AI In Diagnosis: How AI Is Transforming Medical Diagnosis
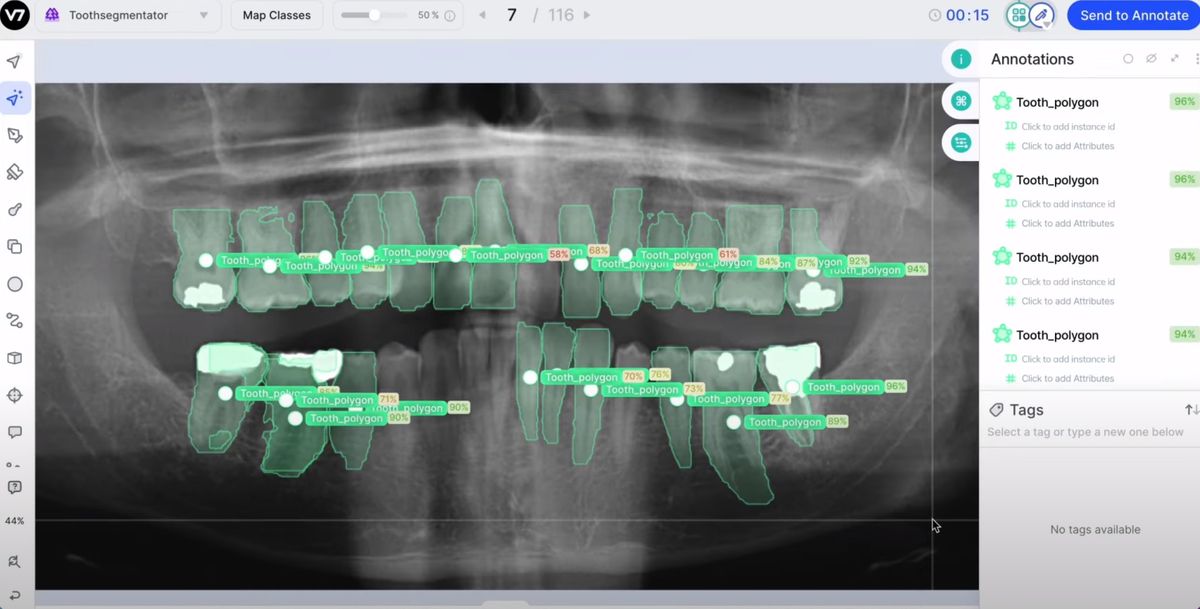
Artificial intelligence (AI) is rapidly transforming the field of medicine, from diagnosis to drug discovery and personalized treatment plans. As AI continues to advance, its potential to revolutionize healthcare is becoming more evident. In this article, we will explore the top three use cases of AI in medicine that are making a significant impact on patient care.
First, we will delve into how AI is transforming medical diagnosis, providing faster and more accurate diagnoses for patients. Then, we will discuss the role of AI in drug discovery, which is accelerating the development of new treatments and therapies. Finally, we will explore how AI is revolutionizing personalized medicine, tailoring treatments to individual patients based on their unique characteristics.
We will also examine the benefits and challenges of AI in medical imaging analysis, which is becoming increasingly important in the diagnosis and treatment of various medical conditions. Join us as we explore the exciting ways in which AI is changing the face of medicine and improving patient outcomes.
Medical diagnosis is an area where AI is increasingly being used to provide patients with more accurate, faster diagnoses. One of the main ways AI is transforming medical diagnostics is through medical imaging analysis. Modern AI platforms are capable of detecting breast cancer, analyzing cardiac MRI images, reading X-rays in emergency departments, tracking lung nodules, diagnosing brain tumors, and identifying strokes.
Machines excel in advanced pattern recognition on a wider variety of anomalies than most human healthcare professionals can recognize. By using advanced data processing techniques and machine learning algorithms that analyze large amounts of patient data points they are able to generate personalized patient care plans at unmatched speeds.
In addition to supporting medical imaging analysis and case triage, AI can also automate assessments and tasks that humans currently do but take a lot of time. This leads to faster and more accurate diagnoses while enabling healthcare practitioners to concentrate on the next steps in treatment planning rather than tiresome repetitive tasks such as catalogue labs or update EMRs (Electronic Medical Records).
At present time, machines with artificial intelligence capabilities have achieved considerable success in enhancing radiology task accuracy thus reducing errors during diagnosis by recognizing patterns in images or accurately measuring sizes for comparison purposes.
As technology continues to evolve across all industries including healthcare it will be interesting to see how chatbots will be assisting front-line staff when acquiring patients' symptoms history...
AI In Drug Discovery: The Role Of AI In Accelerating Drug Discovery
Artificial Intelligence (AI) has transformed drug discovery and is a vital tool in multiple scientific disciplines. The incorporation of AI in pharmaceutical research has allowed scientists to accelerate the process of drug development, improve diagnosis, and enhance treatment outcomes. AI-based applications offer several use cases relevant to drug discovery, including target identification, small molecule library screening, de novo drug design, drug repurposing, and preclinical studies.
The integration of AI can expedite the identification of new therapeutic targets and lead molecules for a given disease by analyzing large volumes of data that would be impossible for humans to review in a timely manner. By reducing the time-consuming process required for traditional experimental approaches like high-throughput screening, it increases the efficiency and speed at which researchers identify targets with higher accuracy. Additionally, machine learning algorithms are improving data analysis by identifying novel chemical compounds that are structurally similar to known active molecules.
AI helps reduce costs by enabling early-stage predictions about potential risks or benefits long before any investment decisions are made using computer simulations rather than traditional trial-and-error methods. Furthermore, pharmacological prediction models also help shorten preclinical trials as well as clinical trial phases 1-3 since decision-making becomes more accurate due to better dose-response assessments initiatives.
To maximize its full potential requires access to extensive amounts of high-quality data models from various fields involved in medicine such as biophysics or biochemistry. Therefore better collaborations between Machine Learning tailored teams and domain-specific experts remain essential for achieving significant results using ML tools ultimately aimed at discovering effective medical treatments.
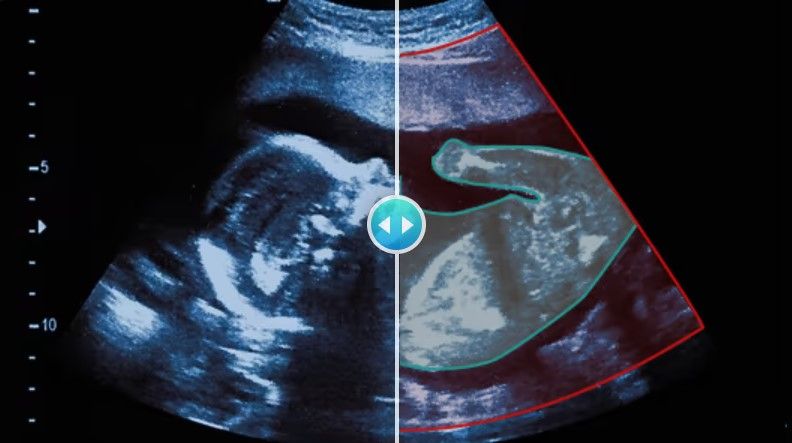
AI In Personalized Medicine: How AI Is Revolutionizing Personalized Medicine
AI is revolutionizing personalized medicine by providing doctors with the tools to develop personalized treatments for each patient. IBM Watson Health has partnered with Brigham and Women's Hospital and Vanderbilt University Medical Center to use AI on electronic health records and claims data. With this technology, doctors can analyze patient data and suggest personalized treatment options based on each patient's genetic history, genomic sequencing, medical records, location, environmental factors, and more.
AI techniques have proven useful for efficient and high-throughput genome interpretation. High-throughput, data-intensive biomedical research assays and technologies have created a need for researchers to develop strategies for analyzing, integrating, and interpreting the massive amounts of data they generate with AI. This allows scientists to better understand how individual genes function in disease prevention while also opening doors to previously unrealized diagnoses.
In addition to improving treatment options through genomic analysis, AI is revolutionizing medical imaging and diagnostics in healthcare. With machine learning algorithms that can recognize patterns within large sets of images taken across the world from various hospital systems or imaging devices improve disease detection accuracy which leads towards devising effective cures.
AI plays a key role in drug discovery as well. In pharma research laboratories worldwide researchers are speeding up drug discovery efforts by creating intelligently designed lead compounds using deep learning algorithms & genomics information instead of relying solely on trial-and-error methods commonly used today dictated by traditional hypothesis-driven scientific methods it often takes years in total time due to manual experimentation on lab animals or humans such as clinical trials thereby cost savings will be high predicted by experts from Academia & Industry alike. Overall The use if AI applied could change radically evolve development workflows allowing cheaper easier access wider usage possibilities for patients all around the globe since building virtual construction modeled studies that cannot exist outside of computational resources enables far greater scale than physical models ever could.
These developments show how AI can improve patient care not only through better diagnosis but also in delivering more effective and customized treatments based on their specific needs. It is evident that AI has the potential to transform healthcare by enabling more efficient and personalized treatment options resulting in better outcomes for patients.
AI In Medical Imaging: The Benefits And Challenges Of AI In Medical Imaging Analysis
AI has the potential to revolutionize medical imaging analysis, improving diagnostic accuracy and efficiency while reducing costs. Medical imaging generates massive amounts of data that can be difficult for even the most skilled radiologists to handle efficiently. AI algorithms, however, can quickly analyze this data and identify abnormalities with high accuracy.
One of the main benefits of AI in medical imaging is case triage. Instead of having radiologists manually examine all images generated from medical scans, AI algorithms can prioritize cases based on urgency or likelihood of detecting anomalies. This not only saves time but also enables healthcare providers to deliver faster and more accurate diagnoses.
Implementing AI in medical imaging does come with challenges, including cost analysis and digitizing health services. However, deploying AI systems can lead to significant cost savings over time as well as improved clinical outcomes for patients. Furthermore, the use of deep learning techniques allows for better population health management by identifying risk factors early on and enabling targeted interventions.
Incorporating AI into medical imaging has enormous benefits that outweigh any potential challenges that come with implementing such technologies. With its ability to significantly improve diagnostic accuracy and efficiency while reducing costs and enhancing patient care delivery via reduced diagnostic turnaround times- it holds great promise for improving overall health outcomes across populations worldwide.
AI In Clinical Trials: The Impact Of AI On Clinical Trials And Patient Outcomes
AI has the potential to revolutionize clinical trials in healthcare. According to recent data, AI can optimize study design, reduce patient burden, decrease amendments, increase success rates and improve trial efficiency. This technology can also attract and engage more committed patients to clinical trials.
In addition, AI can off-load tedious work for clinicians and provide more specific information about individual's treatment options. It enables medical professionals to identify healthcare needs and solutions at a faster pace and make informed decisions quickly.
Predictive analytics powered by AI can predict and reduce patient no-shows in healthcare systems. With improved diagnostic accuracy and patient outcome predictions, AI has tremendous potential to improve patient outcomes by 30-40% while reducing treatment costs up to 50%. Furthermore, pathology digitization for diagnostic healthcare is being improved with AI which ensures higher precision in detecting diseases while lowering medical errors.
AI also shows great promise in assessing acute ischemic stroke cases that enable health institutions to enhance the safety of patient care by delivering more customized treatment plans based on particular data points found on individual cases detected by machine learning algorithms within large data sets.
The impact of AI on clinical trials has yielded significant improvements across numerous aspects of the industry such as study design optimization, increased commitment from patients participating as well as identification of optimal areas where care delivery must be improved.
AI In Healthcare Management: The Potential Of AI In Healthcare Management And Administration
AI-enabled solutions are increasingly being adopted in healthcare management and administration due to their ability to provide immediate returns, including cost reduction, new product development, and better consumer engagement. One of the main use cases of AI in this field is prescription auditing, which can minimize errors that can lead to negative health outcomes for patients. Another use case is pregnancy management where AI can monitor mother and fetus for early diagnosis and reduced worries.
Real-time prioritization and triage is another area where prescriptive analytics on patient data can enable accurate case prioritization. This level of accuracy ensures that medical professionals focus on high-priority cases while still giving attention to lower priority ones. Visual and image-based data are driving AI adoption among healthcare providers particularly in medical imaging diagnostics. Predictive analytics through population health management supports financial success across the value-based care continuum.
AI also has an immense capacity for automation of complex healthcare processes with unstructured information, such as electronic health records (EHR) entries. Generative AI has endless potential as a co-pilot in addressing critical business challenges like clinical trials organization and improving the patient experience by providing support around-the-clock.
As technology continues to progress, understanding how we can enhance medical practices with technology remains crucial; there's been a recent upsurge in research into utilizing routine EHR data gathering as well further implementations within clinics - entire clinical systems may be integrated with storage facilities available through various vendors making it like a personal connected network for your healthcare team rather than just an EHR system! The potential benefits of using Artificial Intelligence alongside machine learning cannot be overstated when it comes down to promoting efficiency while maintaining quality control under stricter regulations continued by governmental agencies worldwide!
Ethical And Legal Implications: The Ethical And Legal Considerations Of AI In Medicine
As the use of AI in medicine continues to grow, so do the ethical and legal considerations surrounding it. One major concern is ensuring informed consent for the use of patient data. Patients must be fully aware of how their data will be used and have the option to opt out if they choose.
Algorithmic fairness and biases are also a concern as AI systems can reinforce systemic inequalities if not designed and implemented correctly. Data privacy is another issue that must be carefully considered, with strict measures in place to protect patient information.
The ethical implications extend beyond individual patients, with broader societal concerns about privacy, surveillance, bias and discrimination, and the role of human judgment in decision making processes. In breast cancer care specifically, there are additional ethical and legal considerations around how AI algorithms are trained on limited data sets that may not reflect diverse populations.
WHO has outlined six key principles for the ethical use of AI in health: transparency, accountability, inclusivity and non-discrimination, quality and safety, user-centered design and participatory approaches. However, even with these guiding principles in place there remain significant bioethical challenges related to implementation.
As we continue to push forward with developing new applications for AI in medicine it's essential that we carefully consider potential ethical concerns at every step along the way. Only by doing so can we ensure that both individual rights are protected while still advancing medical science as a whole.
Future Of AI In Medicine: Predictions For The Future Of AI In Medicine And Healthcare.
By 2030, it is predicted that AI will change healthcare towards truly proactive and predictive care. The current applications of AI in healthcare are numerous and growing. There are already top 18 use cases and vendors in the industry, structured around typical processes used in healthcare.
One significant breakthrough is the digitization of pathology using AI and ML which have improved diagnostic healthcare. Machine learning is one of the most common forms of AI used within healthcare to train algorithms with data sets. The PwC estimates that the market for AI healthcare applications will explode from $663.8 million in 2014 to $6.7 billion by 2021.
The potential impact of AI on medicine is broad reaching, potentially improving experiences for both medical professionals and patients by streamlining workflows as well as personalized treatments . Furthermore, there may be even more profound implications for patients with rare diseases or conditions that can only be diagnosed by medical geneticists who number only a couple thousand worldwide today In summary: advancements in applying artificial intelligence technology to diagnosis, drug discovery and virtually all aspects of clinical care delivery promise great things...
AI has the potential to revolutionize the field of medicine, and we have only just begun to scratch the surface of what it can do. From improving clinical trials to enhancing healthcare management and administration, AI can help us provide better care to patients and improve outcomes. However, we must also consider the ethical and legal implications of using AI in medicine and ensure that we are using it responsibly.
As we look to the future, the possibilities for AI in medicine are truly limitless. With continued research and development, we can expect to see even more exciting use cases emerge in the years to come. As healthcare providers and patients alike continue to embrace these new technologies, we can look forward to a brighter, healthier future for all.
Comments ()