects.
- Maintaining data security and confidentiality.
Responsibility | Description |
---|---|
Analyzing data | Identifying key features and attributes within the data to guide the annotation process. |
Developing annotation guidelines and standards | Creating clear and consistent guidelines for annotators to ensure the accuracy and consistency of annotations. |
Accurately assigning labels and tags | Applying precise labels and tags to data, ensuring its meaningful representation for machine learning models. |
Validating annotated data | Reviewing and verifying the accuracy and quality of annotated data to eliminate errors and inconsistencies. |
Collaborating with cross-functional teams | Working closely with other team members, such as data scientists and engineers, to ensure the smooth execution of annotation projects. |
Maintaining data security and confidentiality | Adhering to strict data security protocols and ensuring the confidentiality of sensitive company information during the annotation process. |
In-House Data Annotators
When it comes to data annotation for your AI project, one option is to build an in-house team of data annotators. This means hiring individuals who will work exclusively for your company, ensuring a higher level of data security. In-house teams can also better understand the specific needs of your project, as they are directly integrated into your organization.
However, building an in-house team does come with its challenges. Finding individuals with the specialized skills required for data annotation can be a time-consuming process. It may require significant resources and effort to recruit and train the right talent for your team. Additionally, ensuring data security is of utmost importance, so it's crucial to have a dedicated HR team in place to handle security protocols and ensure employees sign non-disclosure agreements.
In spite of these challenges, having an in-house team offers several advantages. With easy access to team members, collaboration and communication can be streamlined, leading to increased efficiency. Furthermore, your team will become intimately familiar with your project's objectives, requirements, and unique data challenges, allowing them to deliver more accurate and tailored annotations.
Consider the benefits and trade-offs of building an in-house team before making a decision. Assess the availability of the necessary resources and ensure that you can provide ongoing support and growth opportunities for your data annotators. Overall, an in-house team can provide a dedicated and secure solution to your data annotation needs, ensuring the quality and integrity of your training data.
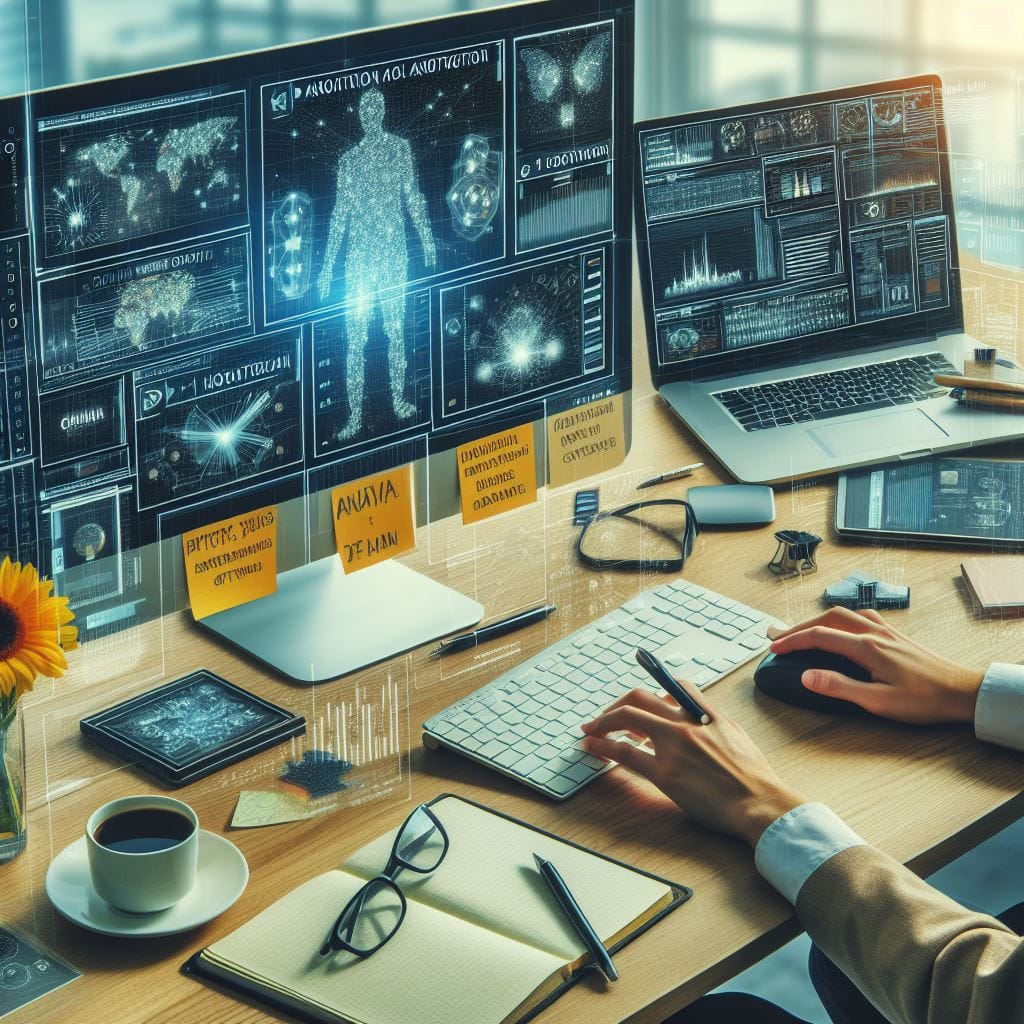
Advantages of an In-House Team:
- Higher data security through non-disclosure agreements
- Better understanding of project needs
- Easier collaboration and communication
- Opportunity for tailored and accurate annotation
Disadvantages of an In-House Team:
- Challenges in finding individuals with specialized skills
- Resource and time-intensive hiring and training process
- Requirement of a dedicated HR team for security measures
In-House Data Annotation Team vs. Outsourced Solutions
While an in-house data annotation team offers many advantages, it's essential to consider other options such as outsourcing or hiring freelancers. Outsourcing data annotation to specialized third-party companies is an alternative that provides access to a pool of skilled annotators without the need for extensive recruiting efforts. The decision will ultimately depend on your project requirements, timeline, budget, and the level of control you desire.
The image below illustrates the pros and cons of an in-house team and outsourced solutions:
In-House Data Annotators | Outsourced Solutions | |
---|---|---|
Advantages | Higher data security through non-disclosure agreements Better understanding of project needs Easier collaboration and communication Opportunity for tailored and accurate annotation | Access to a pool of skilled annotators Faster ramp-up time Ability to scale annotation resources Lower upfront investment |
Disadvantages | Challenges in finding individuals with specialized skills Resource and time-intensive hiring and training process Requirement of a dedicated HR team for security measures | Potential differences in opinion and communication issues Possible compromise on data security Less control over annotation process |
Hiring Freelancers
When it comes to data annotation projects, some companies prefer to hire freelancers on a per-project basis. This option can be cost-effective compared to maintaining an in-house team. Companies can find freelancers with specific skills and expertise for their projects, allowing for flexibility and scalability.
However, it's important to consider the potential drawbacks of hiring freelancers. One concern is ensuring the quality of work. Freelancers may not have the same level of accountability as an in-house team, so it's crucial to thoroughly vet their qualifications and verify their track record of delivering high-quality annotations.
Data security is another aspect to consider. When entrusting sensitive data to freelancers, it's essential to establish data security protocols and ensure that freelancers adhere to them. This can include signing non-disclosure agreements and providing a secure infrastructure for data handling and storage.
While hiring freelancers can provide cost-effective solutions for data annotation projects, it's important to carefully evaluate their skills and trustworthiness. Thorough vetting, clear communication, and strict security measures can help ensure a successful collaboration with freelancers.
"Hiring freelancers for data annotation projects can be a cost-effective option, but it's crucial to thoroughly vet their qualifications and establish data security protocols."
Advantages of Hiring Freelancers
- Cost-effective compared to maintaining an in-house team
- Flexibility and scalability
- Access to specialized skills and expertise
Disadvantages of Hiring Freelancers
- Potential concerns about the quality of work
- Data security risks
- Less accountability compared to an in-house team
Considerations when Hiring Freelancers
- Thoroughly vet freelancers and verify their qualifications
- Establish clear communication channels
- Implement data security protocols and ensure freelancers adhere to them
- Monitor and evaluate the quality of work consistently
When hiring freelancers, it's essential to strike a balance between cost-effectiveness and ensuring the highest quality of work and data security. By carefully selecting and managing freelancers, companies can leverage their expertise while maintaining control over the annotation process.
Advantages | Disadvantages |
---|---|
Cost-effective | Potential concerns about the quality of work |
Flexibility and scalability | Data security risks |
Access to specialized skills and expertise | Less accountability compared to an in-house team |
Outsourcing Professionals from Third-Party Companies
Outsourcing data annotation professionals from third-party companies is another viable option for businesses seeking specialized expertise. These professionals are employed by companies that focus solely on data annotation and can be hired on a project-by-project basis. Outsourcing professionals from third-party companies offers several advantages.
Firstly, these professionals are industry experts with in-depth knowledge and experience in data annotation. They are well-versed in the latest tools and techniques, ensuring high-quality annotations that align with project requirements. By leveraging the expertise of these professionals, businesses can benefit from their specialized skills and ensure accurate and reliable annotations.
Secondly, third-party companies provide a structured and reliable infrastructure for managing data annotation projects. They have established processes and workflows in place, streamlining the entire annotation process. This allows businesses to efficiently scale their annotation projects and meet their project deadlines.
However, it's essential to consider potential challenges when outsourcing to third-party companies. Differences in opinions and communication between the business and the third-party professionals may arise, potentially impacting the timeline of the project. Effective communication and clear project requirements are crucial to ensuring a successful outsourcing arrangement.
In summary, outsourcing data annotation professionals from third-party companies offers businesses access to specialized expertise and a reliable infrastructure. However, effective communication and clear project requirements are vital to overcome potential differences in opinions and ensure successful collaboration.
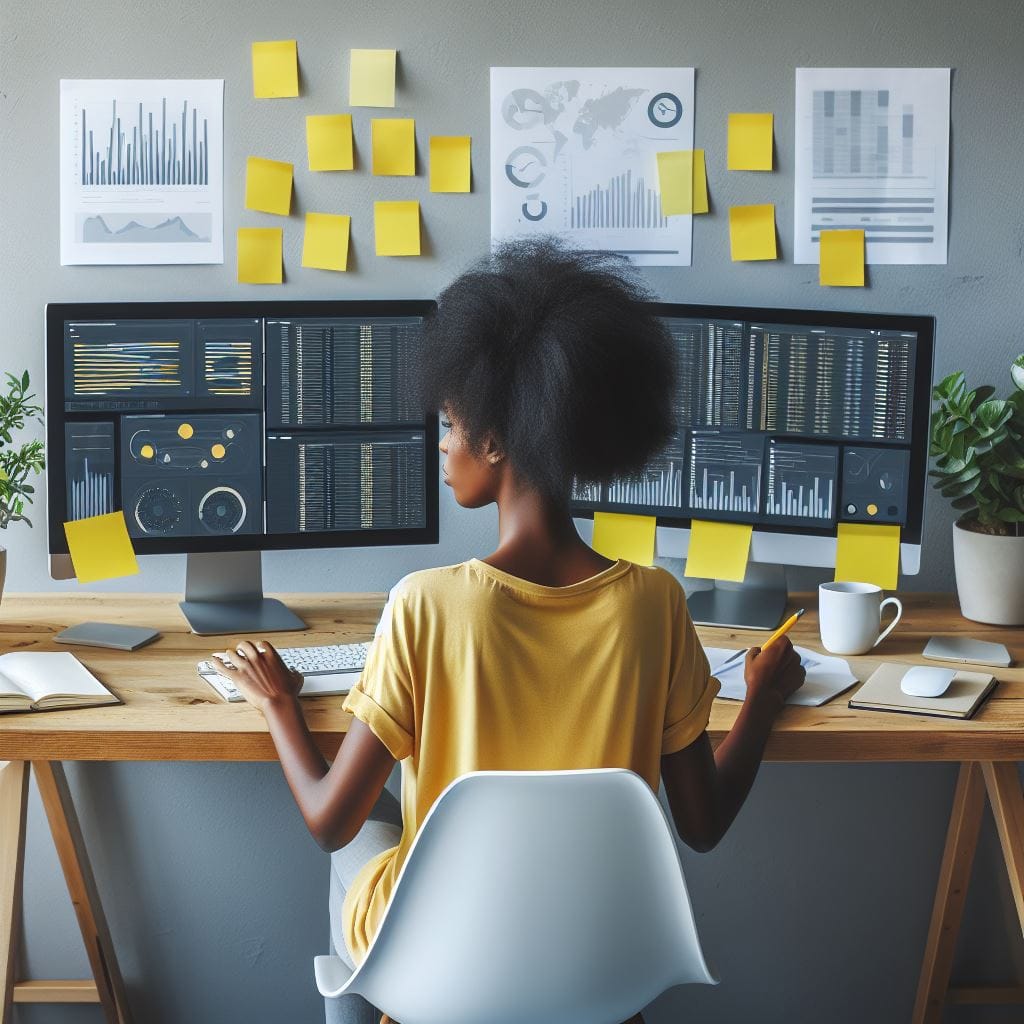
Other Things to Remember About Data Annotation
Data annotation presents several challenges that need to be considered for successful implementation. These challenges include accurate data classification, document labeling, time constraints, data security guidelines, and ensuring quality work. Let's explore each of these challenges in detail:
Data Classification
Accurate data classification is crucial for effective data annotation. It involves categorizing data based on predefined criteria to ensure that machine learning models can learn and make accurate predictions. Data classification can be complex, especially when dealing with large datasets or ambiguous data points.
Document Labeling
Document labeling is an essential part of data annotation, particularly in fields such as natural language processing. It involves assigning descriptive tags or labels to textual documents, making it easier for machines to understand and process the information. Document labeling requires linguistic expertise and domain knowledge to ensure precise and consistent labeling.
Time Constraints
Time constraints can pose challenges in data annotation projects. Annotations need to be completed within a given timeframe to meet project deadlines. Effective project management and allocation of resources are essential to ensure efficient annotation processes under time constraints.
Data Security Guidelines
Data security is vital when handling sensitive information during the annotation process. Strict guidelines and protocols should be followed to maintain data confidentiality and protect against unauthorized access or data breaches. Compliance with data protection regulations, such as GDPR, is crucial in ensuring data security.
Quality Work
Ensuring quality work is essential for reliable training data annotation. Data annotation specialists must adhere to annotation guidelines and maintain consistency in their labeling approach. Quality assurance techniques, such as regular quality checks and inter-annotator agreement evaluations, help ensure the accuracy and reliability of annotated data.
"Data classification, document labeling, time constraints, data security guidelines, and providing quality work are significant challenges that organizations face in data annotation projects. Overcoming these challenges requires careful planning, skilled resources, and robust processes to ensure accurate annotations and dependable training data."
Addressing these challenges is crucial for successful data annotation projects. Being aware of the potential difficulties and having contingency plans in place will help mitigate risks and ensure the delivery of high-quality annotated data for training machine learning models.
Best Places to Hire Data Annotators
When it comes to hiring data annotators for your AI project, there are several options you can explore to find the best talent. Below are some of the best places to consider:
- Job Boards: Posting job openings on popular job boards can help you attract potential candidates with experience in data annotation. These platforms, such as Indeed, LinkedIn, and Glassdoor, are frequented by professionals actively looking for data annotation opportunities. You can also use advanced search filters to target candidates with specific skills or experience.
- Social Media: Utilize social media platforms like Facebook, Twitter, and LinkedIn to promote your data annotator job openings. Engage with relevant communities and groups dedicated to AI, data science, and machine learning. By leveraging the power of social media, you can reach a wider audience and tap into networks of professionals who may be interested in data annotation roles.
- SEO-Friendly Ads: Create targeted and SEO-friendly advertisements to attract data annotators. Optimize your ads with keywords and phrases relevant to the role, such as "data annotation specialist," "data labeling expert," or "AI project annotator." By running ads on search engines and relevant websites, you can increase visibility and draw the attention of qualified data annotators.
- Third-Party Companies: Consider hiring data annotators from third-party companies that specialize in providing data annotation services. These companies have already screened and trained data annotators, ensuring you have access to a pool of skilled professionals. By outsourcing your data annotation needs to these companies, you can save time and resources on recruitment and training.
- Referrals: Tap into your existing network and encourage your employees to refer qualified data annotators. Employee referrals can be a valuable source of talent, as your employees may have connections with individuals experienced in data annotation. Implement a referral program that incentivizes employees to refer suitable candidates and ensure a smooth hiring process for referred candidates.
- Hiring from Specific Countries: Consider hiring data annotators from specific countries based on cost-efficiency and expertise. For example, countries like India, Poland, Ukraine, and the United Kingdom have a pool of skilled data annotators. By hiring from these countries, you can take advantage of cost-effective labor while ensuring quality work.
While cost is an important consideration, prioritize quality when hiring data annotators. Assess their skills, experience, and portfolios to ensure they can meet your project's requirements. Remember, hiring data annotators is a critical step in obtaining accurate and high-quality training data for your AI project.
Factors to Consider When Hiring Data Annotators
When deciding where to hire data annotators, it is important to evaluate the cost-efficiency of different countries, but also consider other factors such as the annotators' expertise and the quality of their work. While cost is an important consideration, it should not be the sole criteria for hiring annotators. Annotators from countries with lower rates may have the necessary skills and experience, but it is important to thoroughly vet them and ensure that they can deliver the required level of quality and accuracy.
Conclusion
Hiring data annotators is an essential step in ensuring the success of your AI project. These specialists play a vital role in providing high-quality training data annotation, which is crucial for improving machine learning models. Whether you decide to build an in-house team, hire freelancers, or outsource to third-party companies, it is important to thoroughly evaluate their expertise, reliability, and security measures.
The accuracy and effectiveness of your AI project rely heavily on finding the right data annotators. Skilled professionals will ensure that your training data is properly labeled and annotated, enabling your machine learning models to learn and make accurate predictions. Their expertise in data annotation will contribute to the high quality of the annotated data, ultimately leading to the improved performance of your AI algorithms.
When hiring data annotators, consider their experience, knowledge, and ability to handle sensitive company data. Assess their track record and determine if they have a good understanding of your project's requirements. By prioritizing quality training data annotation and choosing the right specialists, you will set your AI project up for success and achieve better results in the long run.
FAQ
How can I hire the best data annotators for my AI project?
To hire the best data annotators, you can explore different options such as job boards, social media platforms, and SEO-friendly ads. You can also hire from third-party companies that have already screened and trained data annotators. Referrals from employees can also be a valuable source for finding talented annotators. Consider hiring annotators from specific countries based on cost-efficiency, but remember to prioritize quality over cost alone.
What does a data annotation specialist do?
A data annotation specialist is responsible for tagging and labeling different types of data, such as text, images, and videos. They work in a team to label and tag large volumes of data and are also responsible for validating the annotated data to ensure its suitability for training machines. Data annotation specialists need to have the ability to accurately analyze, tag, and label data.
What are the options for hiring data annotators?
There are three main options for hiring data annotators: building an in-house team, hiring freelancers, and outsourcing professionals from third-party companies.
What are the benefits of building an in-house team of data annotators?
Hiring an in-house team of data annotators provides a higher level of security for your data, as employees are required to sign non-disclosure agreements. In-house teams can also better understand the needs of your project. However, building an in-house team may require time and resources, and it can be challenging to find individuals with the specialized skills required for data annotation.
What are the considerations when hiring freelancers for data annotation projects?
Hiring freelancers can be cost-effective compared to building an in-house team. However, the quality of work and data security can be a concern. It is important to thoroughly vet freelancers and ensure they have the necessary skills and can be trusted with sensitive data.
What are the advantages of outsourcing data annotation professionals from third-party companies?
Outsourcing professionals can be beneficial because they have expertise in their field and follow their company's infrastructure. However, there may be differences in opinions and communication that could affect the timeline of the project.
What are the challenges of data annotation?
Ensuring accurate data classification and document labeling can be a complex task. Time constraints and data security guidelines may also pose challenges, especially when outsourcing. Additionally, there is no guarantee of quality work, especially when working with a team for the first time. It is important to be prepared for these challenges and have contingency plans in place.
Where can I find the best data annotators?
The best places to hire data annotators include job boards, social media platforms, and SEO-friendly ads. You can also consider hiring from third-party companies that have already screened and trained data annotators. Referrals from employees can also be a valuable source for finding talented annotators.
What are the rates for data annotators in different countries?
The rates for data annotators vary depending on the country. In the United States of America, the average annual rate is $39,000. In India, the rate is $20,467, while in Poland, it is $46,545. Ukraine has an average rate of $32,000, and the United Kingdom has an average rate of $29,196.
Why is hiring the best data annotators crucial for an AI project?
Hiring the best data annotators is crucial because data annotation specialists play a vital role in providing high-quality training data annotation, which is essential for improving machine learning models. By finding the right data annotators, you can ensure the accuracy and effectiveness of your AI project.
Comments ()